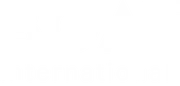
The global authority in superyachting
- NEWSLETTERS
- Yachts Home
- The Superyacht Directory
- Yacht Reports
- Brokerage News
- The largest yachts in the world
- The Register
- Yacht Advice
- Yacht Design
- 12m to 24m yachts
- Monaco Yacht Show
- Builder Directory
- Designer Directory
- Interior Design Directory
- Naval Architect Directory
- Yachts for sale home
- Motor yachts
- Sailing yachts
- Explorer yachts
- Classic yachts
- Sale Broker Directory
- Charter Home
- Yachts for Charter
- Charter Destinations
- Charter Broker Directory
- Destinations Home
- Mediterranean
- South Pacific
- Rest of the World
- Boat Life Home
- Owners' Experiences
- Interiors Suppliers
- Owners' Club
- Captains' Club
- BOAT Showcase
- Boat Presents
- Events Home
- World Superyacht Awards
- Superyacht Design Festival
- Design and Innovation Awards
- Young Designer of the Year Award
- Artistry and Craft Awards
- Explorer Yachts Summit
- Ocean Talks
- The Ocean Awards
- BOAT Connect
- Between the bays
- Golf Invitational
- Boat Pro Home
- Pricing Plan
- Superyacht Insight
- Product Features
- Premium Content
- Testimonials
- Global Order Book
- Tenders & Equipment
BANDIDO is a 27.9 m Motor Yacht, built in Taiwan by Jade Yachts and delivered in 2008. She is one of 8 Bandido 90 Explorer models.
Her top speed is 12.9 kn and she boasts a maximum range of 3500.0 nm when navigating at cruising speed, with power coming from two Caterpillar diesel engines. She can accommodate up to 8 guests in 4 staterooms, with 4 crew members waiting on their every need. She has a gross tonnage of 225.0 GT and a 7.5 m beam.
She was designed by Espinosa Yacht Design , who also designed the interior. Espinosa Yacht Design has designed 38 yachts and designed the interior of 25 yachts for yachts above 24 metres.
The naval architecture was developed by Vripack (61 other superyachts architected) and Jade Yachts (3 other superyachts architected) - she is built with a Teak deck, a Steel hull, and Aluminium superstructure.
BANDIDO is one of 5739 motor yachts in the 24-30m size range, and, compared to similarly sized motor yachts, her volume is 112.98 GT above the average.
BANDIDO is currently sailing under the Portugal flag (along with a total of other 56 yachts). She is known to be an active superyacht and has most recently been spotted cruising near Spain. For more information regarding BANDIDO's movements, find out more about BOAT Pro AIS .

Specifications
- Name: BANDIDO
- Yacht Type: Motor Yacht
- Yacht Subtype: Displacement , Expedition Yacht
- Model: Bandido 90 Explorer
- Builder: Jade Yachts
- Naval Architect: Vripack , Jade Yachts
- Exterior Designer: Espinosa Yacht Design
- Interior Designer: Espinosa Yacht Design
Yachts like this
From our partners, sponsored listings.

- Style & Beauty
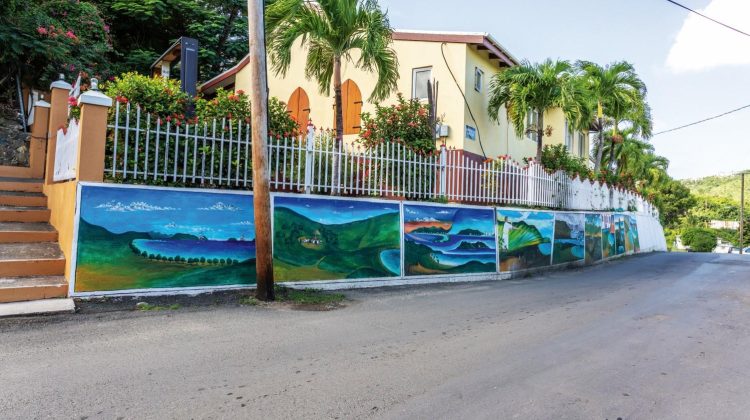
Latest Posts
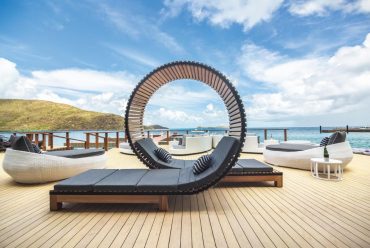
Featured Property
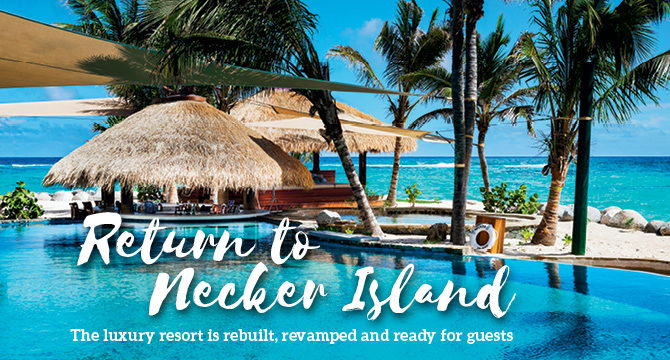
Return to Necker Island
September 2016 brought devastation to the British Virgin Islands like many had never seen. Hurricanes Irma and Maria left a path of destruction and no island was spared. Tens of thousands of people lost everything, and it’s isolated location...
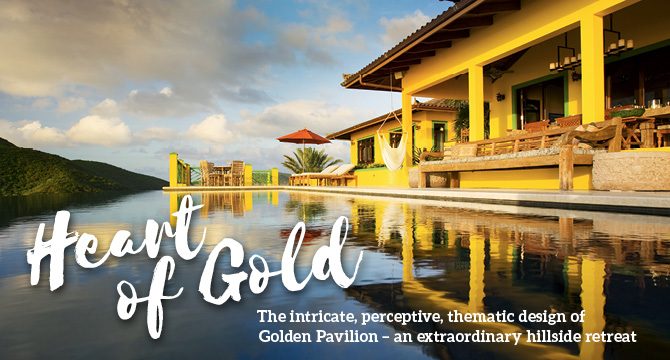
Heart of Gold
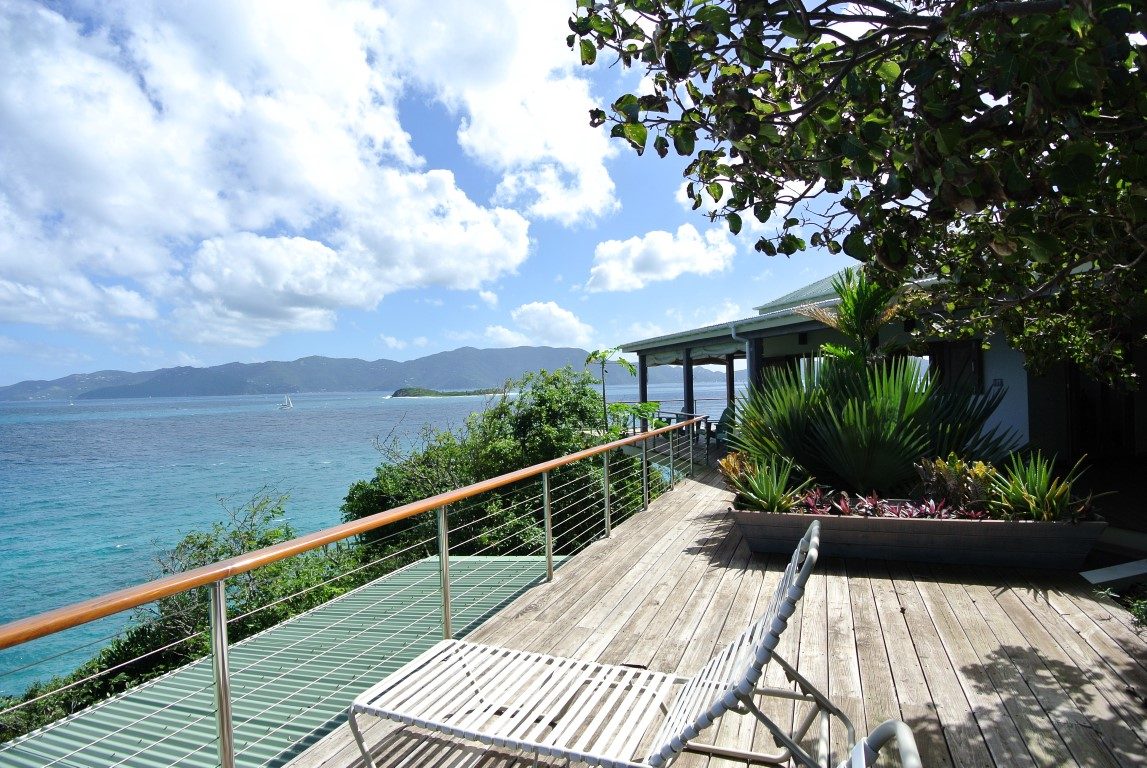
Relishing Retreats
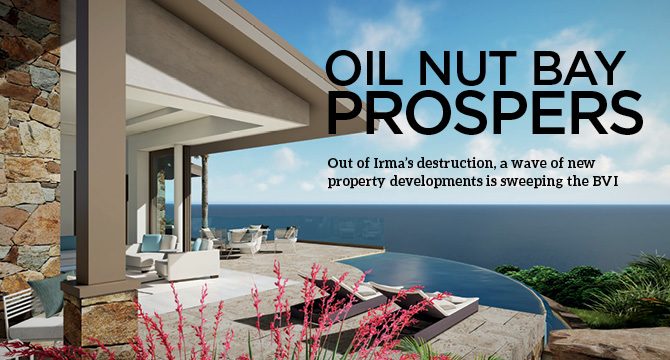
Oil Nut Bay Prospers
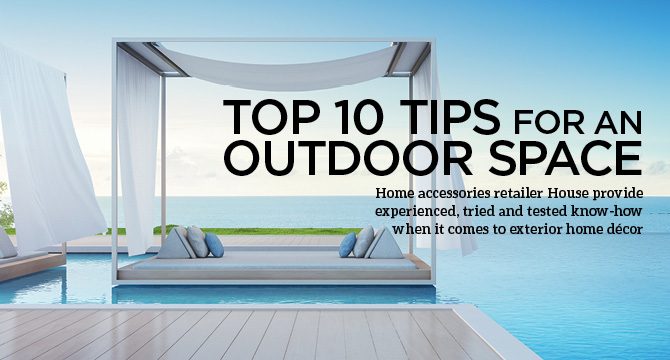
Top 10 Tips for an Outdoor Space
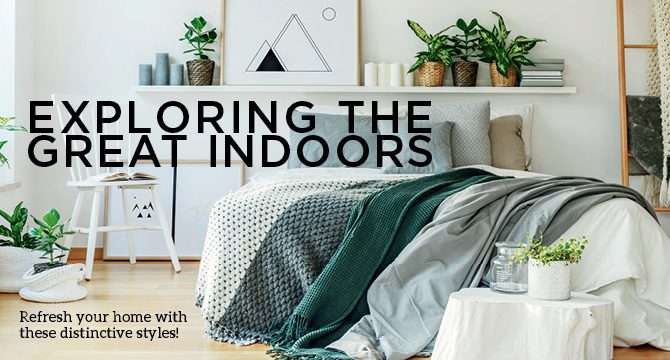
Exploring the Great Indoors
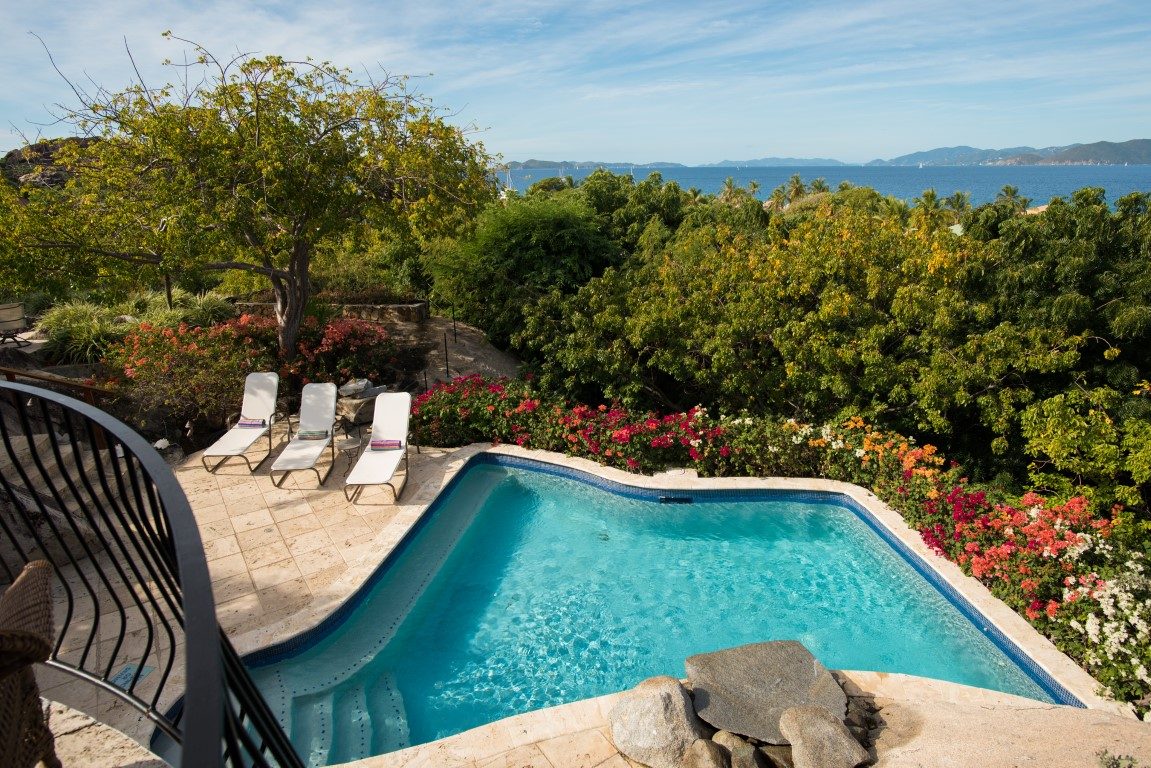
Scenic Serenity
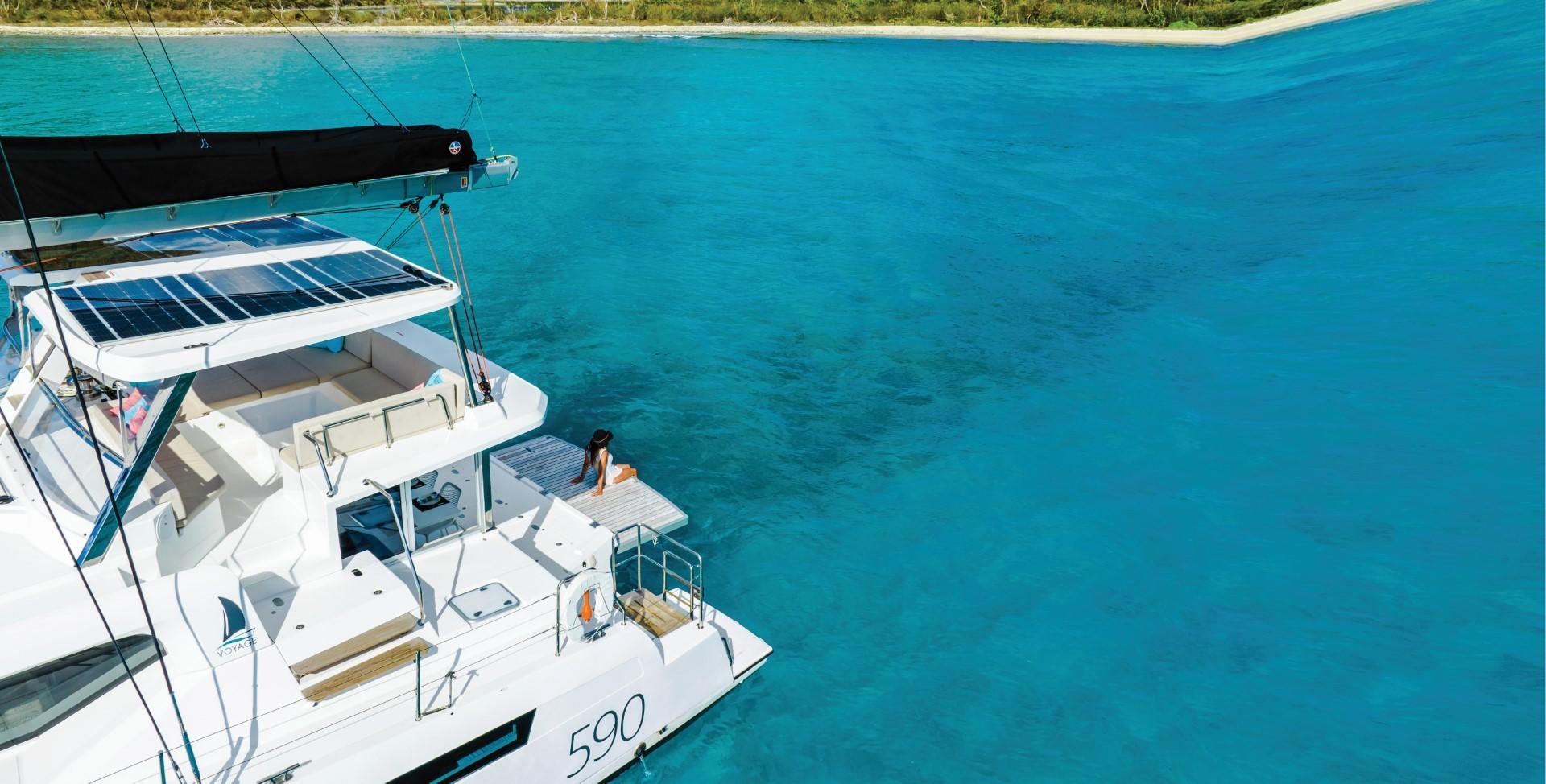
Your Private Villa on the Water
For almost 30 years VOYAGE Yachts have built beautiful, international award-winning yachts well known for their strength and quality craftsmanship. VOYAGE Charters is based in Sopers Hole Marina and incorporates the West End Boat Yard, owned and operated by...
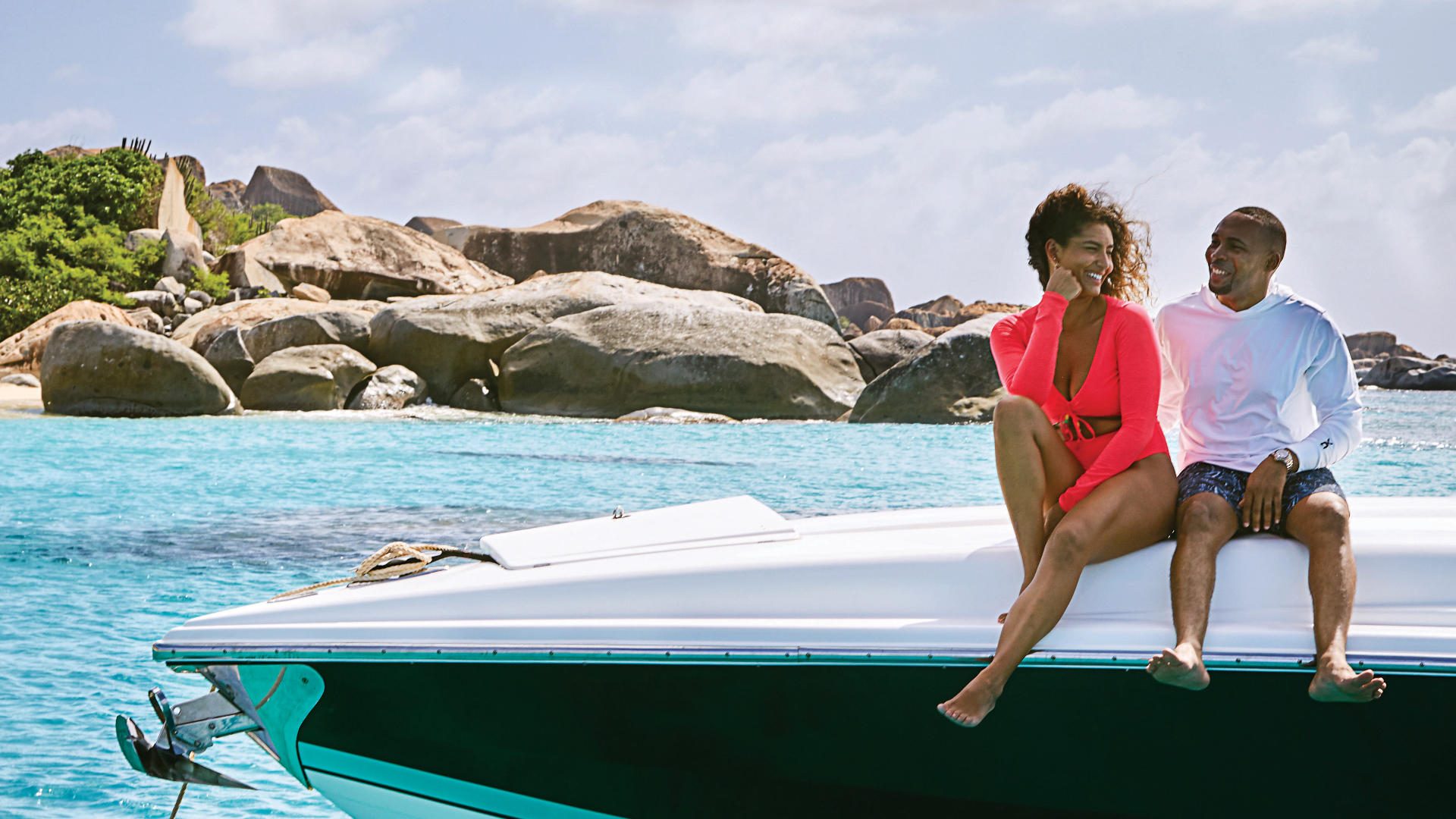
A Maritime Engineering Masterpiece
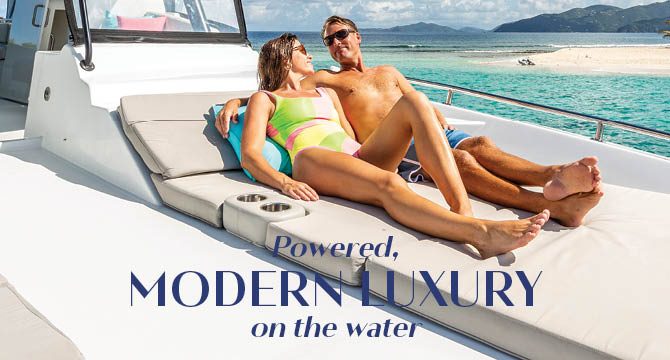
Powered – Modern Luxury on the water
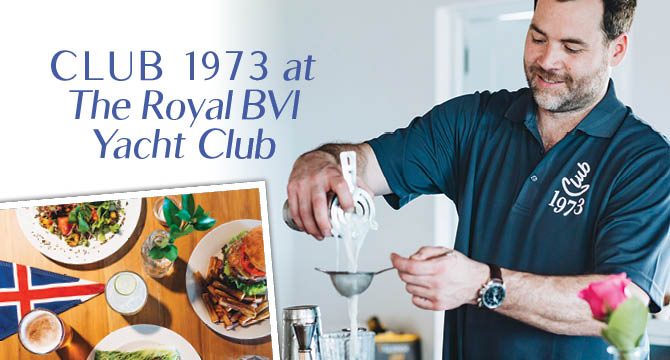
Club 1973 at The Royal BVI Yacht Club
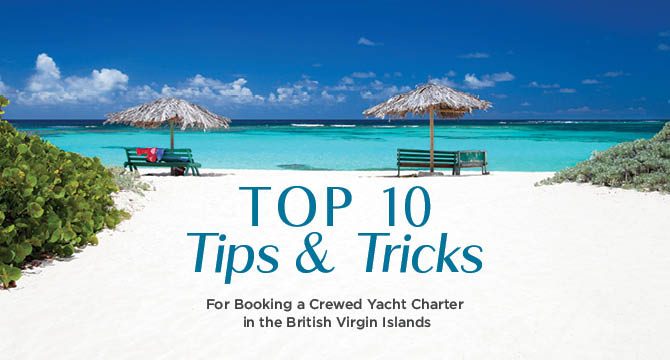
Top 10 Tips & Tricks
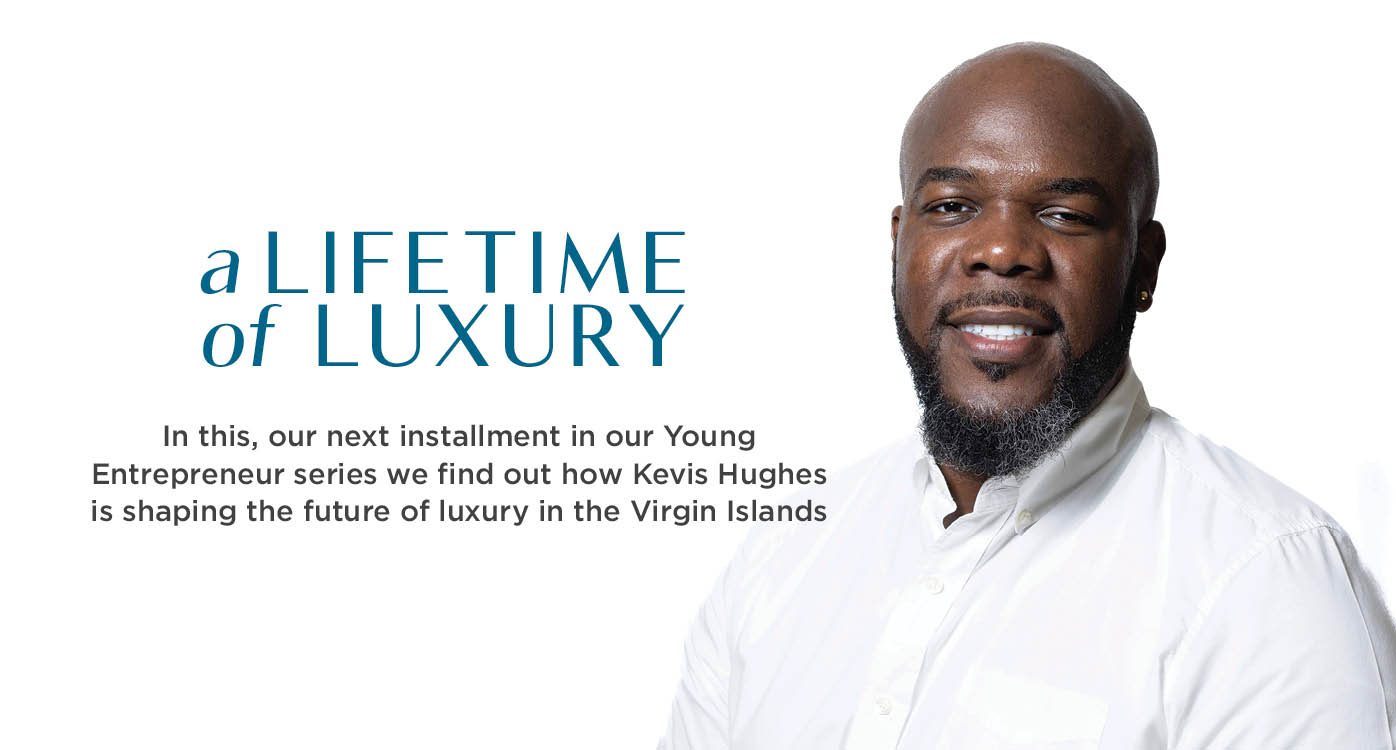
a lifetime of Luxury
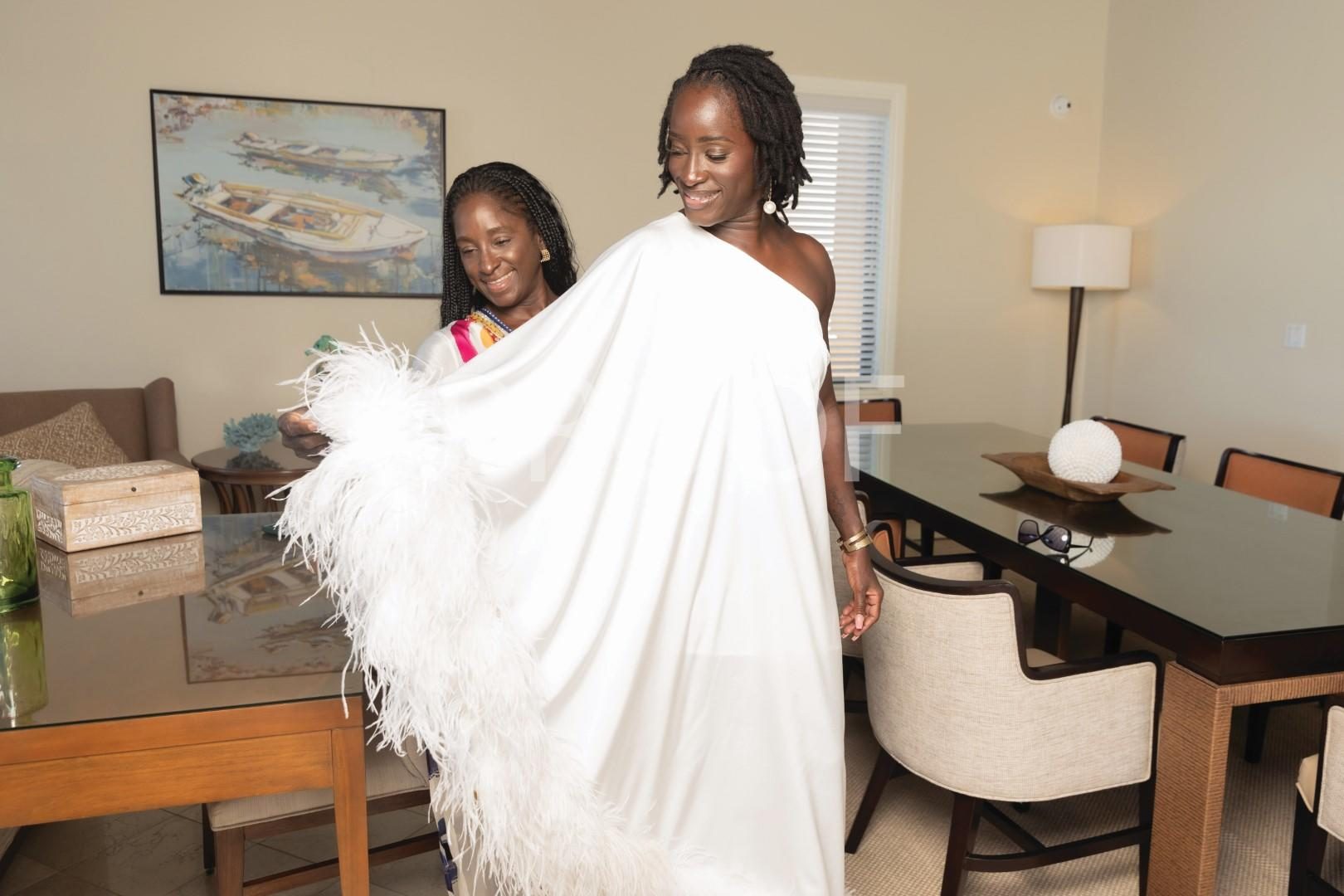
As I step inside UMI on Road Town’s Waterfront Drive, I’m drawn to rails of beautiful garments – a sheer kaftan in the deepest of green, a soft white off-the shoulder gown trimmed with feathers and an aqua blue...
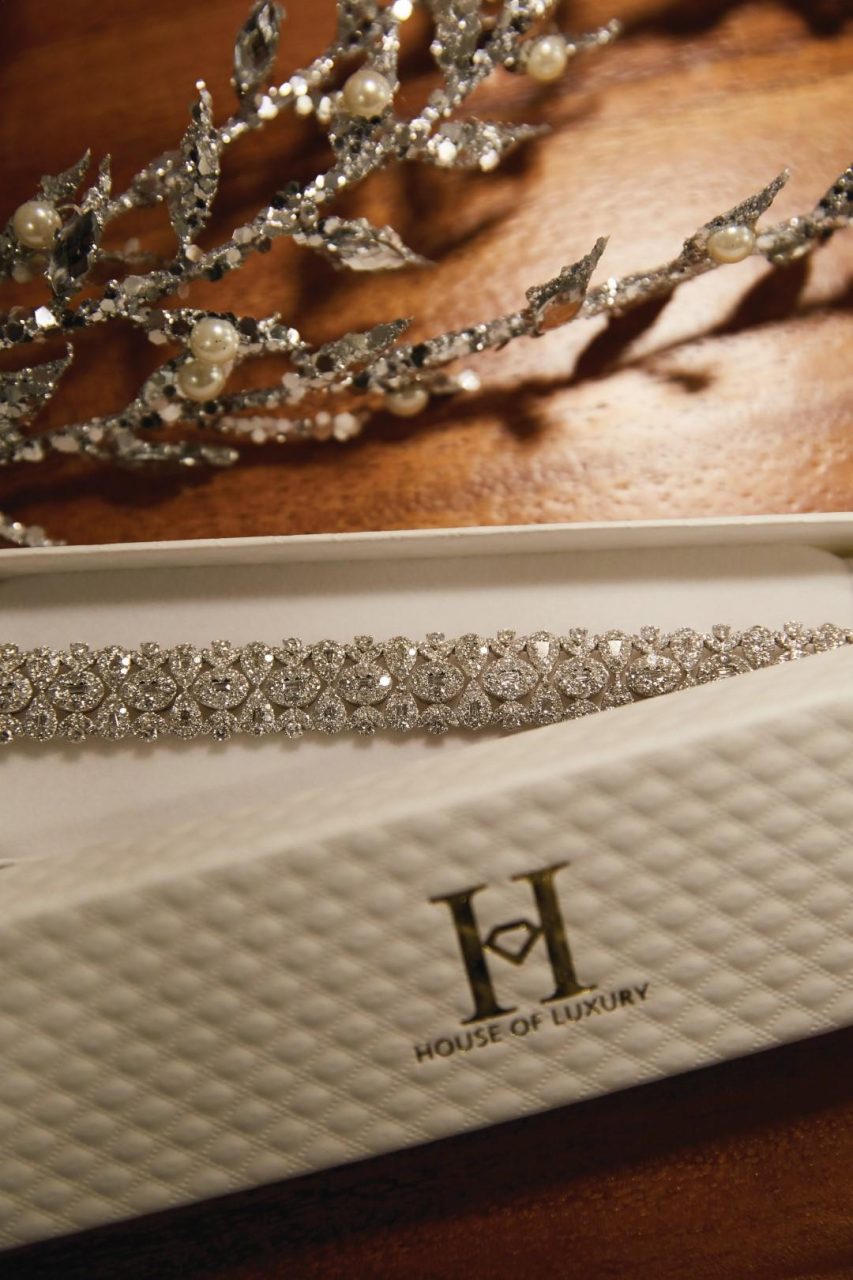
LUXURY BY DESIGN
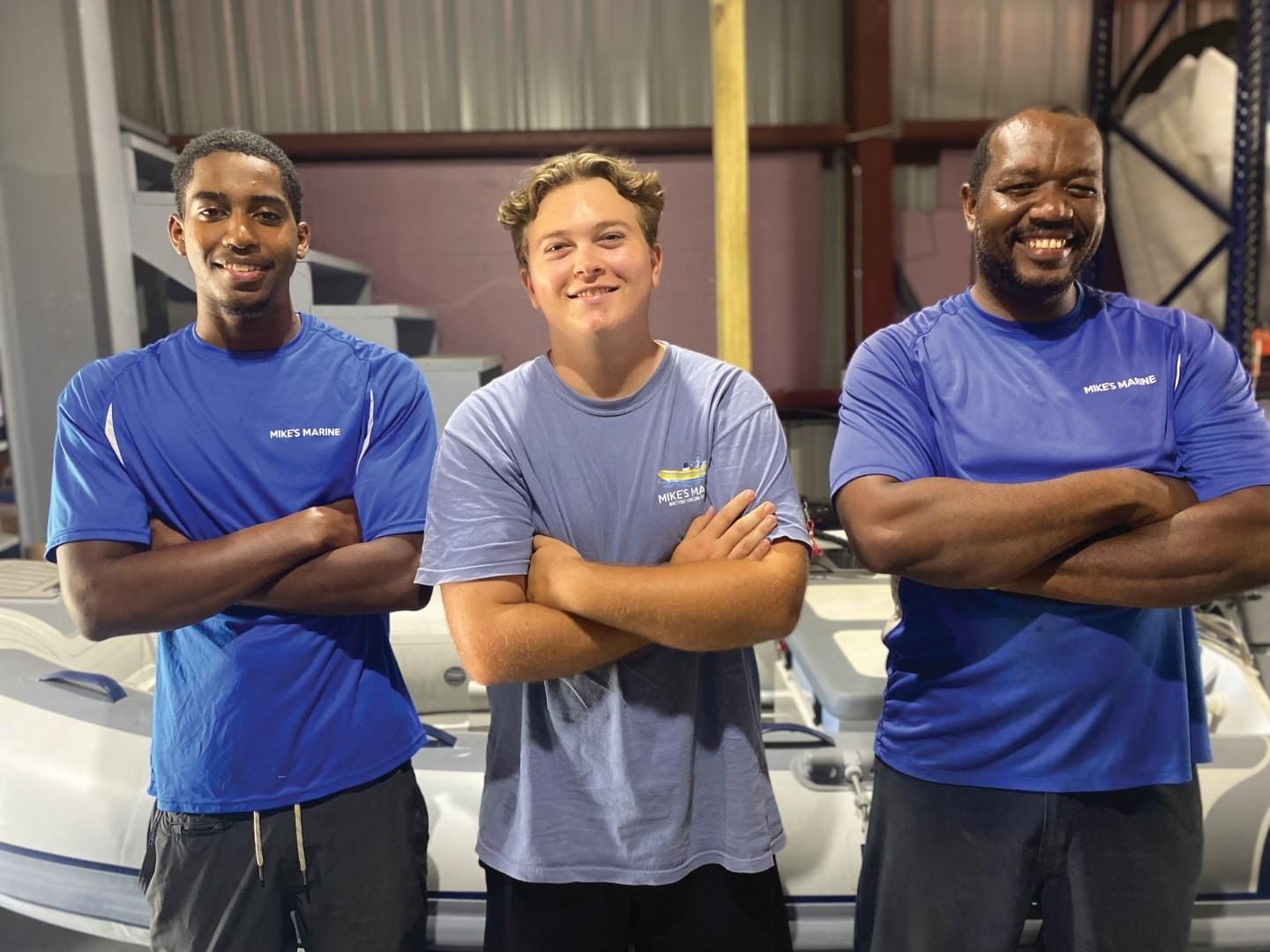
Meet Captain Mike
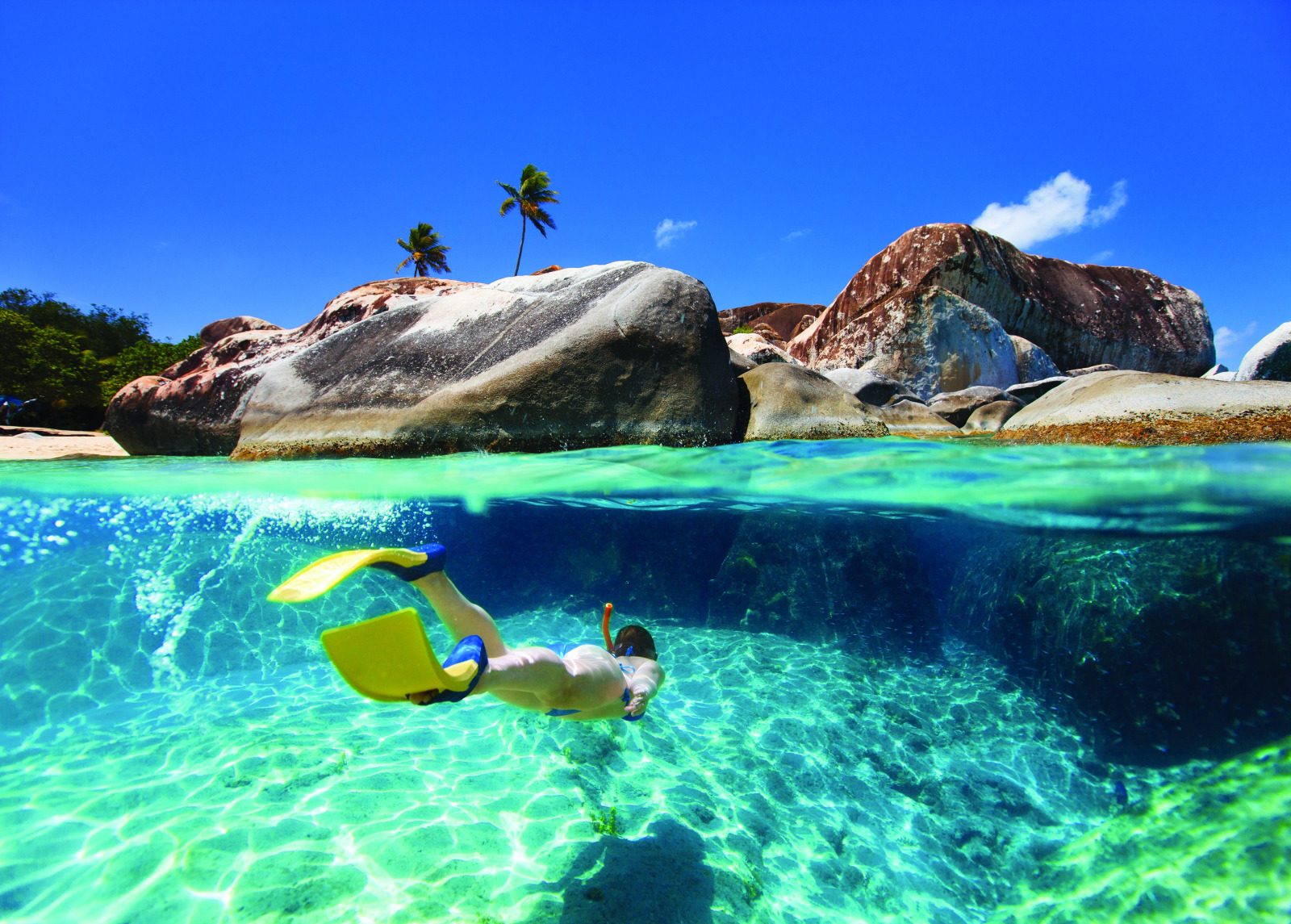
A Geological Wonder the National Park
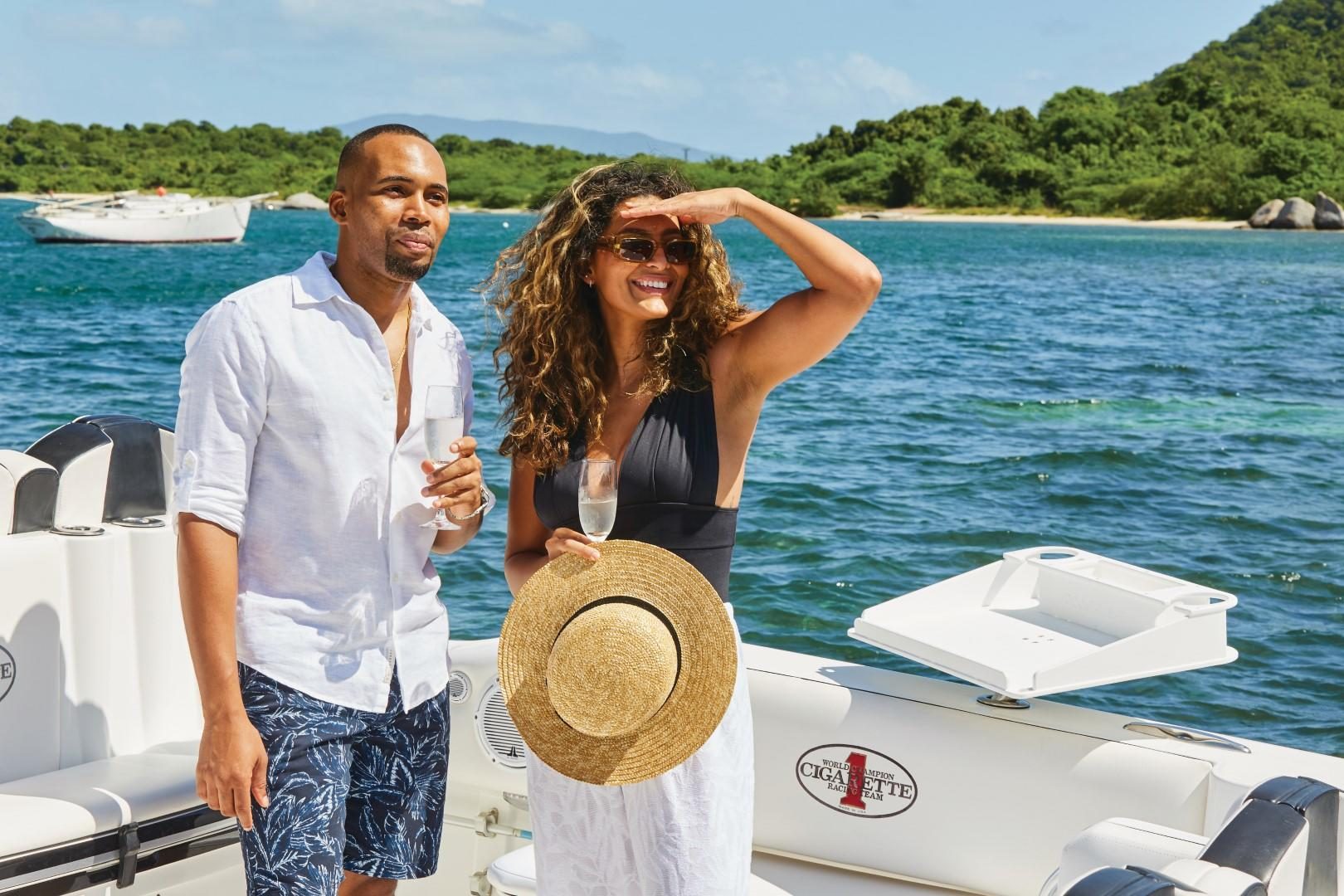
BVI Specialists on Land and Sea
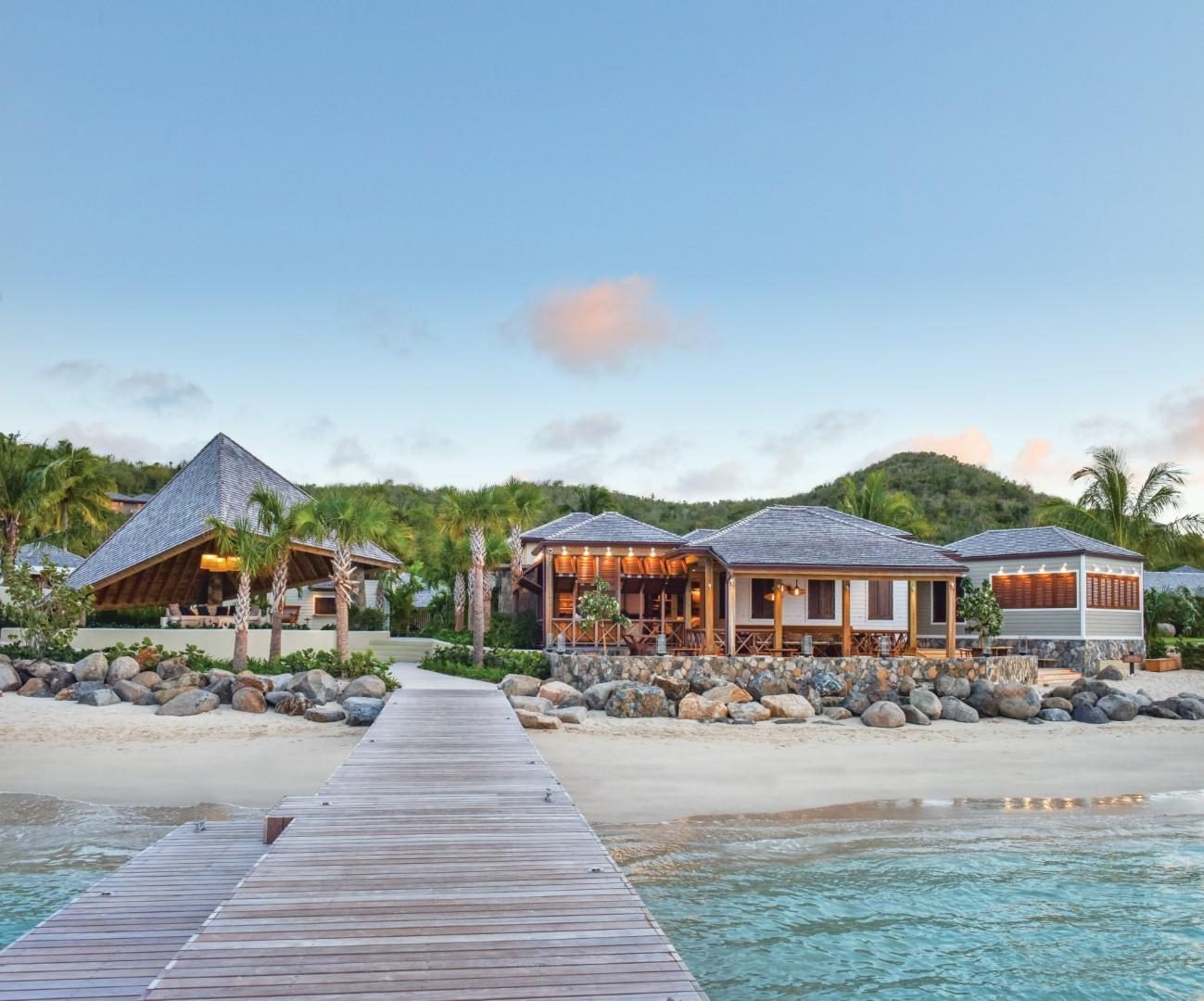
Architect of Storied Places
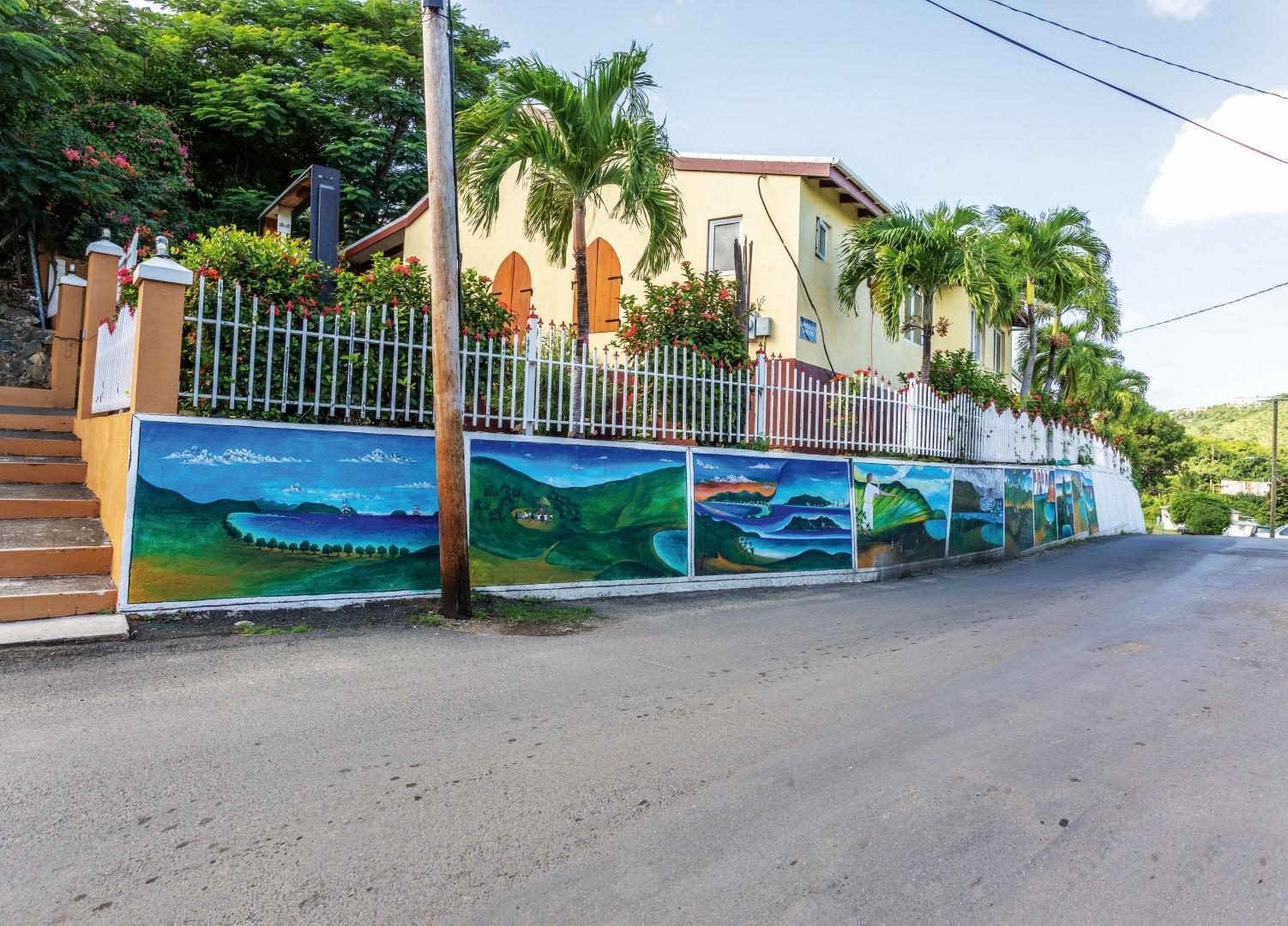
Meet the Maker
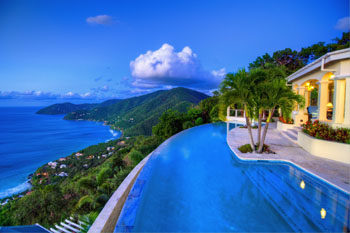
Celestial House
Awe-inspiring surroundings, Tortola
VILLAS FOR SALE:
CLICK FOR PRICE
Parcel 29 Virgin Gorda
FREEHOLD land in the British Virgin Islands
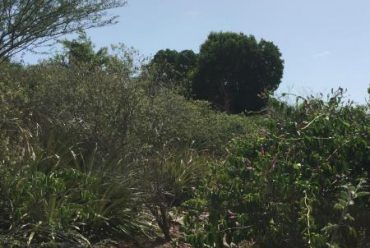
Parcel 11 Virgin Gorda
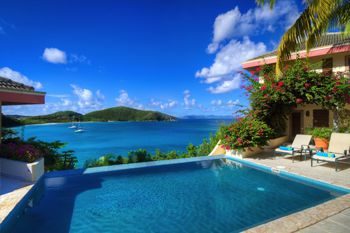
A Dream Come True
Oceanfront Estate, Virgin Gorda
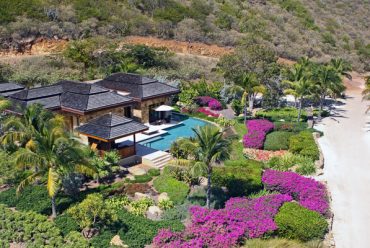
Jewel Box Villa
Neighbourhood at the heart of Oil Nut Bay
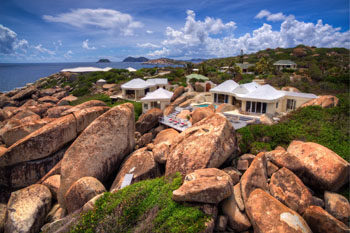
Water’s Edge
Caribbean Fantasy Home, Virgin Gorda
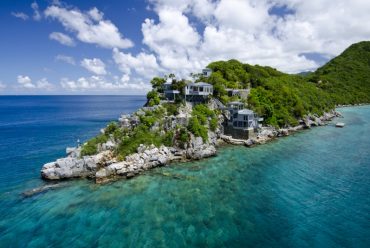
Steele Point Estate
This stunning Estate consisting of four homes on
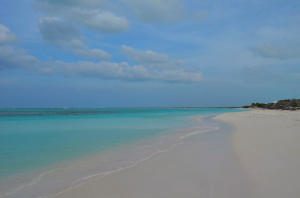
17 & 19 Anegada
A 9-acre piece of virgin beachfront Caribbean is
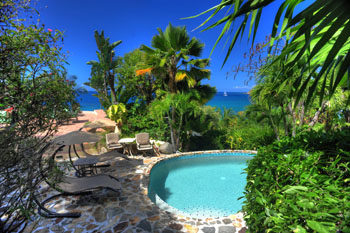
Sunset Watch & Sundowner
On Paradise Lane, Virgin Gorda
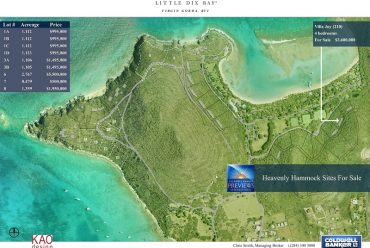
Lot 6 at Little Dix Bay
Prime waterfront on Virgin Gorda.
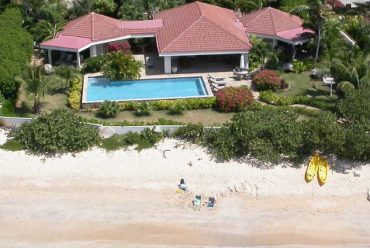
Exquisite beachfront villa
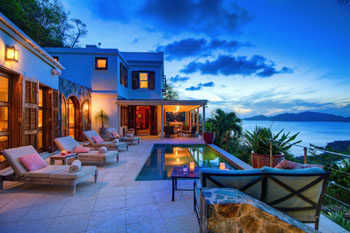
Cohoba House
Modern and Turn-Key, Tortola
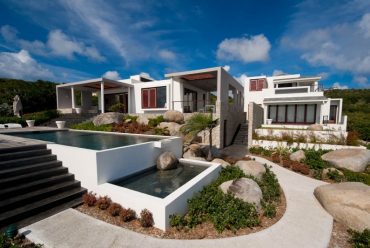
A contemporary BVI villa
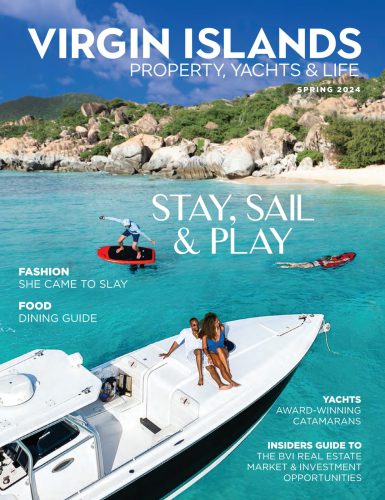
Lolalita’s Top Fish Recipe
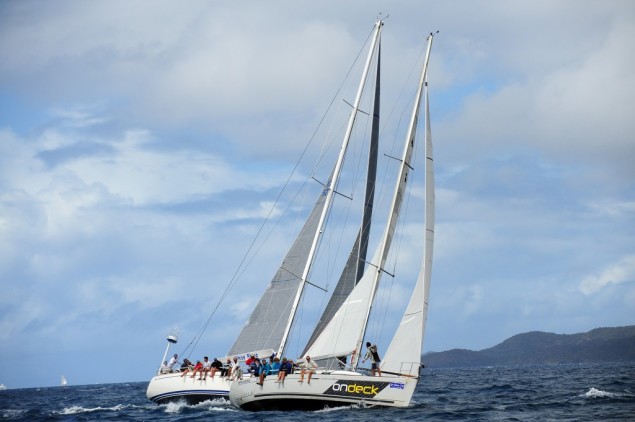
44 Years and Still Sailing – Spring Regatta
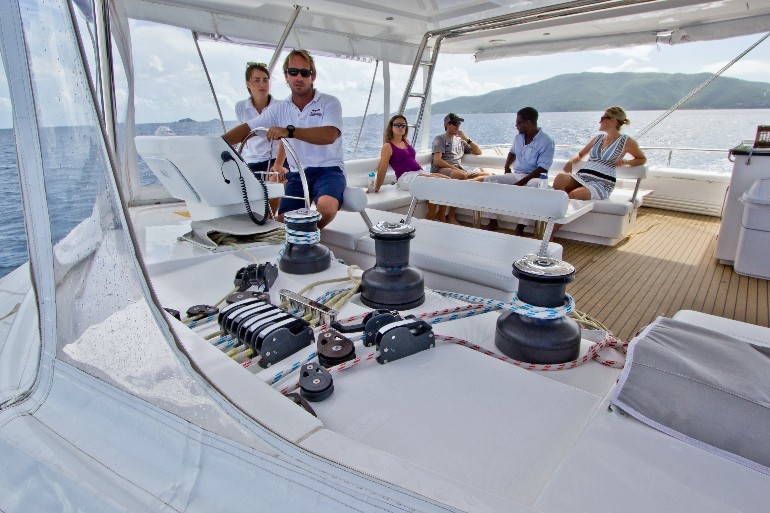
Get The Best Of The BVI Today!
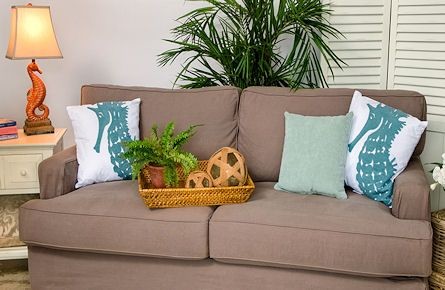
Great Christmas Gift Ideas from the British Virgin Islands [Retail]
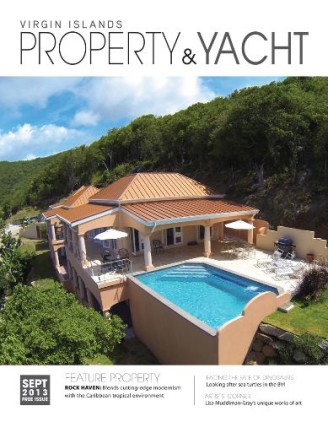
Editor’s Letter: September 2013 – BVI Property and Yacht Magazine
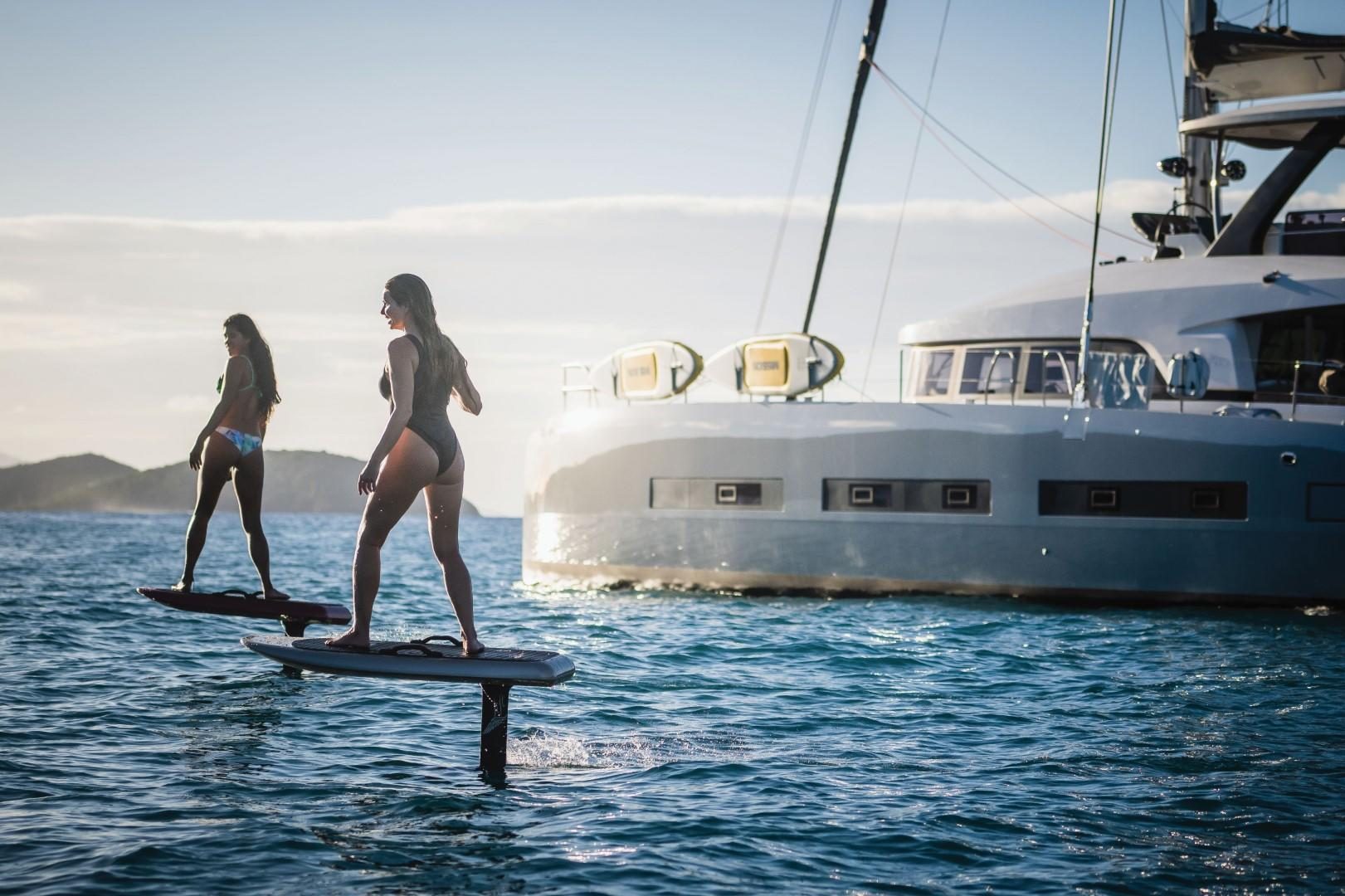
Foil & Fly
Please use a modern browser to view this website. Some elements might not work as expected when using Internet Explorer.
- Landing Page
- Luxury Yacht Vacation Types
- Corporate Yacht Charter
- Tailor Made Vacations
- Luxury Exploration Vacations
- View All 3600
- Motor Yachts
- Sailing Yachts
- Classic Yachts
- Catamaran Yachts
- Filter By Destination
- More Filters
- Latest Reviews
- Charter Special Offers
- Destination Guides
- Inspiration & Features
- Mediterranean Charter Yachts
- France Charter Yachts
- Italy Charter Yachts
- Croatia Charter Yachts
- Greece Charter Yachts
- Turkey Charter Yachts
- Bahamas Charter Yachts
- Caribbean Charter Yachts
- Australia Charter Yachts
- Thailand Charter Yachts
- Dubai Charter Yachts
- Destination News
- New To Fleet
- Charter Fleet Updates
- Special Offers
- Industry News
- Yacht Shows
- Corporate Charter
- Finding a Yacht Broker
- Charter Preferences
- Questions & Answers
- Add my yacht
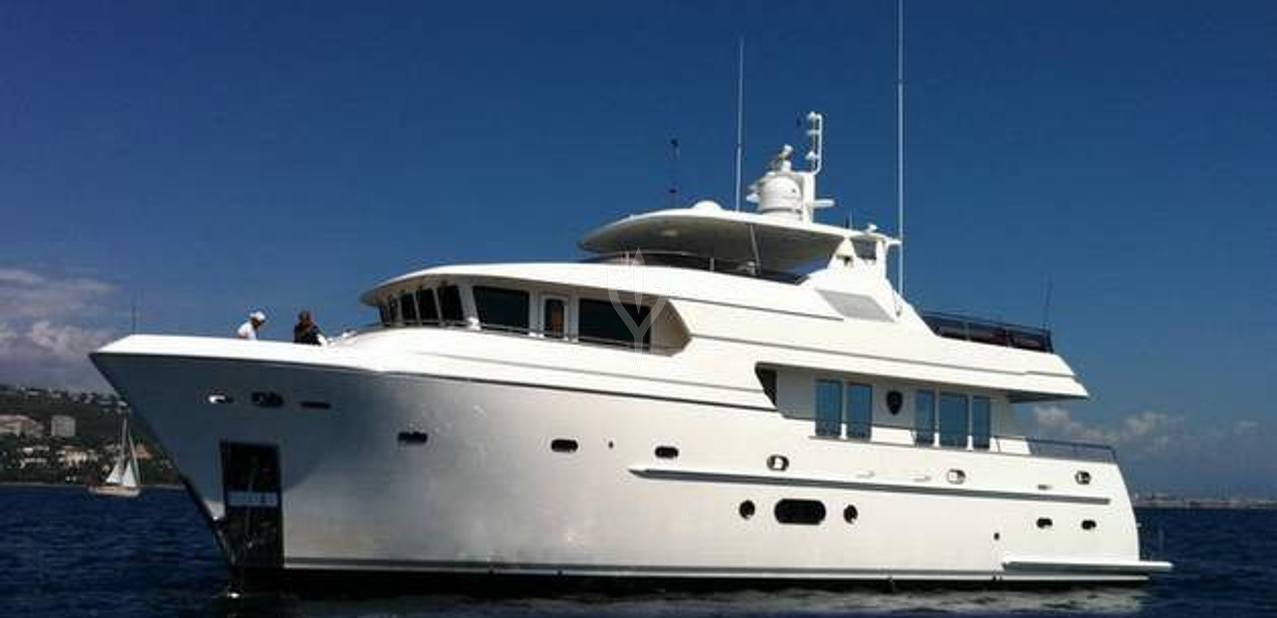
NOT FOR CHARTER *
This Yacht is not for Charter*
SIMILAR YACHTS FOR CHARTER
View Similar Yachts
Or View All luxury yachts for charter
- Luxury Charter Yachts
- Motor Yachts for Charter
- Amenities & Toys
BANDIDO 75 yacht NOT for charter*
23.67m / 77'8 | horizon | drettmann yachts | 2008.
Owner & Guests
Cabin Configuration
- Previous Yacht
The 23.67m/77'8" motor yacht 'Bandido 75' was built by Horizon in Taiwan. This luxury vessel's exterior design is the work of Horizon.
Guest Accommodation
Bandido 75 has been designed to comfortably accommodate up to 8 guests in 4 suites. She is also capable of carrying up to 2 crew onboard to ensure a relaxed luxury yacht experience.
Range & Performance
Bandido 75 is built with a GRP hull and GRP superstructure, with teak decks. Her water tanks store around 3,000 Litres of fresh water.
*Charter Bandido 75 Motor Yacht
Motor yacht Bandido 75 is currently not believed to be available for private Charter. To view similar yachts for charter , or contact your Yacht Charter Broker for information about renting a luxury charter yacht.
Bandido 75 Yacht Owner, Captain or marketing company
'Yacht Charter Fleet' is a free information service, if your yacht is available for charter please contact us with details and photos and we will update our records.
Bandido 75 Photos
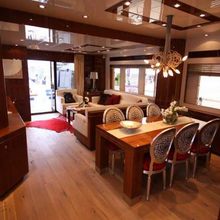
NOTE to U.S. Customs & Border Protection
Specification
M/Y Bandido 75
SIMILAR LUXURY YACHTS FOR CHARTER
Here are a selection of superyachts which are similar to Bandido 75 yacht which are believed to be available for charter. To view all similar luxury charter yachts click on the button below.
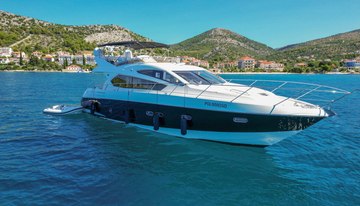
21m | Sunseeker
from $26,000 p/week ♦︎
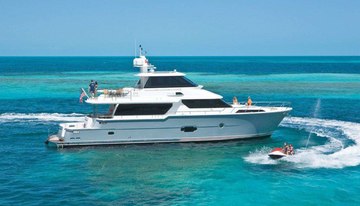
23m | Horizon
from $29,000 p/week
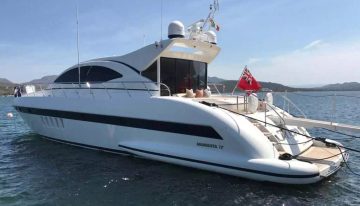
22m | Overmarine
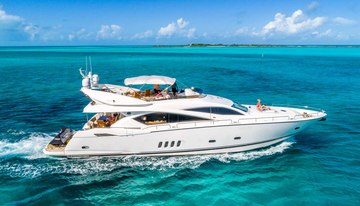
Acqua Alberti
25m | Sunseeker
from $44,500 p/week
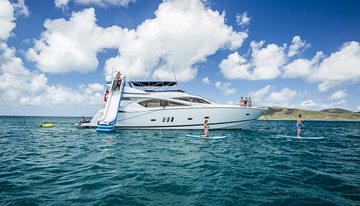
24m | Sunseeker
from $41,000 p/week ♦︎
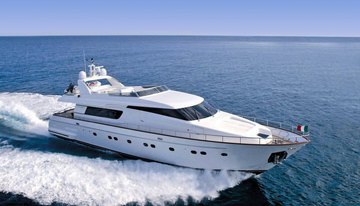
24m | Sanlorenzo
from $34,000 p/week ♦︎
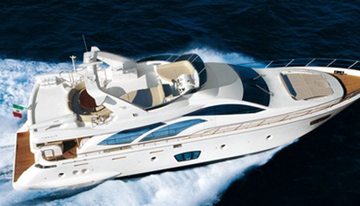
23m | Azimut
from $30,000 p/week
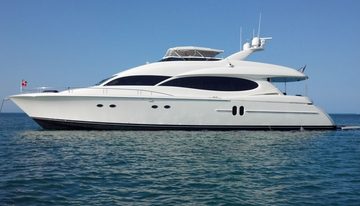
Always Barefoot
24m | Lazzara
from $28,500 p/week
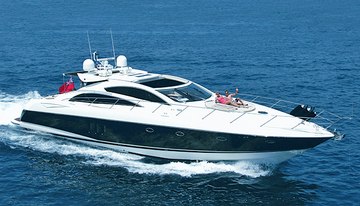
22m | Sunseeker
POA ♦︎
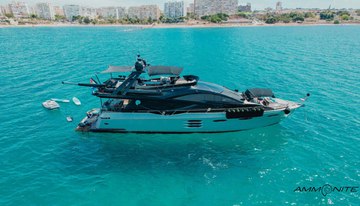
24m | Numarine
from $52,000 p/week ♦︎
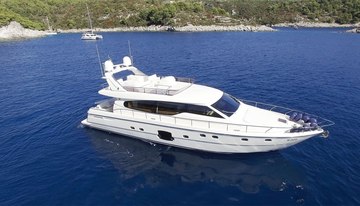
20m | Ferretti Yachts
from $18,000 p/week ♦︎
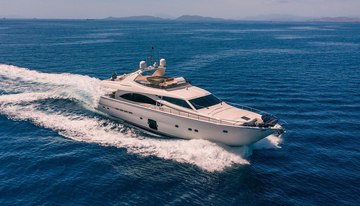
25m | Ferretti Yachts
from $42,000 p/week ♦︎
As Featured In
The YachtCharterFleet Difference
YachtCharterFleet makes it easy to find the yacht charter vacation that is right for you. We combine thousands of yacht listings with local destination information, sample itineraries and experiences to deliver the world's most comprehensive yacht charter website.
San Francisco
- Like us on Facebook
- Follow us on Twitter
- Follow us on Instagram
- Find us on LinkedIn
- Add My Yacht
- Affiliates & Partners
Popular Destinations & Events
- St Tropez Yacht Charter
- Monaco Yacht Charter
- St Barts Yacht Charter
- Greece Yacht Charter
- Mykonos Yacht Charter
- Caribbean Yacht Charter
Featured Charter Yachts
- Maltese Falcon Yacht Charter
- Wheels Yacht Charter
- Victorious Yacht Charter
- Andrea Yacht Charter
- Titania Yacht Charter
- Ahpo Yacht Charter
Receive our latest offers, trends and stories direct to your inbox.
Please enter a valid e-mail.
Thanks for subscribing.
Search for Yachts, Destinations, Events, News... everything related to Luxury Yachts for Charter.
Yachts in your shortlist

Find anything, super fast.
- Destinations
- Documentaries
We don't have any additional photos of this yacht. Do you?
Motor Yacht
El Bandido is a custom motor yacht launched in 2008 by Custom, in Turkey.
El Bandido measures 31.70 metres in length and has a beam of 6.50 feet.
El Bandido has a steel hull.
Performance and Capabilities
El Bandido has a top speed of 14.00 knots and a cruising speed of 12.00 knots. .
Accommodation
El Bandido accommodates up to 8 guests in 4 cabins. She also houses room for up to 4 crew members.
Other Specifications
El Bandido flies the flag of Turkish.
- Yacht Builder Custom No profile available
Yacht Specs
Other custom yachts.
BANDIDO ICE YACHTS
- Inspiration
BANDIDO has 1 Photos
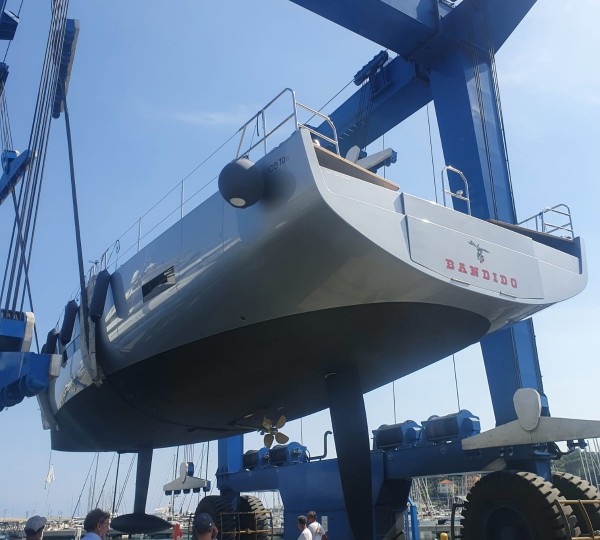
Bandido News
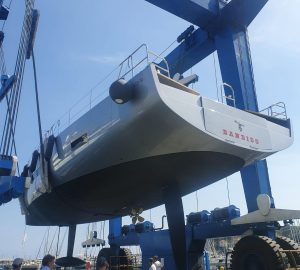
Ice Yachts launches ICE 70 sailing ...
Similar yachts.
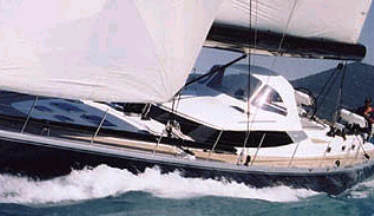
Storm 2 Eagle 66 | From AUS$ 35,000/wk
- Yachts >
- All Yachts >
- All Sail Boats 60ft/18m - 100ft/30m >
If you have any questions about the BANDIDO information page below please contact us .
Luxury sailing yacht BANDIDO from Italian shipyard Ice Yachts is a 21.3m/70ft ICE 70 model with exterior styling from Felci Yacht Design. The hull and superstructure are constructed from carbon with a fibreglass sandwich to create a lightweight draft and excellent efficiency while cruising. Depending on the ICE 70 layout the Owner selected, S/Y BANDIDO sleeps 6-8 guests in 3-4 cabins, plus a crew of 2.
NOTABLE FEATURES OF BANDIDO: ~Ideal family-sized vessel ~Lifting keel for draft of 2.8m/9.2ft-4.5m/15ft ~Top speed of 18 knots ~Air conditioning ~Wi-Fi
On deck, the forward section is left clear for crew operations, and when at anchor the space can be used for sunbathing, lounging or observation. Amidships is the cockpit, which is shaded by a coachroof for use throughout the day. L-shaped seating is placed on both sides, with a table centre for casual meals and drinks. The helm controls are behind to port and starboard on both sides.
BANDIDO Specifications
Below deck, the interior offers a warm and contemporary setting that uses traditional elements to connect to maritime heritage. The crew accommodation is placed in the bow, followed by the guest accommodation, galley and the salon and formal dining area amidships. A sofa and widescreen TV are placed on the port side, and an L-shaped sofa and table to starboard. A stairway connects up to the cockpit, and farther aft are the remaining guest cabins and the tender garage in the stern.
The Yanmar 4LV engine of 195 HP ensures that guests can continue cruising even in windless conditions.
Yacht Accommodation
There are two different accommodation layouts available in the ICE 70 model: The first sleeping 6 guests in 3 guest cabins, the second sleeping 8 guests in 4 guest cabins. The three-cabin layout consists of a Master suite, double cabin, and a twin or bunk cabin. The four-cabin layout consists of a Master suite, double cabin, twin cabin and bunk cabin. A crew cabin for 2 is placed in the bow in both versions.
Amenities and Extras
We do have available further amenity, owner and price information for the 21.3m (70') yacht BANDIDO, so please enquire for more information.
BANDIDO Disclaimer:
The luxury yacht BANDIDO displayed on this page is merely informational and she is not necessarily available for yacht charter or for sale, nor is she represented or marketed in anyway by CharterWorld. This web page and the superyacht information contained herein is not contractual. All yacht specifications and informations are displayed in good faith but CharterWorld does not warrant or assume any legal liability or responsibility for the current accuracy, completeness, validity, or usefulness of any superyacht information and/or images displayed. All boat information is subject to change without prior notice and may not be current.
Quick Enquiry
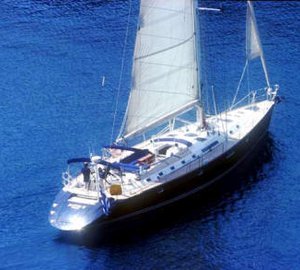
Marina II crewed | From EUR€ 14,700/wk
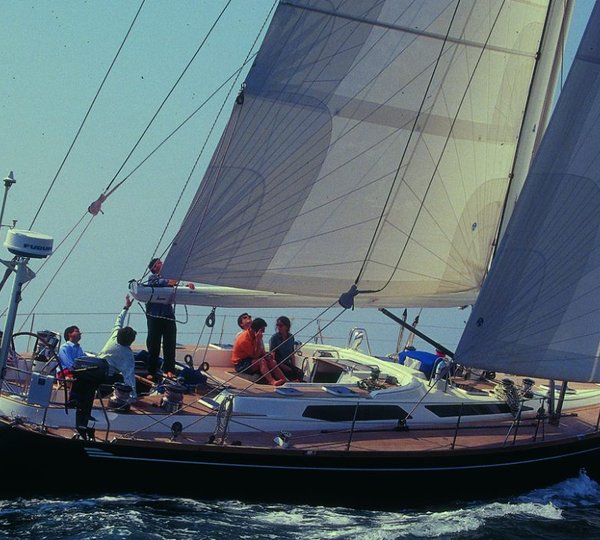
Triumph | From US$ 15,000/wk
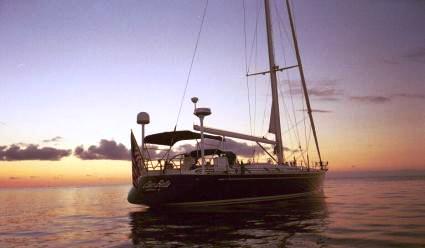
Ciao Bella | From US$ 15,000/wk
education consultant jobs remote part time
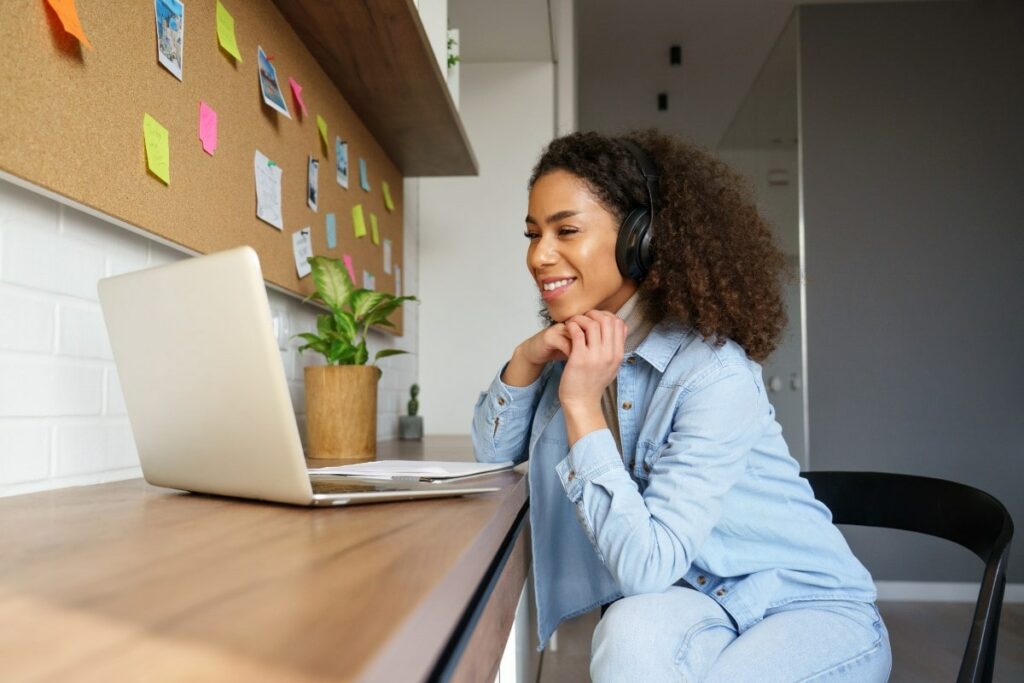
- Majesty Yachts
- Bandido Yachts
- Moonen Yachts
- Nomad Yachts
- Buy a yacht
- Sell a yacht
- Charter a Yacht
- News & Events
Bandido 115
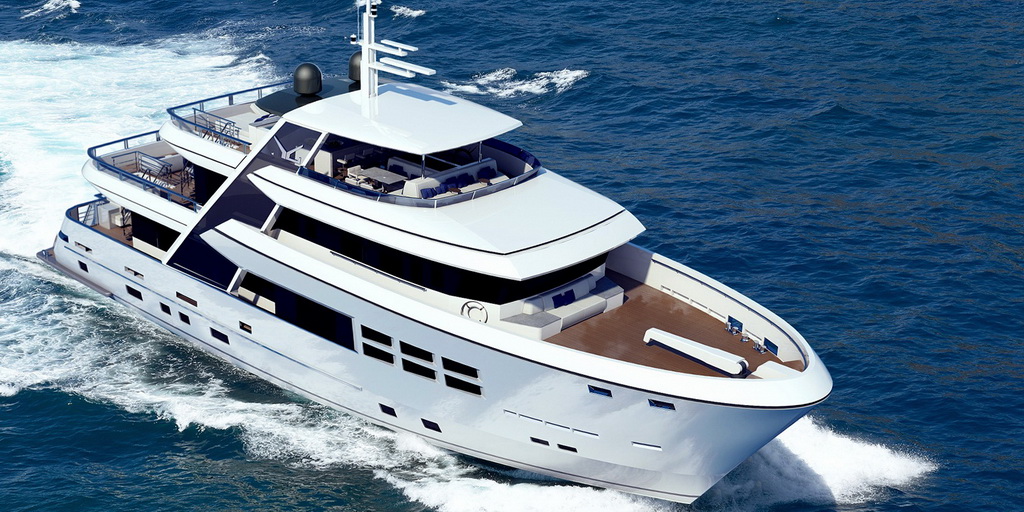
Specifications
Price on request.
Location Germany
Project No. DY20052
Key features
- Interior - Claudia Drettmann
- Exterior - Drettmann Yachts
- Space for Dinghy and surfboards
- Large beach club
About Bandido 115
Embark on a journey of luxury and unparalleled maritime exploration with Drettmann. Renowned for its enduring legacy on the world's oceans, our brand stands as a beacon of "Made in Germany" excellence, showcasing impeccable design and enduring value. Our products and service have not just claimed pivotal positions in the yachting market but have also earned the admiration of countless owners. To maintain this legacy, our latest models have been meticulously redesigned from the very beginning, unveiling a new level of comfort, spaciousness, and cutting-edge engine innovations. These yachts transcend the norms of their class, setting a new standard in design, technology and performance. At Drettmann, we recognize that individuals hold unique visions or aspirations. Our team specializes in crafting bespoke dream yachts in collaboration with our handpicked, highly skilled partners. The Bandido yacht, true to its name, embodies the spirit of exploration and active sea-bound adventures. Whether indulging in water sports, diving escapades, cruising the Caribbean, or navigating the far reaches of the North, it stands as the ideal companion. Fusing activities with luxury, this robust yacht boasts generous onboard space for tenders, surfboards, diving gear, and water toys, without compromising on comfort. The latest models ranging from 80 to 148 feet represent an exponential leap forward in refinement compared to their esteemed predecessors. Features, such as the revamped interior design, the innovative POD-drive, or the significantly expanded beach club, redefine luxury yachting, offering an experience previously reserved for much larger vessels. Discover a new era of opulence and adventure with Drettmann.
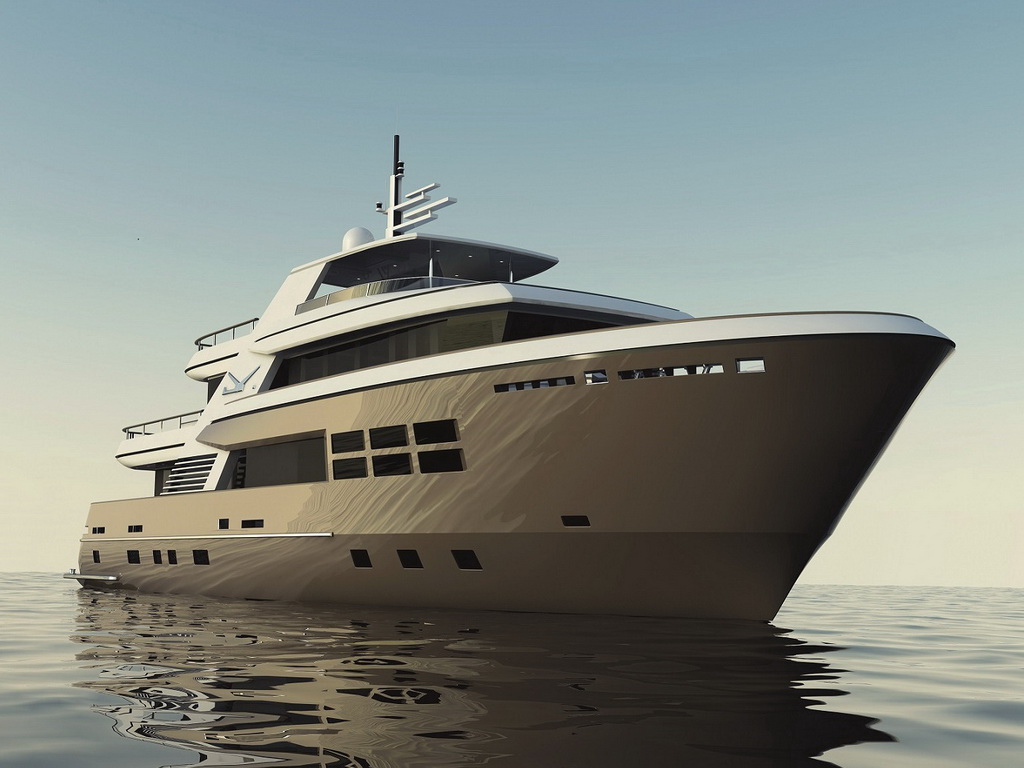
<< Back to overview
Still have questions about the yacht? Ask our yacht specialists!
The fields marked with * are mandatory fields for sending the request.
Here you can view privacy policy . Your data will only be used to contact you and will not be disclosed to third parties without your consent.
More new yachts from Bandido Yachts
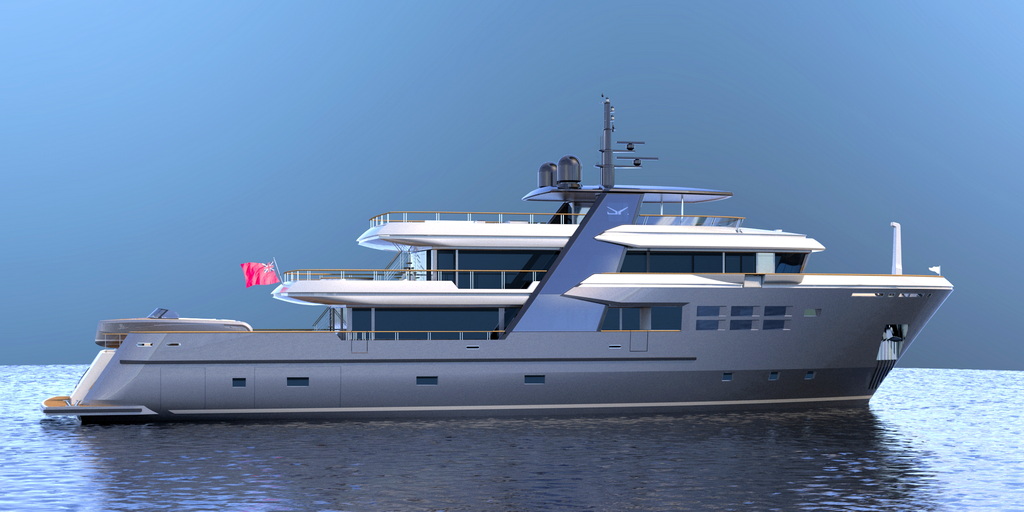
Our Pre-Owned Yachts from Bandido Yachts
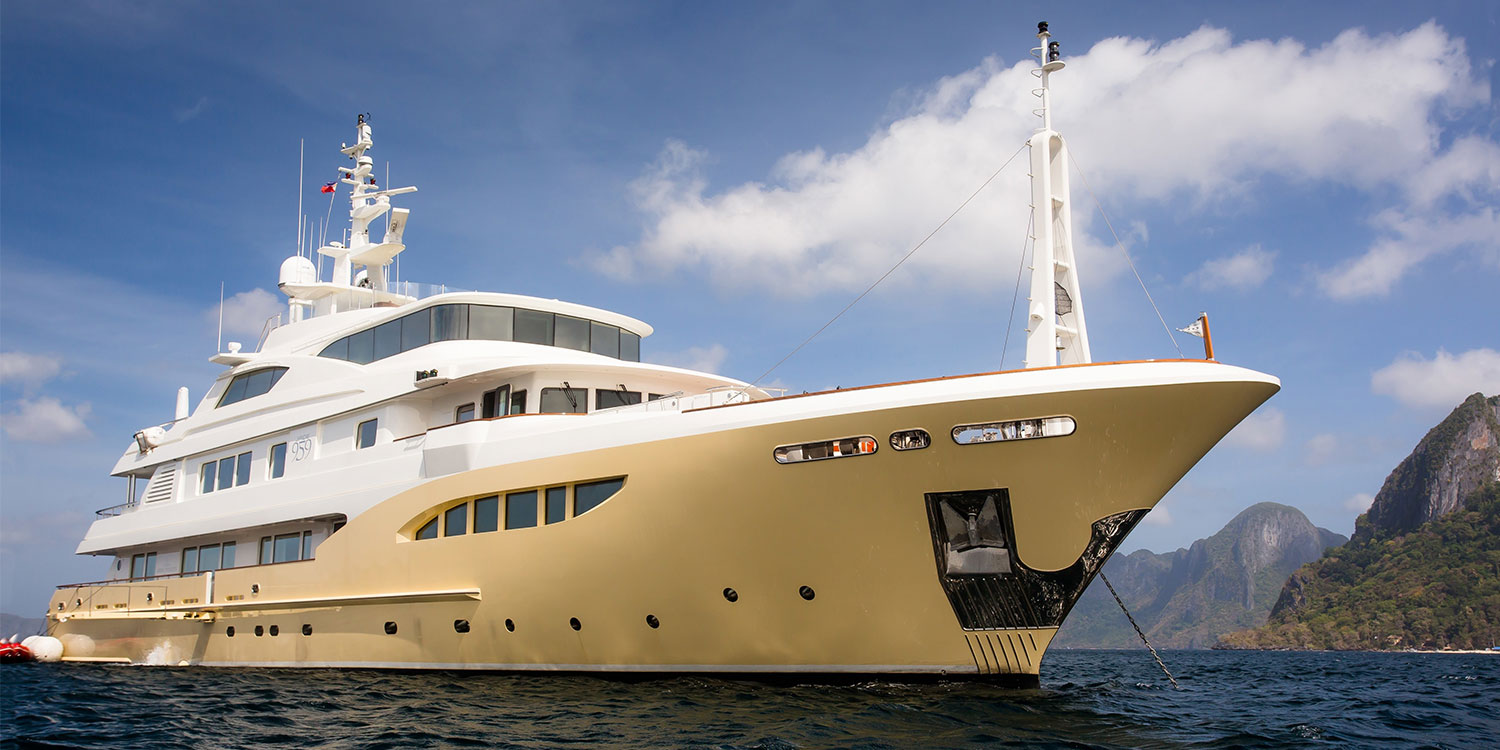
statistical problem solving tool
Please ensure that your password is at least 8 characters and contains each of the following:
- a special character: @$#!%*?&
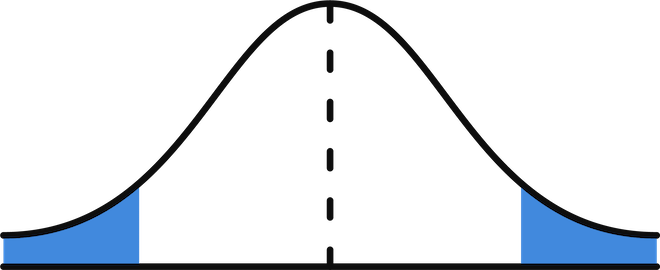
Step-by-Step Statistics Solutions
Get help on your statistics homework with our easy-to-use statistics calculators.
Here, you will find all the help you need to be successful in your statistics class. Check out our statistics calculators to get step-by-step solutions to almost any statistics problem. Choose from topics such as numerical summary, confidence interval, hypothesis testing, simple regression and more.
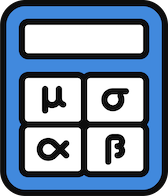
Statistics Calculators
Table and graph, numerical summary, basic probability, discrete distribution, continuous distribution, sampling distribution, confidence interval, hypothesis testing, two population, population variance, goodness of fit, analysis of variance, simple regression, multiple regression, time series analysis.
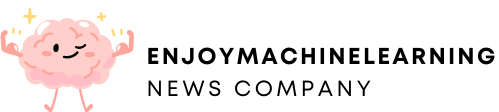
How to Solve Statistical Problems Efficiently [Master Your Data Analysis Skills]
- November 17, 2023
Are you tired of feeling overstimulated by statistical problems? Welcome – you have now found the perfect article.
We understand the frustration that comes with trying to make sense of complex data sets.
Let’s work hand-in-hand to unpack those statistical secrets and find clarity in the numbers.
Do you find yourself stuck, unable to move forward because of statistical roadblocks? We’ve been there too. Our skill in solving statistical problems will help you find the way in through the toughest tough difficulties with confidence. Let’s tackle these problems hand-in-hand and pave the way to success.
As experts in the field, we know what it takes to conquer statistical problems effectively. This article is adjusted to meet your needs and provide you with the solutions you’ve been searching for. Join us on this voyage towards mastering statistics and unpack a world of possibilities.

Key Takeaways
- Data collection is the foundation of statistical analysis and must be accurate.
- Understanding descriptive and inferential statistics is critical for looking at and interpreting data effectively.
- Probability quantifies uncertainty and helps in making smart decisionss during statistical analysis.
- Identifying common statistical roadblocks like misinterpreting data or selecting inappropriate tests is important for effective problem-solving.
- Strategies like understanding the problem, choosing the right tools, and practicing regularly are key to tackling statistical tough difficulties.
- Using tools such as statistical software, graphing calculators, and online resources can aid in solving statistical problems efficiently.
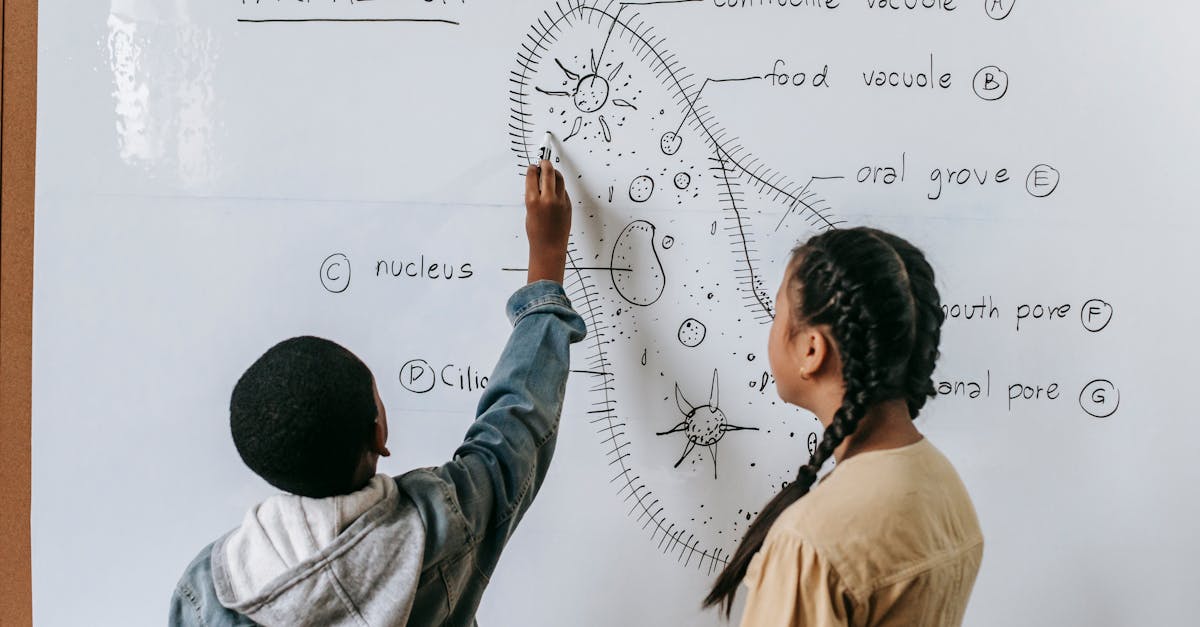
Understanding Statistical Problems
When exploring the world of statistics, it’s critical to assimilate the nature of statistical problems. These problems often involve interpreting data, looking at patterns, and drawing meaningful endings. Here are some key points to consider:
- Data Collection: The foundation of statistical analysis lies in accurate data collection. Whether it’s surveys, experiments, or observational studies, gathering relevant data is important.
- Descriptive Statistics: Understanding descriptive statistics helps in summarizing and interpreting data effectively. Measures such as mean, median, and standard deviation provide useful ideas.
- Inferential Statistics: This branch of statistics involves making predictions or inferences about a population based on sample data. It helps us understand patterns and trends past the observed data.
- Probability: Probability is huge in statistical analysis by quantifying uncertainty. It helps us assess the likelihood of events and make smart decisionss.
To solve statistical problems proficiently, one must have a solid grasp of these key concepts.
By honing our statistical literacy and analytical skills, we can find the way in through complex data sets with confidence.
Let’s investigate more into the area of statistics and unpack its secrets.
Identifying Common Statistical Roadblocks
When tackling statistical problems, identifying common roadblocks is important to effectively find the way in the problem-solving process.
Let’s investigate some key problems individuals often encounter:
- Misinterpretation of Data: One of the primary tough difficulties is misinterpreting the data, leading to erroneous endings and flawed analysis.
- Selection of Appropriate Statistical Tests: Choosing the right statistical test can be perplexing, impacting the accuracy of results. It’s critical to have a solid understanding of when to apply each test.
- Assumptions Violation: Many statistical methods are based on certain assumptions. Violating these assumptions can skew results and mislead interpretations.
To overcome these roadblocks, it’s necessary to acquire a solid foundation in statistical principles and methodologies.
By honing our analytical skills and continuously improving our statistical literacy, we can adeptly address these tough difficulties and excel in statistical problem-solving.
For more ideas on tackling statistical problems, refer to this full guide on Common Statistical Errors .
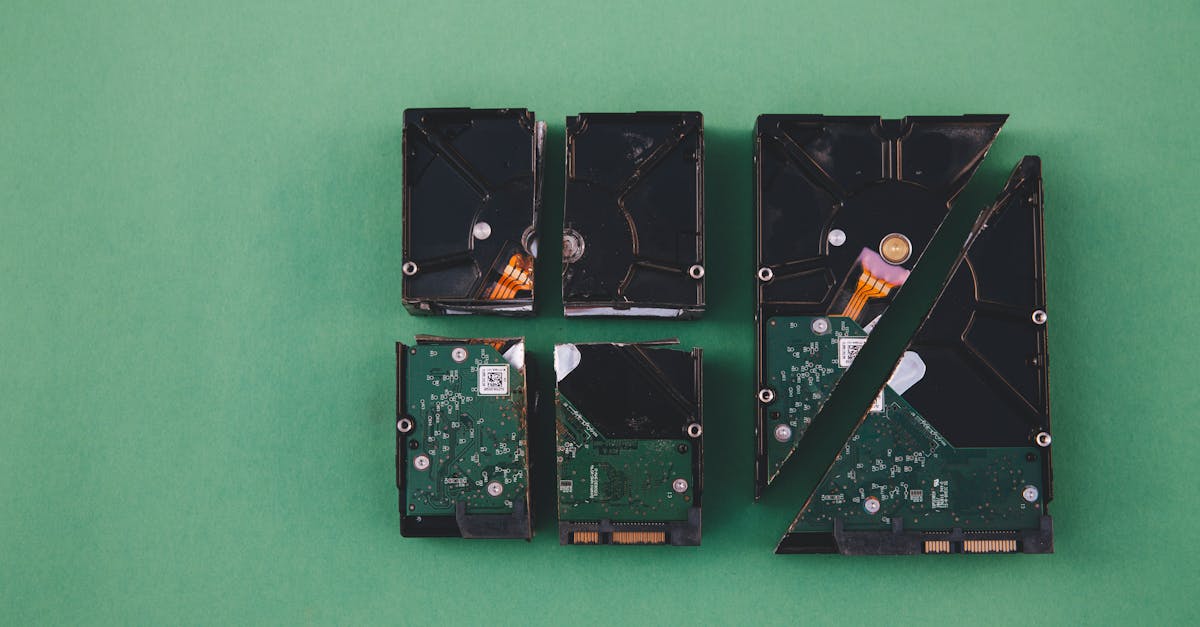
Strategies for Tackling Statistical Tough difficulties
When facing statistical tough difficulties, it’s critical to employ effective strategies to find the way in through complex data analysis.
Here are some key approaches to tackle statistical problems:
- Understand the Problem: Before exploring analysis, ensure a clear comprehension of the statistical problem at hand.
- Choose the Right Tools: Selecting appropriate statistical tests is important for accurate results.
- Check Assumptions: Verify that the data meets the assumptions of the chosen statistical method to avoid skewed outcomes.
- Consult Resources: Refer to reputable sources like textbooks or online statistical guides for assistance.
- Practice Regularly: Improve statistical skills through consistent practice and application in various scenarios.
- Seek Guidance: When in doubt, seek advice from experienced statisticians or mentors.
By adopting these strategies, individuals can improve their problem-solving abilities and overcome statistical problems with confidence.
For further ideas on statistical problem-solving, refer to a full guide on Common Statistical Errors .
Tools for Solving Statistical Problems
When it comes to tackling statistical tough difficulties effectively, having the right tools at our disposal is important.
Here are some key tools that can aid us in solving statistical problems:
- Statistical Software: Using software like R or Python can simplify complex calculations and streamline data analysis processes.
- Graphing Calculators: These tools are handy for visualizing data and identifying trends or patterns.
- Online Resources: Websites like Kaggle or Stack Overflow offer useful ideas, tutorials, and communities for statistical problem-solving.
- Textbooks and Guides: Referencing textbooks such as “Introduction to Statistical Learning” or online guides can provide in-depth explanations and step-by-step solutions.
By using these tools effectively, we can improve our problem-solving capabilities and approach statistical tough difficulties with confidence.
For further ideas on common statistical errors to avoid, we recommend checking out the full guide on Common Statistical Errors For useful tips and strategies.
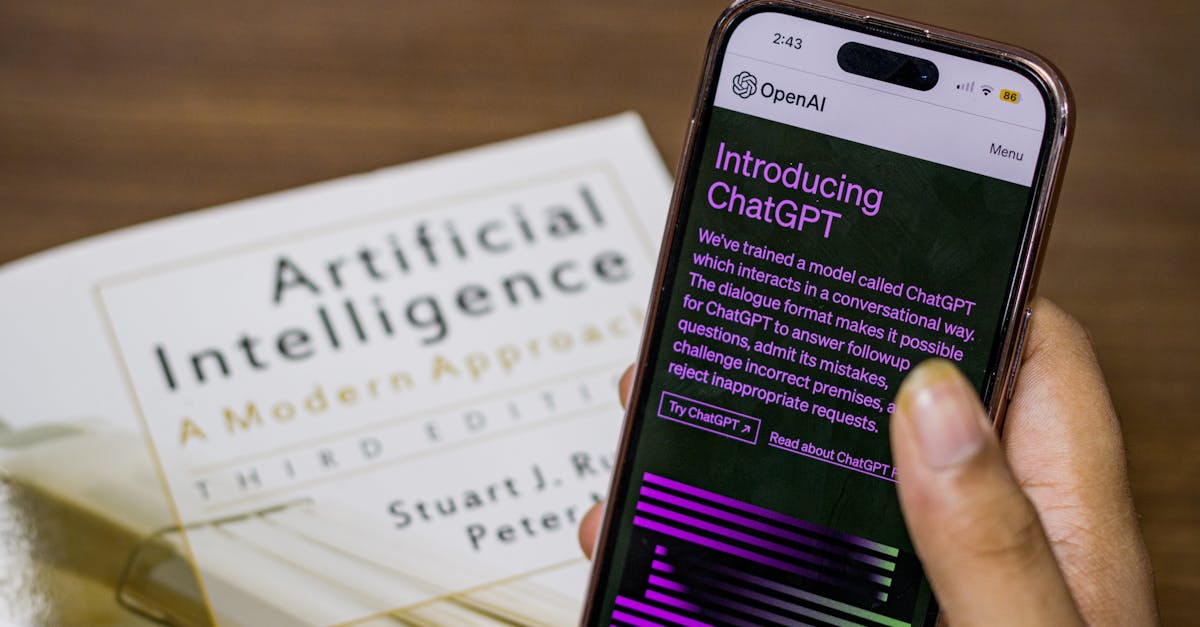
Putting in place Effective Solutions
When approaching statistical problems, it’s critical to have a strategic plan in place.
Here are some key steps to consider for putting in place effective solutions:
- Define the Problem: Clearly outline the statistical problem at hand to understand its scope and requirements fully.
- Collect Data: Gather relevant data sets from credible sources or conduct surveys to acquire the necessary information for analysis.
- Choose the Right Model: Select the appropriate statistical model based on the nature of the data and the specific question being addressed.
- Use Advanced Tools: Use statistical software such as R or Python to perform complex analyses and generate accurate results.
- Validate Results: Verify the accuracy of the findings through strict testing and validation procedures to ensure the reliability of the endings.
By following these steps, we can streamline the statistical problem-solving process and arrive at well-informed and data-driven decisions.
For further ideas and strategies on tackling statistical tough difficulties, we recommend exploring resources such as DataCamp That offer interactive learning experiences and tutorials on statistical analysis.
Recent Posts
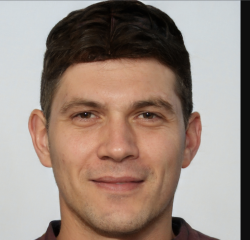
- Crack Puzzle Questions for Machine Learning Interview [Ace Your Interview Now] - April 24, 2024
- Do Product Managers Make More Than Software Engineers? [Uncover the Salary Truth] - April 24, 2024
- How is Regression Testing Done in Software Testing? [Boost Your Testing Efficiency Now] - April 24, 2024
Trending now
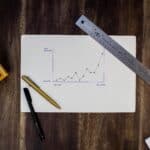
Have questions? Contact us at (770) 518-9967 or [email protected]
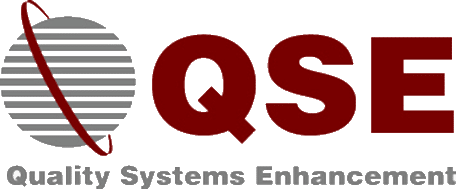
Statistical Problem Solving (SPS)
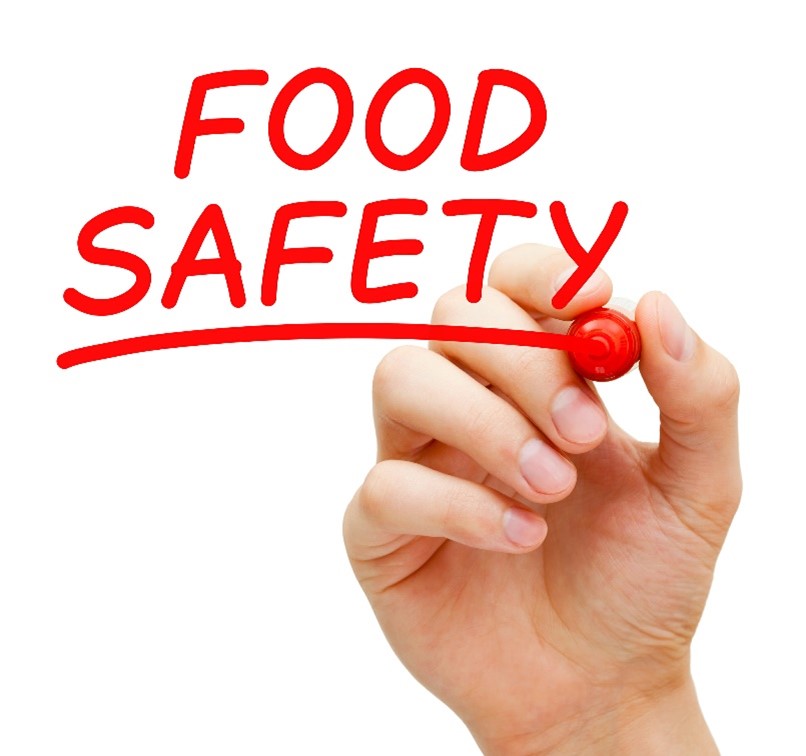
- Statistical Problem Solving
Problem solving in any organization is a problem. Nobody wants to own the responsibility for a problem and that is the reason, when a problem shows up fingers may be pointing at others rather than self.

This is a natural human instinctive defense mechanism and hence cannot hold it against any one. However, it is to be realized the problems in industry are real and cannot be wished away, solution must be sought either by hunch or by scientific methods. Only a systematic disciplined approach for defining and solving problems consistently and effectively reveal the real nature of a problem and the best possible solutions .
A Chinese proverb says, “ it is cheap to do guesswork for solution, but a wrong guess can be very expensive”. This is to emphasize that although occasional success is possible trough hunches gained through long years of experience in doing the same job, but a lasting solution is possible only through scientific methods.
One of the major scientific method for problem solving is through Statistical Problem Solving (SPS) this method is aimed at not only solving problems but may be used for improvement on existing situation. It involves a team armed with process and product knowledge, having willingness to work together as a team, can undertake selection of some statistical methods, have willingness to adhere to principles of economy and willingness to learn along the way.
Statistical Problem Solving (SPS) could be used for process control or product control. In many situations, the product would be customer dictated, tried, tested and standardized in the facility may involve testing at both internal to facility or external to facility may be complex and may require customer approval for changes which could be time consuming and complex. But if the problem warrants then this should be taken up.
Process controls are lot simpler than product control where SPS may be used effectively for improving profitability of the industry, by reducing costs and possibly eliminating all 7 types of waste through use of Kaizen and lean management techniques.
The following could be used as 7 steps for Statistical Problem Solving (SPS)
- Defining the problem
- Listing variables
- Prioritizing variables
- Evaluating top few variables
- Optimizing variable settings
- Monitor and Measure results
- Reward/Recognize Team members
Defining the problem: Source for defining the problem could be from customer complaints, in-house rejections, observations by team lead or supervisor or QC personnel, levels of waste generated or such similar factors.
Listing and prioritizing variables involves all features associated with the processes. Example temperature, feed and speed of the machine, environmental factors, operator skills etc. It may be difficult to try and find solution for all variables together. Hence most probable variables are to be selected based on collective wisdom and experience of the team attempting to solve the problem.
Collection of data: Most common method in collecting data is the X bar and R charts. Time is used as the variable in most cases and plotted on X axis, and other variables such as dimensions etc. are plotted graphically as shown in example below.
Once data is collected based on probable list of variables, then the data is brought to the attention of the team for brainstorming on what variables are to be controlled and how solution could be obtained. In other words , optimizing variables settings . Based on the brainstorming session process control variables are evaluated using popular techniques like “5 why”, “8D”, “Pareto Analysis”, “Ishikawa diagram”, “Histogram” etc. The techniques are used to limit variables and design the experiments and collect data again. Values of variables are identified from data which shows improvement. This would lead to narrowing down the variables and modify the processes, to achieve improvement continually. The solutions suggested are to be implemented and results are to be recorded. This data is to be measured at varying intervals to see the status of implementation and the progress of improvement is to be monitored till the suggested improvements become normal routine. When results indicate resolution of problem and the rsults are consistent then Team memebres are to be rewarded and recognized to keep up their morale for future projects.
Who Should Pursue SPS
- Statistical Problem Solving can be pursued by a senior leadership group for example group of quality executives meeting weekly to review quality issues, identify opportunities for costs saving and generate ideas for working smarter across the divisions
- Statistical Problem solving can equally be pursued by a staff work group within an institution that possesses a diversity of experience that can gather data on various product features and tabulate them statistically for drawing conclusions
- The staff work group proposes methods for rethinking and reworking models of collaboration and consultation at the facility
- The senior leadership group and staff work group work in partnership with university faculty and staff to identify research communications and solve problems across the organization
Benefits of Statistical Problem Solving
- Long term commitment to organizations and companies to work smarter.
- Reduces costs, enhances services and increases revenues.
- Mitigating the impact of budget reductions while at the same time reducing operational costs.
- Improving operations and processes, resulting in a more efficient, less redundant organization.
- Promotion of entrepreneurship intelligence, risk taking corporations and engagement across interactions with business and community partners.
- A culture change in a way a business or organization collaborates both internally and externally.
- Identification and solving of problems.
- Helps to repetition of problems
- Meets the mandatory requirement for using scientific methods for problem solving
- Savings in revenue by reducing quality costs
- Ultimate improvement in Bottom -Line
- Improvement in teamwork and morale in working
- Improvement in overall problem solving instead of harping on accountability
Business Impact
- Scientific data backed up problem solving techniques puts the business at higher pedestal in the eyes of the customer.
- Eradication of over consulting within businesses and organizations which may become a pitfall especially where it affects speed of information.
- Eradication of blame game
QSE’s Approach to Statistical Problem Solving
By leveraging vast experience, it has, QSE organizes the entire implementation process for Statistical Problem Solving in to Seven simple steps
- Define the Problem
- List Suspect Variables
- Prioritize Selected Variables
- Evaluate Critical Variables
- Optimize Critical Variables
- Monitor and Measure Results
- Reward/Recognize Team Members
- Define the Problem (Vital Few -Trivial Many):
List All the problems which may be hindering Operational Excellence . Place them in a Histogram under as many categories as required.
Select Problems based on a simple principle of Vital Few that is select few problems which contribute to most deficiencies within the facility
QSE advises on how to Use X and R Charts to gather process data.
- List Suspect Variables:
QSE Advises on how to gather data for the suspect variables involving cross functional teams and available past data
- Prioritize Selected Variables Using Cause and Effect Analysis:
QSE helps organizations to come up prioritization of select variables that are creating the problem and the effect that are caused by them. The details of this exercise are to be represented in the Fishbone Diagram or Ishikawa Diagram

- Evaluate Critical Variables:
Use Brain Storming method to use critical variables for collecting process data and Incremental Improvement for each selected critical variable
QSE with its vast experiences guides and conducts brain storming sessions in the facility to identify KAIZEN (Small Incremental projects) to bring in improvements. Create a bench mark to be achieved through the suggested improvement projects
- Optimize Critical Variable Through Implementing the Incremental Improvements:
QSE helps facilities to implement incremental improvements and gather data to see the results of the efforts in improvements
- Monitor and Measure to Collect Data on Consolidated incremental achievements :
Consolidate and make the major change incorporating all incremental improvements and then gather data again to see if the benchmarks have been reached
QSE educates and assists the teams on how these can be done in a scientific manner using lean and six sigma techniques
QSE organizes verification of Data to compare the results from the original results at the start of the projects. Verify if the suggestions incorporated are repeatable for same or better results as planned
Validate the improvement project by multiple repetitions
- Reward and Recognize Team Members:
QSE will provide all kinds of support in identifying the great contributors to the success of the projects and make recommendation to the Management to recognize the efforts in a manner which befits the organization to keep up the morale of the contributors.
Need Certification?
Quality System Enhancement has been a leader in global certification services for the past 30 years . With more than 800 companies successfully certified, our proprietary 10-Step Approach™ to certification offers an unmatched 100% success rate for our clients.
Cdfa proposition 12 – farm animal confinement.
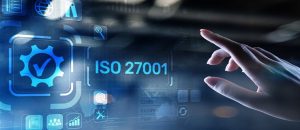
ISO 27001 Flyer
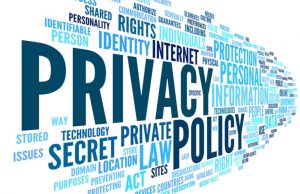
ISO 27701 Flyer
Have a question, sign up for our newsletter.
Hear about the latest industry trends from the QSE team of experts. Receive special offers for training services and invitations to free webinars.
ISO Standards
- ISO 9001:2015
- ISO 10993-1:2018
- ISO 13485:2016
- ISO 14001:2015
- ISO 15189:2018
- ISO 15190:2020
- ISO 15378:2017
- ISO/IEC 17020:2012
- ISO/IEC 17025:2017
- ISO 20000-1:2018
- ISO 22000:2018
- ISO 22301:2019
- ISO 27001:2015
- ISO 27701:2019
- ISO 28001:2007
- ISO 37001:2016
- ISO 45001:2018
- ISO 50001:2018
- ISO 55001:2014
Telecommunication Standards
- TL 9000 Version 6.1
Automotive Standards
- IATF 16949:2016
- ISO/SAE 21434:2021
Aerospace Standards
Forestry standards.
- FSC - Forest Stewardship Council
- PEFC - Program for the Endorsement of Forest Certification
- SFI - Sustainable Forest Initiative
Steel Construction Standards
Food safety standards.
- FDA Gluten Free Labeling & Certification
- Hygeine Excellence & Sanitation Excellence
GFSI Recognized Standards
- BRC Version 9
- FSSC 22000:2019
- Hygeine Excellent & Sanitation Excellence
- IFS Version 7
- SQF Edition 9
- All GFSI Recognized Standards for Packaging Industries
Problem Solving Tools
- Corrective & Preventative Actions
- Root Cause Analysis
- Supplier Development
Excellence Tools
- Bottom Line Improvement
- Customer Satisfaction Measurement
- Document Simplification
- Hygiene Excellence & Sanitation
- Lean & Six Sigma
- Malcom Baldridge National Quality Award
- Operational Excellence
- Safety (including STOP and OHSAS 45001)
- Sustainability (Reduce, Reuse, & Recycle)
- Total Productive Maintenance
Other Standards
- California Transparency Act
- Global Organic Textile Standard (GOTS)
- Hemp & Cannabis Management Systems
- Recycling & Re-Using Electronics
- ESG - Environmental, Social & Governance
- CDFA Proposition 12 Animal Welfare
Simplification Delivered™
QSE has helped over 800 companies across North America achieve certification utilizing our unique 10-Step Approach ™ to management system consulting. Schedule a consultation and learn how we can help you achieve your goals as quickly, simply and easily as possible.
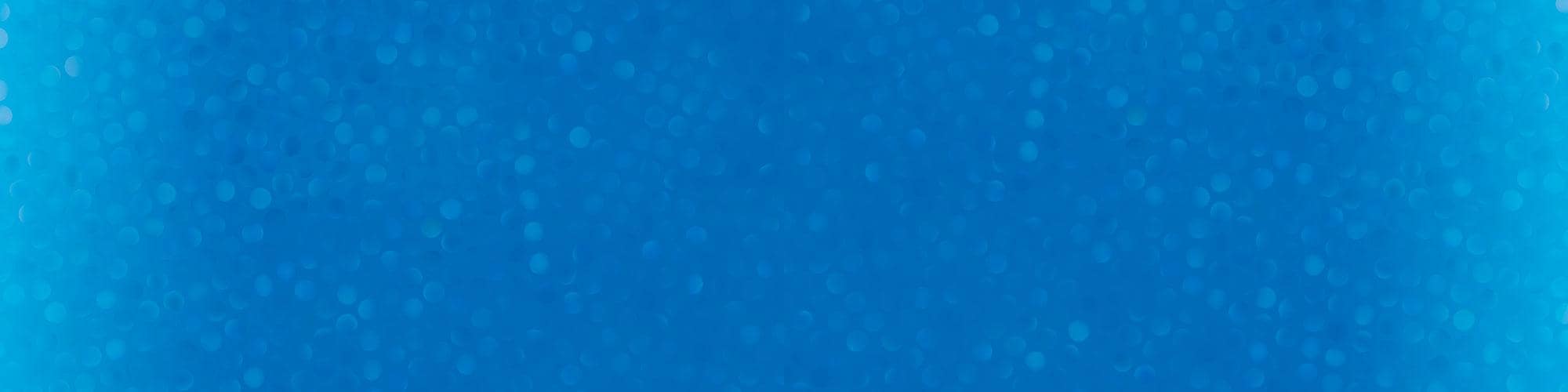
Statistical Thinking for Industrial Problem Solving
A free online statistics course.
Back to Course Overview
Statistical Thinking and Problem Solving
Statistical thinking is vital for solving real-world problems. At the heart of statistical thinking is making decisions based on data. This requires disciplined approaches to identifying problems and the ability to quantify and interpret the variation that you observe in your data.
In this module, you will learn how to clearly define your problem and gain an understanding of the underlying processes that you will improve. You will learn techniques for identifying potential root causes of the problem. Finally, you will learn about different types of data and different approaches to data collection.
Estimated time to complete this module: 2 to 3 hours
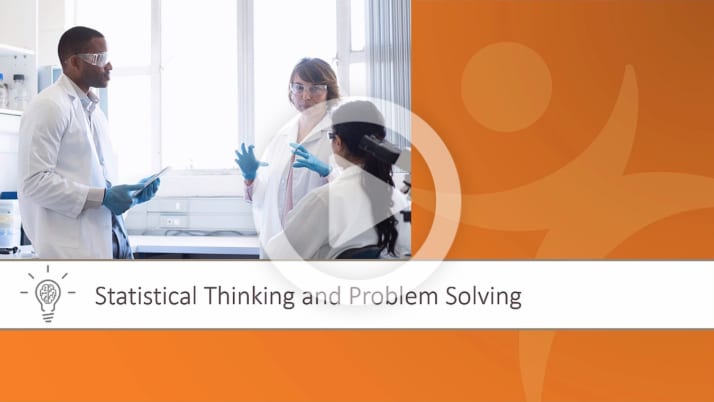
Statistical Thinking and Problem Solving Overview (0:36)
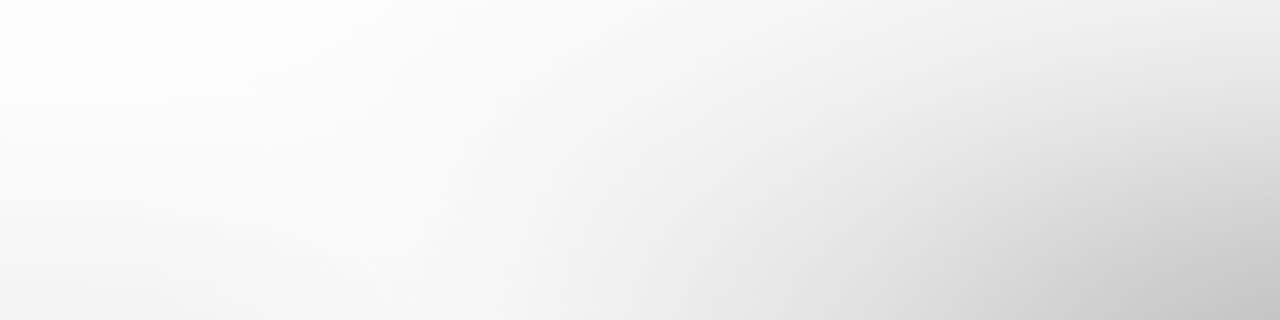
Specific topics covered in this module include:
Statistical thinking.
- What is Statistical Thinking
Problem Solving
- Overview of Problem Solving
- Types of Problems
- Defining the Problem
- Goals and Key Performance Indicators
- The White Polymer Case Study
Defining the Process
- What is a Process?
- Developing a SIPOC Map
- Developing an Input/Output Process Map
- Top-Down and Deployment Flowcharts
Identifying Potential Root Causes
- Tools for Identifying Potential Causes
- Brainstorming
- Multi-voting
- Using Affinity Diagrams
- Cause-and-Effect Diagrams
- The Five Whys
- Cause-and-Effect Matrices
Compiling and Collecting Data
- Data Collection for Problem Solving
- Types of Data
- Operational Definitions
- Data Collection Strategies
- Importing Data for Analysis
- Topical Articles =>
- PMP Certification
- CAPM Certification
- Agile Training
- Corporate Training
- Project Management Tools
Home / Six Sigma / The Six Sigma Approach: A Data-Driven Approach To Problem-Solving
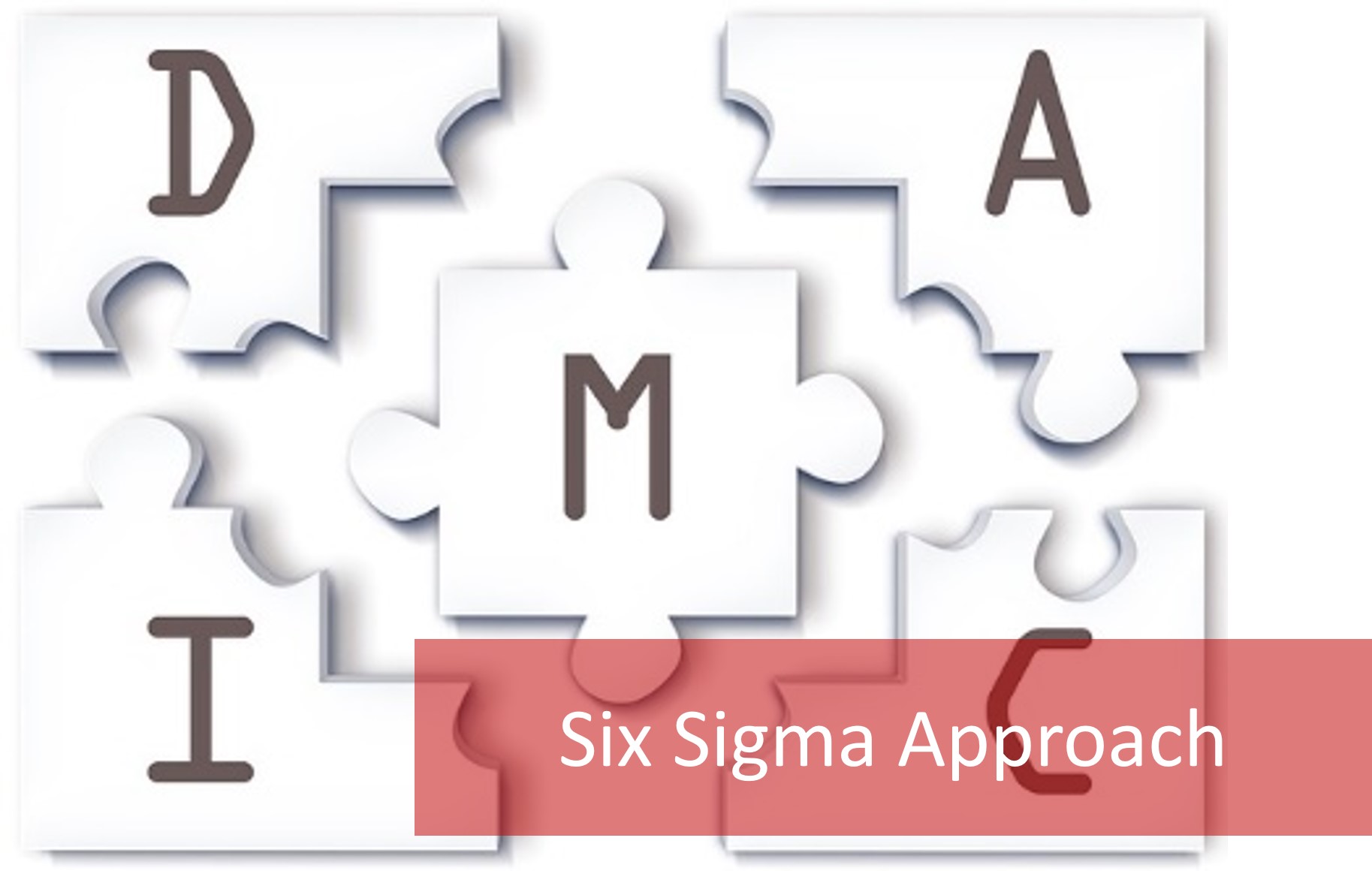
The Six Sigma Approach: A Data-Driven Approach To Problem-Solving
If you are a project manager or an engineer, you may have heard of the 6 Sigma approach to problem-solving by now. In online Six Sigma courses that teach the Six Sigma principles , you will learn that a data-driven approach to problem-solving , or the Six Sigma approach, is a better way to approach problems. If you have a Six Sigma Green Belt certification then you will be able to turn practical problems into practical solutions using only facts and data.
Attend our 100% Online & Self-Paced Free Six Sigma Training .

This approach does not have room for gut feel or jumping to conclusions. However, if you are reading this article, you are probably still curious about the Six Sigma approach to problem-solving.
What is the Six Sigma Approach?
Let’s see what the Six Sigma approach or thinking is. As briefly described in free Six Sigma Green Belt Certification training , this approach is abbreviated as DMAIC. The DMAIC methodology of Six Sigma states that all processes can be Defined, Measured, Analyzed, Improved and Controlled . These are the phases in this approach. Collectively, it is called as DMAIC. Every Six Sigma project goes through these five stages. In the Define phase, the problem is looked at from several perspectives to identify the scope of the problem. All possible inputs in the process that may be causing the problem are compared and the critical few are identified. These inputs are Measured and Analyzed to determine whether they are the root cause of the problem. Once the root cause has been identified, the problem can be fixed or Improved. After the process has been improved, it must be controlled to ensure that the problem has been fixed in the long-term.
Check our Six Sigma Training Video
Every output (y) is a function of one or multiple inputs (x)
Any process which has inputs (X), and delivers outputs (Y) comes under the purview of the Six Sigma approach. X may represent an input, cause or problem, and Y may represent output, effect or symptom . We can say here that controlling inputs will control outputs. Because the output Y will be generated based on the inputs X.
This Six Sigma approach is called Y=f(X) thinking. It is the mechanism of the Six Sigma. Every problematic situation has to be converted into this equation. It may look difficult but it is just a new way of looking at the problem.
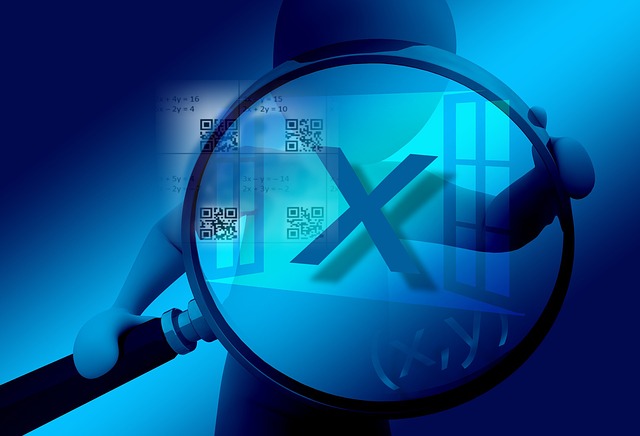
Please remember that the context of relating X and Y to each other would vary from situation to situation. If X is your input, then only Y becomes your output. If X is your cause, Y will not be regarded as the output. If X is your input, Y cannot be called as an effect.
Let’s go further. The equation of Y=f(X) could involve several subordinate outputs, perhaps as leading indicators of the overall “Big Y.” For example, if TAT was identified as the Big Y, the improvement team may examine leading indicators, such as Cycle Time; Lead Time as little Ys. Each subordinate Y may flow down into its own Y= f(X) relationship wherein some of the critical variables for one also may affect another little Y. That another little variable could be your potential X or critical X.
A practical vs. a statistical problem and solution
In the Six Sigma approach, the practical problem is the problem or pain area which has been persisting on your production or shop floor. You will need to c onvert this practical problem into a statistical problem. A statistical problem is the problem that is addressed with facts and data analysis methods. Just a reminder, the measurement, and analysis of a statistical problem is completed in Measure and Analyze phase of the Six Sigma approach or DMAIC.
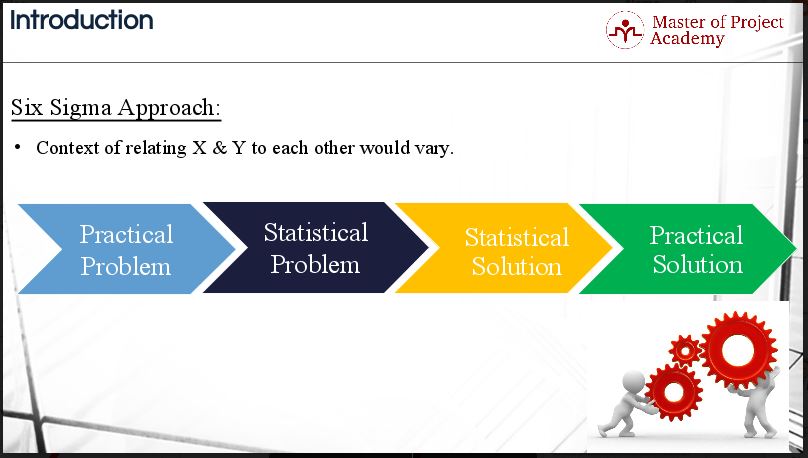
In this approach, the statistical problem will then be converted into a statistical solution. It is the solution with a known confidence or risk levels versus an “I think” solution. This solution is not based on gut feeling. It’s a completely data-driven solution because it was found using the Six Sigma approach.
A Six Sigma approach of DMAIC project would assist you to convert your Practical Problem into Statistical Problem and then your Statistical Problem into Statistical Solution. The same project would also give you the Practical Solutions that aren’t complex and too difficult to implement. That’s how the Six Sigma approach works.
This approach may seem like a lot of work. Wouldn’t it be better to guess what the problem is and work on it from there? That would certainly be easier, but consider that randomly choosing a root cause of a problem may lead to hard work that doesn’t solve the problem permanently. You may be working to create a solution that will only fix 10% of the problem while following the Six Sigma approach will help you to identify the true root cause of the problem . Using this data-driven Six Sigma approach, you will only have to go through the problem-solving process once.
The Six Sigma approach is a truly powerful problem-solving tool. By working from a practical problem to a statistical problem, a statistical solution and finally a practical solution, you will be assured that you have identified the correct root cause of the problem which affects the quality of your products. The Six Sigma approach follows a standard approach – DMAIC – that helps the problem-solver to convert the practical problem into a practical solution based on facts and data . It’s very important to note that the Six Sigma approach is not a one-man show. Problem solving should be approached as a team with subject matter experts and decicion makers involved.
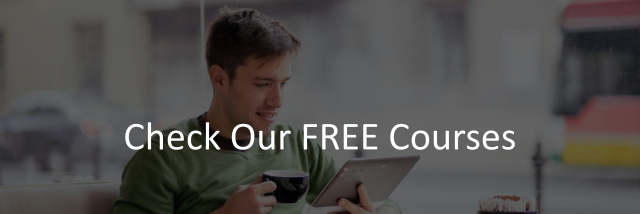
Popular Problems
Popular statistics problems.
Please add a message.
Message received. Thanks for the feedback.
- DRIVING WORLDWIDE BUSINESS EXCELLENCE

- Latest in Omnex
- Electric & Autonomous Vehicles
- Medical Devices
- High-Tech / Semiconductors
- Telecom - SCS 9001 / TIA
- General Manufacturing
- Consulting, Implementation, & Coaching
- Training and Competency Development
- Digital Collaboration Software Platform
- Quality Outsourcing
- Product Design
- About Omnex
- Omnex Group
- Omnex Leadership Team
- Worldwide Offices
- Awards and Accreditations
- Client List
- Standards & Methods
- Seven Statistical Tools
Seven Statistical Tools Overview
- Register for courses 60 days in advance and get 10% off this price.
- Register for courses 30 days in advance and get 5% off this price
- Note:Pricing is dependent on location and may vary.
Course Duration: 1 Day - 8 Hours/day
This one-day seminar provides training on the seven statistical tools. These tools are used in problem solving and continual improvement endeavors. The seven statistical tools were first introduced by Dr. Ishikawa when he introduced problem solving in Japan. Dr. Ishikawa put together seven simple tools that were already available for use. While the entire tool set is not named after him, the cause and effect diagram or the fishbone diagram which has been attributed to his creation has often been also called the “Ishikawa” diagram. When using the seven statistical tools, it is always good to remember Dr. Ishikawa’s words to those doing improvement – “speak with data”. Omnex teaches this course as a standalone one day or in conjunction with our problem solving courses.
Learning Objectives
· Understand the seven statistical tools. What are they? How are they used?
· Understand the different uses of each tool and how it can be used for problem solving or improvement
· The role of the seven statistical tools in a PDCA cycle
Course Outline
· Introduction and Background
· Understanding the Process
o Breakout Exercise 1: Process Flow
· Data Collection and Analysis
o Checksheets
o Breakout Exercise 2: Histograms
o Breakout Exercise 3: Run Charts
o Breakout Exercise 4: Control Charts
· Data Analysis
o Breakout Exercise 5: Pareto Charts
o Breakout Exercise 6: Cause and Effect Diagrams
o Graphs
· Process Analysis
o Scatter Diagrams
o Stratification
· Summary and Process Control
Who Should Attend
This seminar is designed for individuals and teams who are responsible for identifying, solving and eliminating problems that hinder quality, productivity and Customer Satisfaction. All personnel involved with improvement would benefit from this course.
Course Materials
Each participant will receive a seminar manual, including a complete package of problem solving worksheets and checklists for each step of the process, as well as all team exercise materials.
Note: Omnex does not provide copies of standard(s) during training courses, but clients are encouraged to have their own copy.
Pre-Requisite
Participants should possess the ability and/or desire to work with small groups of people in a cooperative and productive manner to achieve planned objectives.
Upcoming Training
Related courses.
Problem Solving - Employing the 8D Methodology
Geometric Dimensioning and Tolerance (GD&T) - Gage Design
Design of Experiments (DOE)
Tolerance Stack-Up Analysis (GD&T Application)
Effective Problem Solving (EPS) - Problem Solving Methodology and Concepts
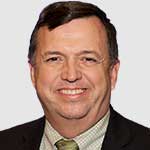
MICHAEL DOWN
Michael Down is a Senior Consultant with extensive Engineering, Quality and Reliability experience. Whether it may be in Product Development, Manufacturing, or Quality Management Systems, his greatest desires are to improve clients understanding and improving system to provide optimum performance, quality and durability of the product or process design. He also well understands the need for reducing costs while continually improving quality & compliance/conformance.
Mr. Down has extensive experience working in the automotive industry from manufacturing and assembly to vehicle design development and software/hardware Reliability, DMFEA and PFMEAs. He spent over 32 years working for GM in the quality engineering, statistical problem solving and continuous improvement and teaching. Have taught thousands of employees over the years in relation to FMEA, Probability and Statistics, SPC, System Thinking, Deming, Reliability, and statistical problem solving. Used SPC principles to manufacturing processes at GM, increasing line efficiency and reducing cost, saving GM millions of dollars. Applied DOE to advanced design and process development, identifying critical variables and optimizing process performance. Statistically solved process and product issues in relation to casting, metal fabrication, electronics, injection molding and SMC plastics. Also, statistically solved issues in relation to stamping, heat treating, paint, and in relation to issues with oxygen sensors.
In addition, he was instrumental in the development of the GM Powertrain PFMEA guidelines. Managed quality engineers in manufacturing and assembling. Was a part of the leadership group that directed the Statistical Network within GM (assisted in facilitating Deming seminars and assisted in the training of his courses and seminar) Mike also represented GM at both SAE and AIAG, providing extensive guidance and input to the development of Global Automotive Standards reference documents on Quality and Core tools, including PFMEA, APQP/CP, PPAP, SPC, MSA, and DRBFM reference documents. Mike has been involved with FMEA standards and including developing and teaching FMEAs since the 1990s. Today, Mike is actively working on the SAE J1739 committee updating the FMEA standard to reflect AIAG-VDA FMEA.
Specialties: Training and support the development of DFMEAs and PFMEAs for FMEA 4th edition and AIAG-VDA FMEA. Lead for PQMS training development, IQFMEA tech expert, taught and developed DFA and Robust engineering courses. Deming expert, facilitation and application, DOE trainer and implementer, Represent GM at SAE and at AIAG. Expert in the area of AIAG-VDA FMEA, SPC, MSA, FMEA 4th edition, and DRBFM
EDUCATION Bachelor of Science, Electrical Engineering, MTU, Bachelor Industrial Management in Electronic Engineering Technology from Baker College, and a Master Degree in Applied Statistics from Oakland University
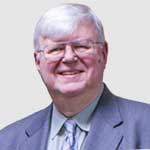
GREG GRUSKA
Greg Gruska is the Omnex Champion for APQP, PPAP, FMEA, ISO 26262, Lean Six Sigma and a Fellow of the American Society for Quality (ASQ). His strength in ISO 26262 is a strong understanding and experience in systems engineering and reliability/safety analysis in both hardware and software development. Greg managed the Quality Engineering Activity at Chevrolet. This group provided benchmarking, quality engineering and statistical support to all divisional and corporate activities and their suppliers. Besides the application of statistics within the design, manufacturing, and support environments, this group was active in the development of new technologies and training in these areas. Greg additional served as a Divisional and Corporate consultant in Statistical Engineering and Management. He has traveled extensively in assisting engineering, financial, and support staffs and manufacturing plants in the investigation and solution of problems affecting quality, new product development, product failures and customer satisfaction.
Greg is also an active/writing member of the MSA, SPC, FMEA, and EFMEA Manual subcommittees of the American Automotive industry�s Supplier Quality Requirements Task Force which is part of the international task force governing TS-16949. Greg is an adjunct professor at Madonna University. He has advanced degrees in mathematics and engineering from the University of Detroit, Michigan State University and Wayne State University. He was the Deming Memorial Lecturer at the Sheffield Hallam University for the year 2000.
Greg is a charter member of the Greater Detroit Deming Study Group and the W. E. Deming Institute. He is an ASQ certified Quality Engineer, a licensed Professional Engineer (CA - Quality) and a member of the Board of Examiners of and Judge for the Michigan Quality Leadership Award (1994-2011). Greg is on the writing committee of AIAG on FMEA, a member of the SAE Functional Safety Committee (J2980) and is considered one of the foremost authorities on risk management in the world. He has considerable hardware and software experience in Automotive applications.
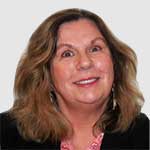
MARY E. ROWZEE, ASQ FELLOW
Mary Rowzee is an Omnex consultant with extensive experience and achievements in Quality Systems development, implementation and auditing to ISO 9000 series and IATF 16949 standards; Six Sigma Black Belt Problem Solving and Advance Quality Tools including: Design and Process FMEA, Design and Process Verification and Test Planning, Complex Statistical Analyses and Reliability Prediction, Modelling and Risk Reduction. Mary is a writing member of AIAG-VDA FMEA 1st edition and the Core Tools Guidelines: SPC 2nd edition, MSA 4th edition, EFMEA 1st edition, PPAP 4th edition and APQP 2nd edition.
Mary has been actively leading industry practices and application of ISO 26262 Functional Safety Standard for Electrical/ Electronic Products; Software FMEAs, ASPICE, CMMI and Quality; Supplemental Monitoring and Systems Response (MSR) FMEAs; Safety of the Intended Functionality (SOTIF) ISO 21448 and use of Safety Engineering tools (Reliability Block Diagrams, Hazard and Risk Analyses, Addressing ASIL rated risks) in Advanced Driver Assistance Systems (ADAS). She also served as GM Global representative on AIAG-VDA and SAE Quality Standards development teams.
Mary has worked for Daimler Chrysler Fiat, TRW and recently GM working as a Senior Engineer ADAS Electrical sub-systems quality for Autonomous Vehicles. She was the Quality and Reliability Resource on ADAS Electrical Sub-systems teams, used in Autonomous Vehicles. For GM she worked with internal and first tier supplier teams to develop Safety Analyses and Design FMEAs on Electrical, Mechanical and Software products in support of ISO 26262 requirements. Mary also assisted in the establishment and implementation of an aggressive Advanced Product Quality Process within GM and Supply Base. Additionally, at GM, she served as in-house consultant and coach to more than 5,000 product engineers in Six Sigma project development and implementation. Mary was an Operational Excellence Master for the GM Quality organization, leading and facilitating the highest impact, most financially significant corporate projects, in addition to teaching many courses on Six Sigma tools and techniques. Also at GM, Mary served as the Senior Leader for Global Design and Process FMEA. In this she revitalized the use of FMEAS within General Motors by developing and teaching all live and web based FMEA classes in North America and developing criteria and assessment processes for Global FMEA software selection.
While at Daimler Chrysler Fiat she served as the manager of Product and Process Integrity. In this position she supported interior and electrical product development (SMTs) areas in writing technical specifications, developing reliability requirements, constructing and executing designed experiments, developing FMEAs and Validation plans.
EDUCATION Mary has Bachelor of Arts (BA), Psychology and Human Factors from University of Delaware, Newark, DE. She also has a Master of Science (MS), Industrial Psychology and Applied Statistics from University of Akron, Akron, OH. Mary hold numerous certifications including: Certified Reliability Engineer CRE, Certified Manager of Quality and Organizational Excellence CQM/OE, Certified Quality Engineer CQE, Certified Quality Auditor CQA, Registrar Accreditation Board Quality Auditor.

- Engineering
The Partner of Choice for Engineers Across the Globe
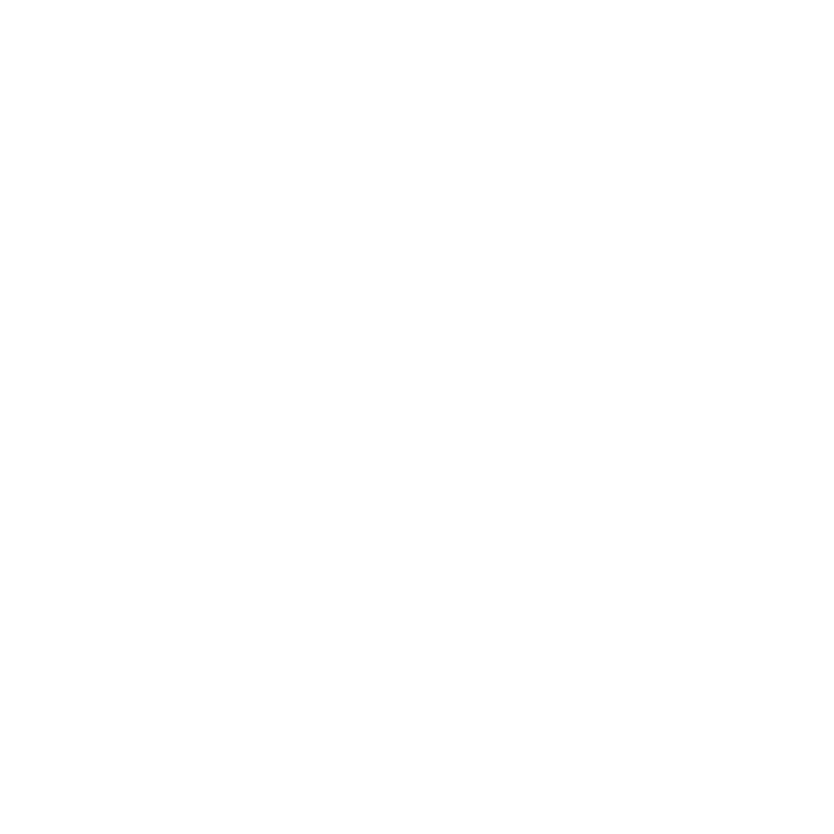
SOLUTION TOPICS
Quality engineering, process engineering, chemical engineering, biomedical engineering, electrical engineering, reliability engineering, mechanical engineering, solutions built for engineers.
Minitab provides a multitude of solutions for engineers to aid in problem solving and analytics. Attack problems with brainstorming tools, plan projects and process improvements through visual tools, and then collect data and analyze it, all within the Minitab ecosystem. Our solutions can help you find the answers you need using graphical tools, statistics, and predictive analytics.
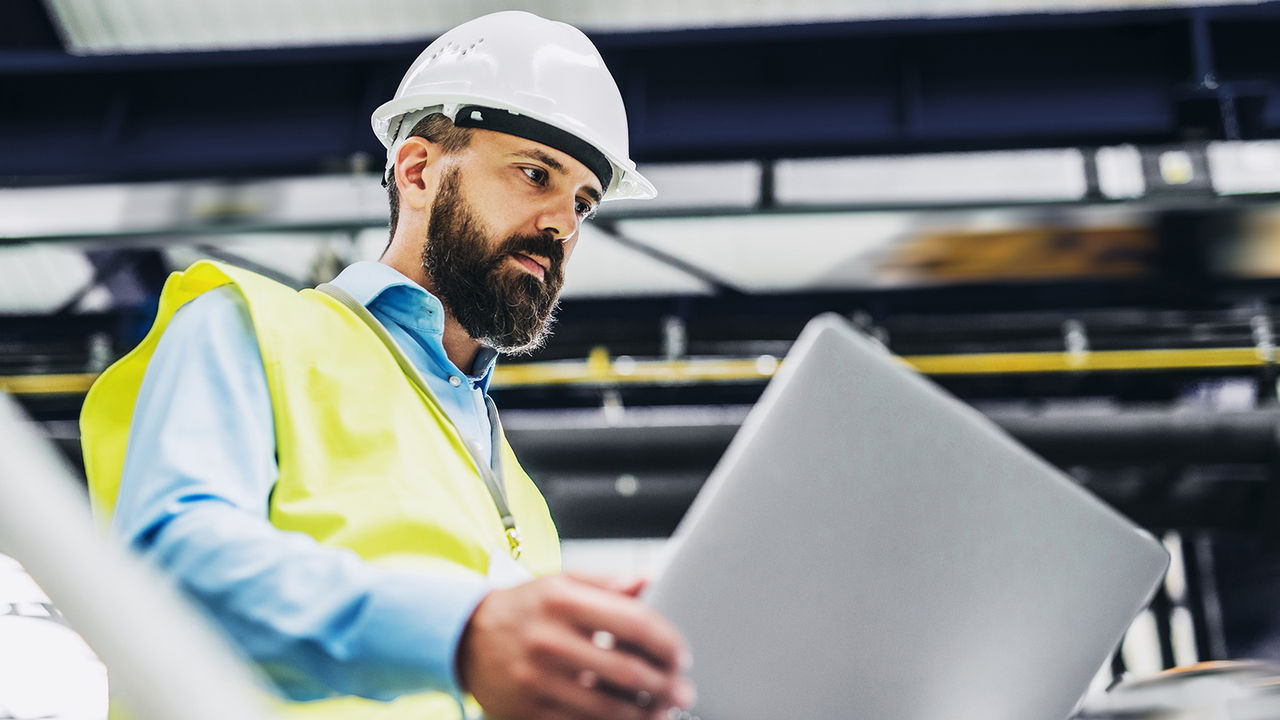
For quality professionals, it is crucial to quickly and clearly identify the root cause when customers are experiencing problems with services or products. Minitab is the ideal partner for quality professionals who want to take on additional projects, or lead the development of a formal continuous improvement or operational excellence program.
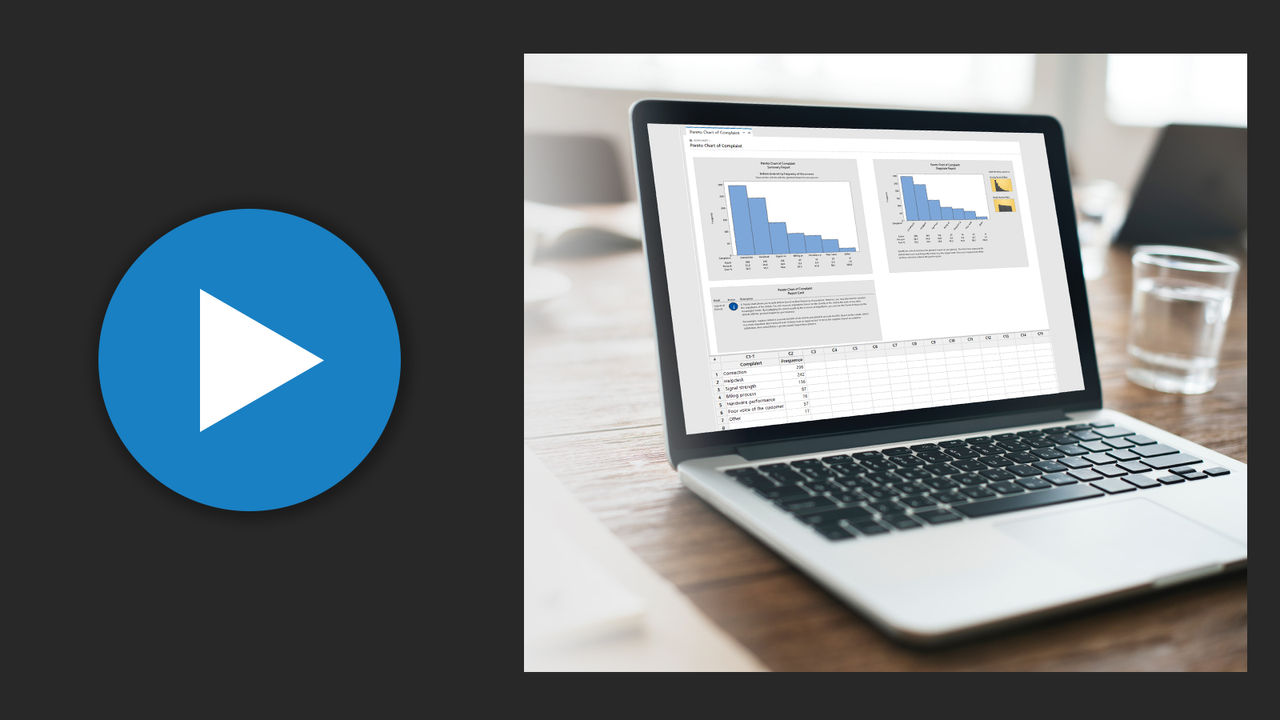
Watch the webinar to explore a step-by-step project roadmap to effectively investigate, improve, and maintain a process.
Process engineers need to understand their processes. Minitab is the expert in process improvement, with solutions designed to identify areas of improvement, measure processes outcomes, and monitor them. See an example of how Minitab can help with capability statistics that tell you how well your process is meeting the specifications that you have.
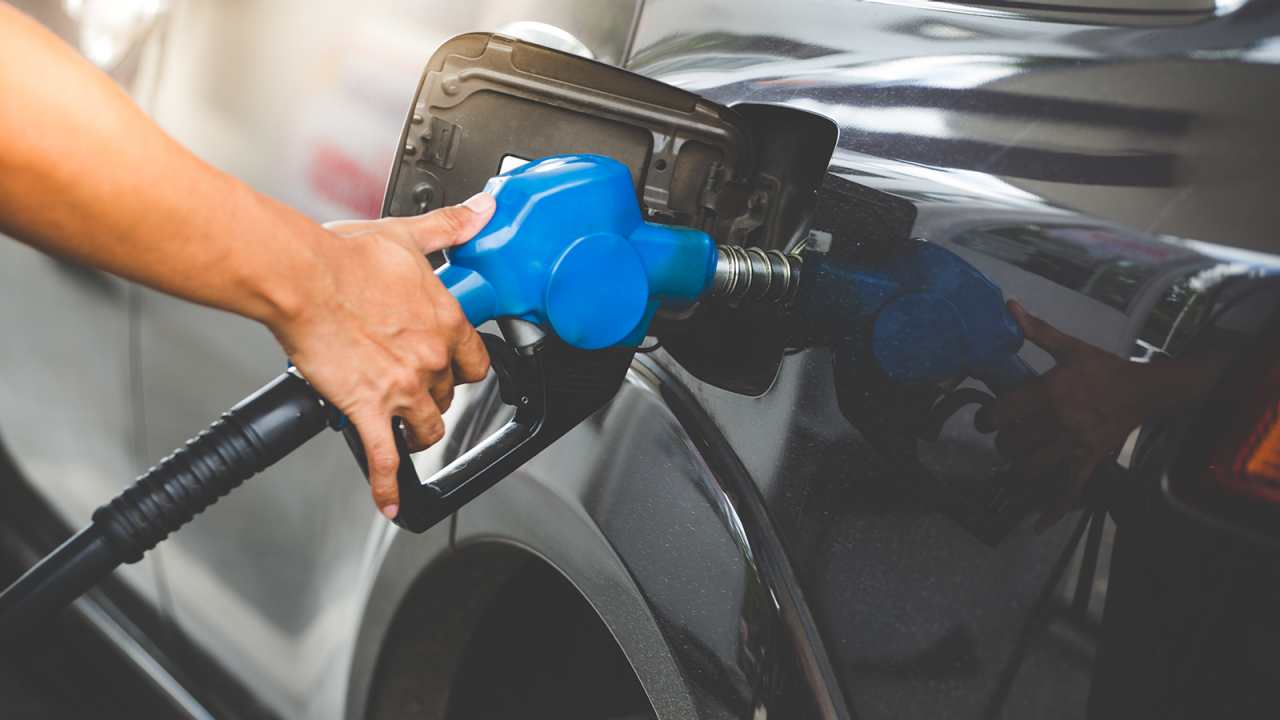
Statistics plays a big role in all engineering professions and has become even more important for chemical engineers. Because of the proliferation of inexpensive instrumentation, an engineer working in a modern plant has access to a tremendous amount of data. As a result, chemical engineers are dealing with more, and more complex, data than ever before. Learning data analysis and data science skills will enable you to provide unique and in-depth insights from your data that can create significant value for your organization today.
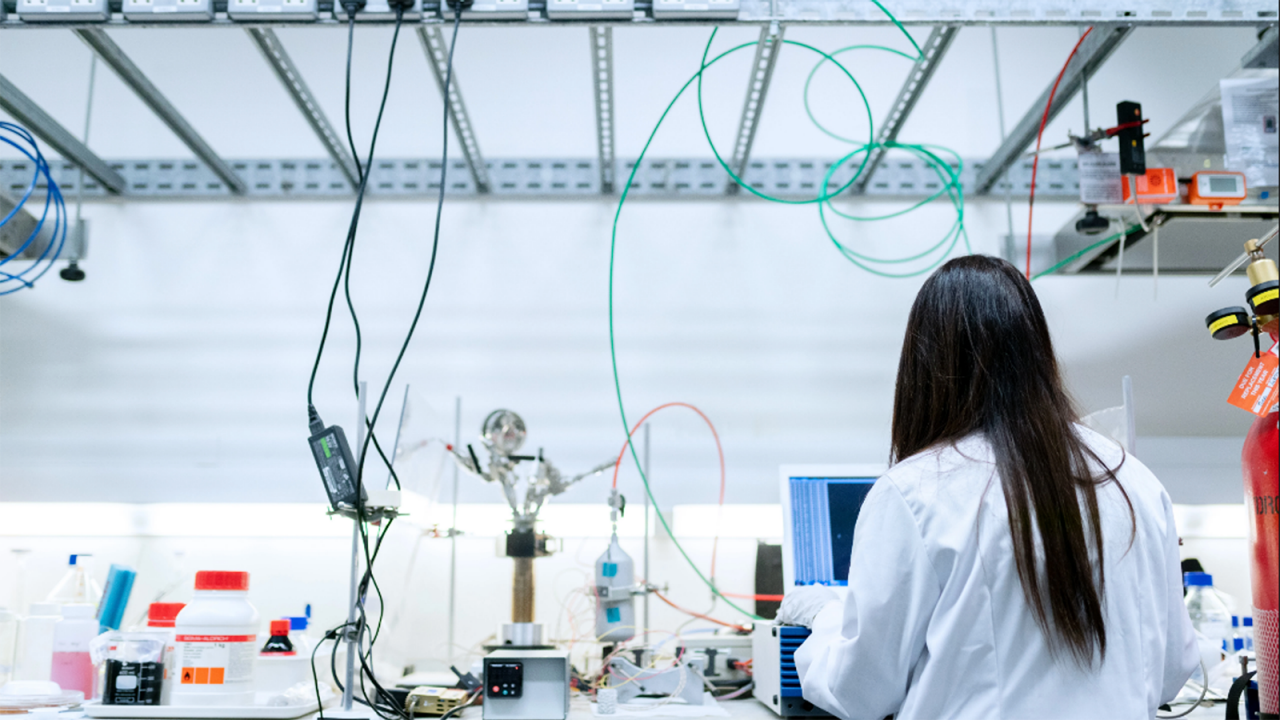
Applying the principles of biology, medicine, and engineering create life-saving solutions. That’s why Minitab works closely with biomedical engineers to ensure they are creating the safest and highest quality products. Learn how Boston Scientific leveraged Minitab solutions to improve manufacturing and as a result, drove significant savings.
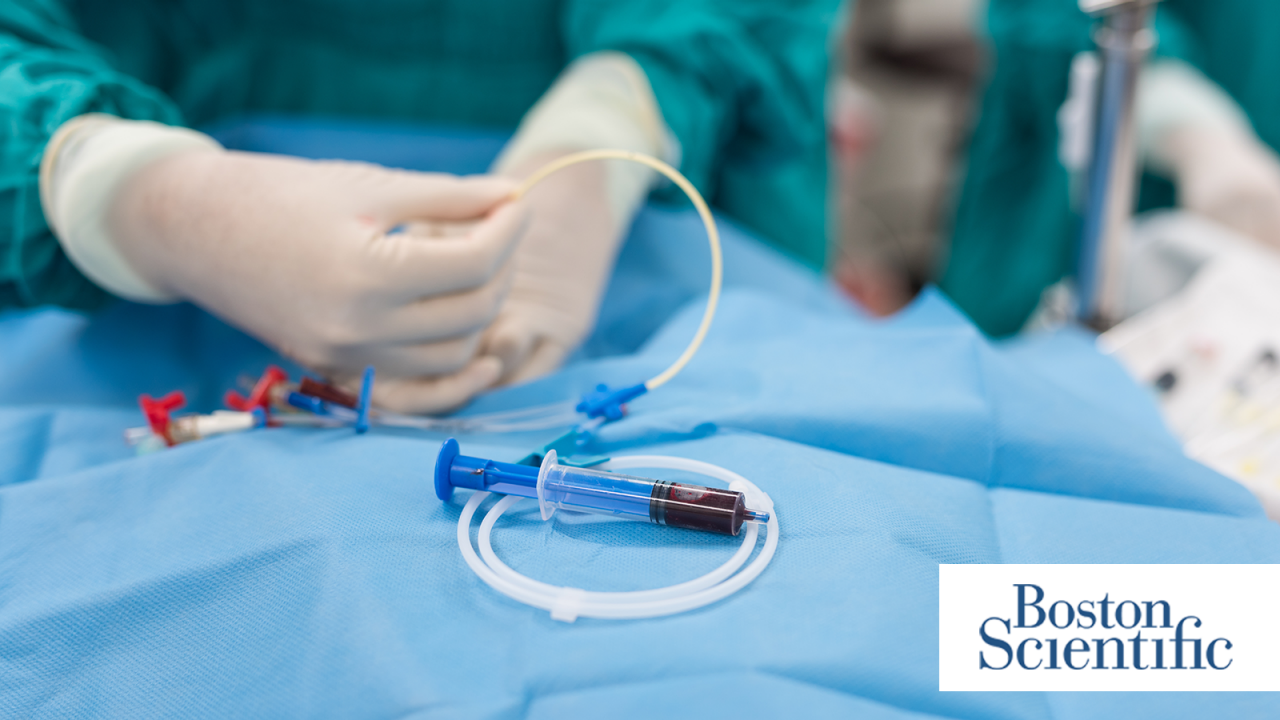
Minitab provides solutions to enable electrical engineers to design, develop, test, and supervise the manufacture of electrical equipment. With different analytical and problem-solving tools, Minitab is the partner of choice for electrical engineers. Learn more about how one electronics maker used one of Minitab’s tools to find better specifications for suppliers and realize significant cost savings.
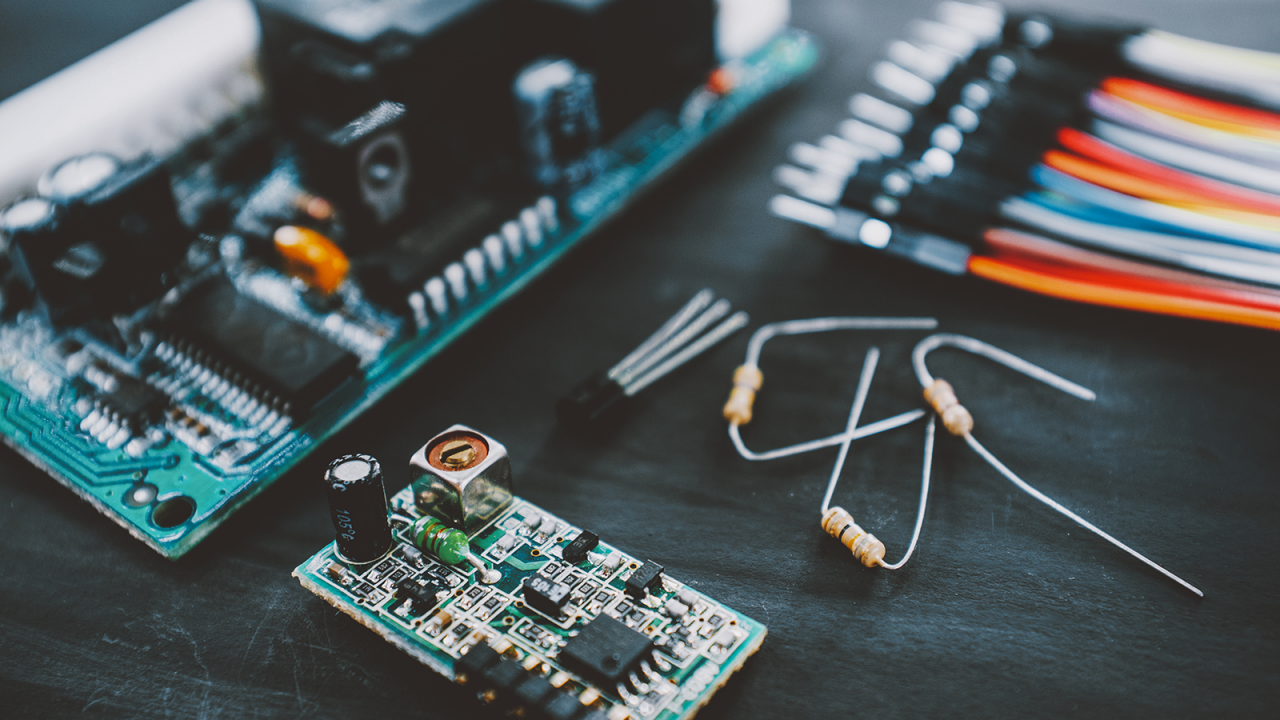
Imagine your new car breaks down after driving 60 miles. The engine light turns on and your vehicle must now be towed to be serviced. This is not only a warranty issue but also a field problem, due to lack of product reliability. Minitab partners with engineers to both optimize the design of products and ensure reliability to prevent this happening to you.
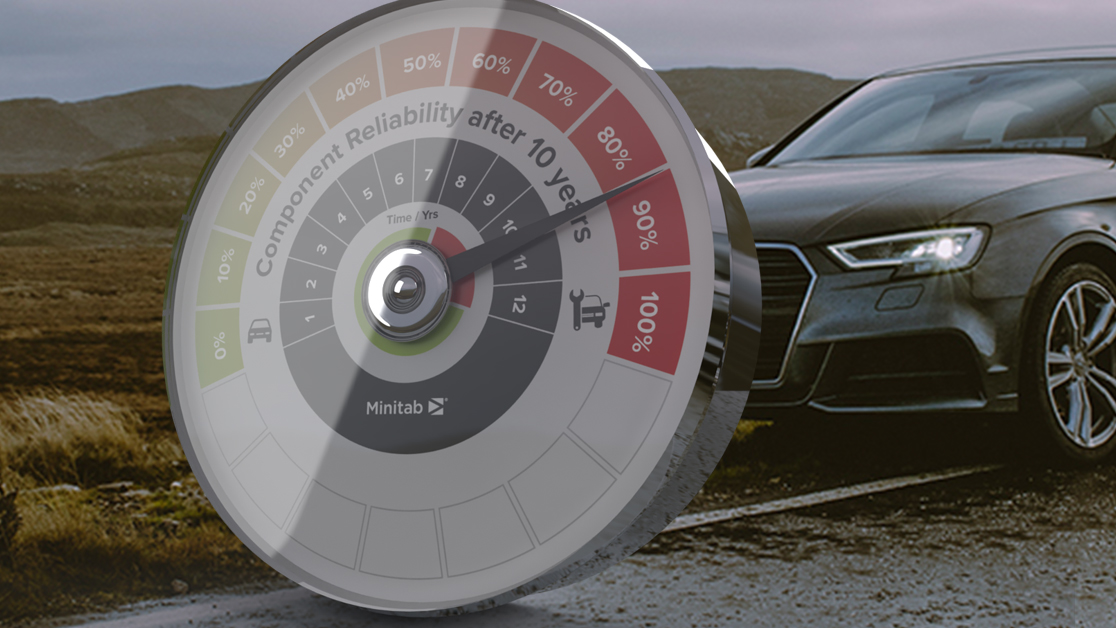
Mechanical engineers work on a wide range of projects from creating detailed designs to conducting simulations and analyses. Mechanical engineers must not only collaborate with multidisciplinary teams to ensure projects are completed successfully and on schedule, but they also play a key role in troubleshooting and resolving technical issues, conducting research, and improving mechanical systems and devices. Minitab provides mechanical engineers the broadest solutions and resources needed to perform their duties better, faster and easier.
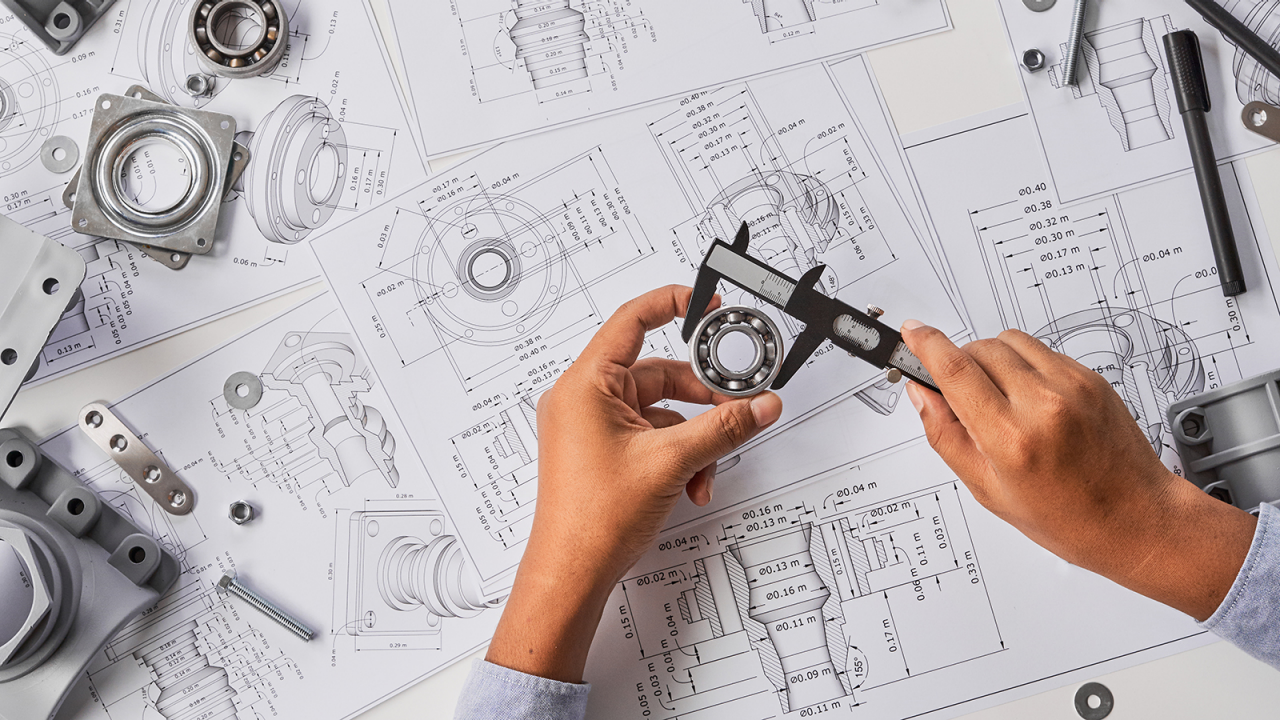
The Partner of Choice for Engineers Around the Globe
When there is a problem, engineers are tasked to solve it. As if that isn’t challenging enough, working without proper tools and solutions makes problem-solving more difficult. To truly be successful, engineers need access to data, solutions to integrate and analyze it, project management skills, and templates to improve the process and ensure continuous improvement.
That’s why Minitab created an ecosystem that tackles problem-solving the way engineers do. Need structured problem-solving tools to brainstorm? Check! Want to standardize forms like FMEAs, PPAP, or House of Quality? We have them! Looking to pull data from different sources to analyze on one platform? You’ve come to the right place!
Whether you’re an engineer in quality or process improvement, or in product development, we understand the day-to-day challenges of properly designing and testing. That’s because we’ve been doing it for over 50 years. We provide solutions to collect, monitor, and measure data with an eye toward improvement. Plus, with our services, training, and education, we can help you do your job better and even expand your skillset. We can help solve your problems, so that you can solve them for your organization.
OUR CUSTOMERS
“Minitab [is] the best tool for quality management. I use Minitab to run time series plots, charts, and control charts, as well as Pareto charts, fish bone charts…all the quality data was presented to upper management using Minitab. I would recommend it without hesitation!”
Jose Luis P. Quality Chief
“I deal with a few projects at a time and Minitab really helps me in understanding the failure points and relations within sub activities of a process. I can do a better root cause analysis to find the origin of a problem and also use the FMEA tool to weigh the severity, occurrence, and detection. I can tell with confidence that we have saved a whole lot of time compared to the hours of discussions and calculations we would have spent if we didn't invest in this software. The efficiency of our team has gone up significantly.”
Rahul V. Senior Development Engineer
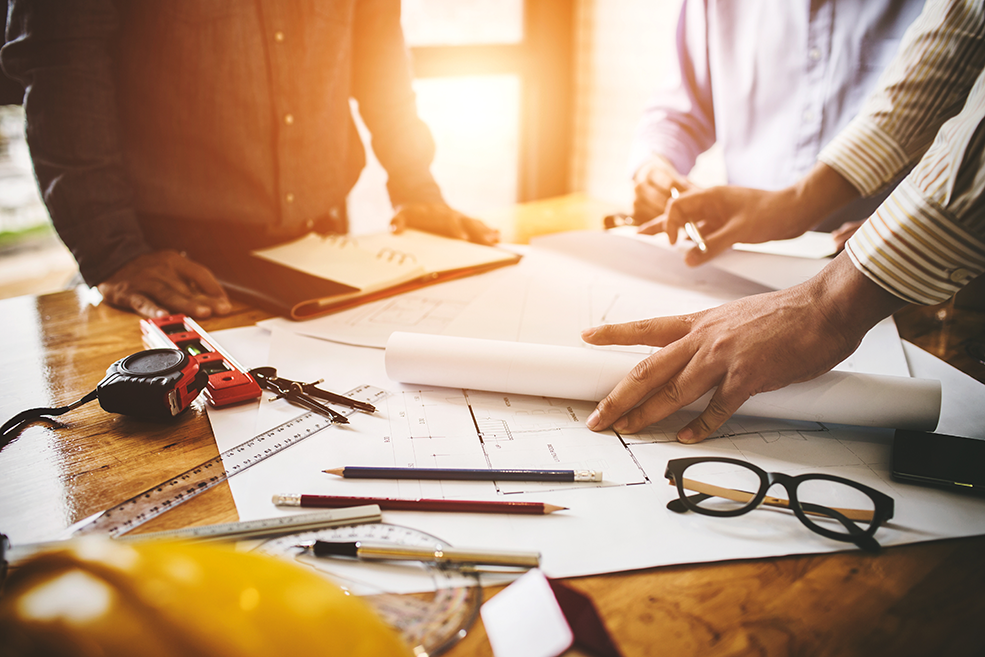
“Minitab has made it so easy to analyze [my] data... from creating graphs, statistical analysis, and forming reports, it is the best thing an engineer can ask for. You don't need to be an expert to use it.”
Maria M. Industrial & Manufacturing Engineer
- Trust Center
© 2024 Minitab, LLC. All Rights Reserved.
- Terms of Use
- Privacy Notice
- Cookie Settings
You are now leaving minitab.com.
Click Continue to proceed to:
Using Statistics to Improve Problem Solving Skills
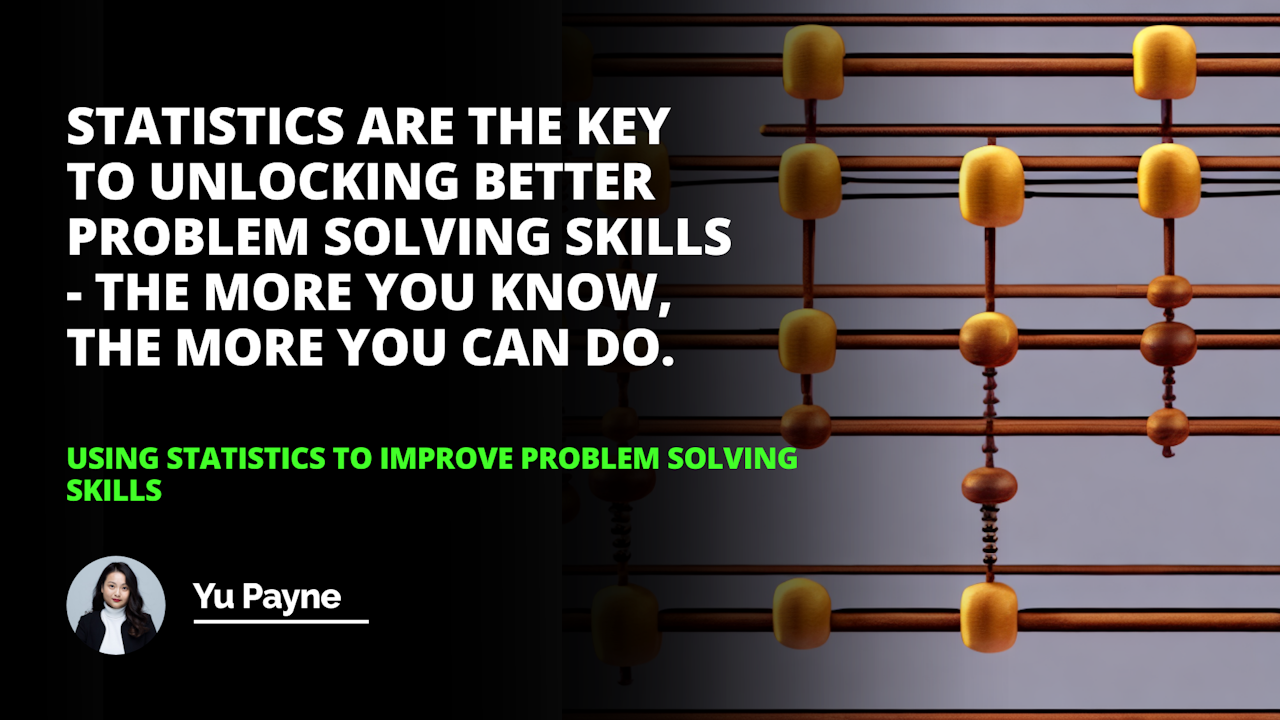
Problem-solving is an essential skill that everyone must possess, and statistics is a powerful tool that can be used to help solve problems. Statistics uses probability theory as its base and has a rich assortment of submethods, such as probability theory, correlation analysis, estimation theory, sampling theory, hypothesis testing, least squares fitting, chi-square testing, and specific distributions.
Each of these submethods has its unique set of advantages and disadvantages, so it is essential to understand the strengths and weaknesses of each method when attempting to solve a problem.
Introduction
Overview of Problem-Solving
Role of statistics in problem-solving, probability theory, correlation analysis.
Introduction: Problem-solving is a fundamental part of life and an essential skill everyone must possess. It is an integral part of the learning process and is used in various situations. When faced with a problem, it is essential to have the necessary tools and knowledge to identify and solve it. Statistics is one such tool that can be used to help solve problems.
Problem-solving is the process of identifying and finding solutions to a problem. It involves understanding the problem, analyzing the available information, and coming up with a practical and effective solution. Problem-solving is used in various fields, including business, engineering, science, and mathematics.
Statistics is a powerful tool that can be used to help solve problems. Statistics uses probability theory as its base, so when your problem can be stated as a probability, you can reliably go to statistics as an approach. Statistics, as a discipline, has a rich assortment of submethods, such as probability theory, correlation analysis, estimation theory, sampling theory, hypothesis testing, least squares fitting, chi-square testing, and specific distributions (e.g., Poisson, Binomial, etc.).
Probability theory is the mathematical study of chance. It is used to analyze the likelihood of an event occurring. Probability theory is used to determine the likelihood of an event, such as the probability of a coin landing heads up or a certain number being drawn in a lottery. Probability theory is used in various fields, including finance, economics, and engineering.
Correlation analysis is used to determine the relationship between two variables. It is used to identify the strength of the relationship between two variables, such as the correlation between the temperature and the amount of rainfall. Correlation analysis is used in various fields, including economics, finance, and psychology.
Estimation Theory
Estimation theory is used to estimate the value of a variable based on a set of data. It is used to estimate the value of a variable, such as a city's population, based on a sample of the population. Estimation theory is used in various fields, including economics, finance, and engineering.
Conclusion: Statistics is a powerful tool that can be used to help solve problems. Statistics uses probability theory as its base, so when your problem can be stated as a probability, you can reliably go to statistics as an approach. Statistics, as a discipline, has a rich assortment of submethods, such as probability theory, correlation analysis, estimation theory, sampling theory, hypothesis testing, least squares fitting, chi-square testing, and specific distributions (e.g., Poisson, Binomial, etc.). Each submethod has unique advantages and disadvantages, so it is essential to select the one that best suits your problem. With the right approach and tools, statistics can be a powerful tool in problem-solving.
Statistics are the key to unlocking better problem-solving skills - the more you know, the more you can do. IIENSTITU
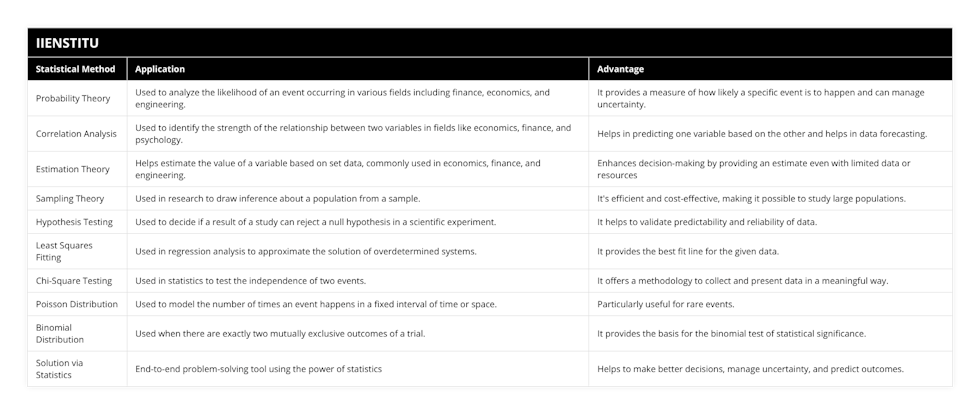
What role does probability theory play in using statistics to improve problem solving skills?
Probability theory and statistics are both essential tools for problem-solving, and the two disciplines share an interdependent solid relationship. This article will discuss the role that probability theory plays in using statistics to improve problem-solving skills.
Probability theory provides a framework for understanding the behavior of random variables and their associated distributions. We can use statistics to make better predictions and decisions by understanding and applying probability theory. For example, when calculating the probability of a desired outcome, we can use statistical methods to determine the likelihood of that outcome occurring. This can be used to inform decisions and help us optimize our strategies.
Statistics also provide us with powerful tools for understanding the relationship between variables. By analyzing the correlation between two or more variables, we can gain valuable insights into the underlying causes and effects of a problem. For example, by studying a correlation between two variables, we can determine which variable is more likely to cause a particular outcome. This can help us to design more effective solutions to problems.
By combining probability theory and statistics, we can develop powerful strategies for problem-solving. Probability theory helps us understand a problem's underlying structure, while statistics provide us with the tools to analyze the data and make better predictions. By understanding how to use these two disciplines together, we can develop more effective solutions to difficult problems.
In conclusion, probability theory and statistics are both essential for problem-solving. Probability theory provides a framework for understanding the behavior of random variables, while statistics provide powerful tools for understanding the relationships between variables. By combining the two disciplines, we can develop more effective strategies for solving complex problems.
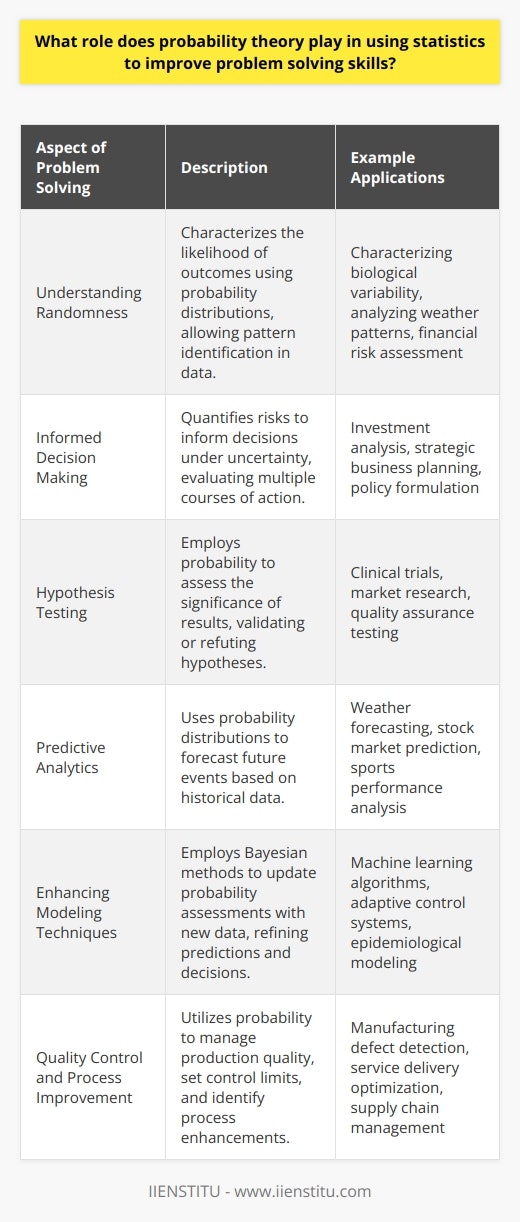
How can correlation analysis be used to identify relationships between variables when solving problems?
Correlation analysis is a powerful tool for identifying relationships between variables when solving problems. It is a statistical approach that measures how two variables are related. By analyzing the correlation between two variables, researchers can identify the strength and direction of their relationship. For example, a correlation analysis can determine if a change in one variable is associated with a change in the other.
When conducting correlation analysis, researchers often use Pearson’s correlation coefficient (r) to measure the strength of the association between two variables. This coefficient ranges from -1 to +1, where -1 indicates a perfect negative correlation, 0 indicates no correlation, and +1 indicates a perfect positive correlation. A perfect positive correlation indicates that when one variable increases, the other variable also increases, and a perfect negative correlation indicates that when one variable increases, the other variable decreases.
Correlation analysis helps identify relationships between variables when solving problems. For example, in a study of the relationship between dietary habits and body weight, a researcher may use correlation analysis to determine if there is a relationship between the two variables. Suppose the researcher finds a significant correlation between dietary habits and body weight. In that case, this can provide insight into the studied problem and help inform solutions.
Correlation analysis can also be used to identify causal relationships between variables. By examining the relationship between two variables over time, researchers can determine if a change in one variable is associated with a change in the other. For example, a researcher may use correlation analysis to determine if temperature changes are associated with changes in air quality. If a significant correlation is found, then the researcher can conclude that temperature changes are likely causing changes in air quality.
Overall, correlation analysis is a powerful tool for identifying relationships between variables when solving problems. By examining the strength and direction of the relationship between two variables, researchers can gain insight into the problem being studied and inform potential solutions.
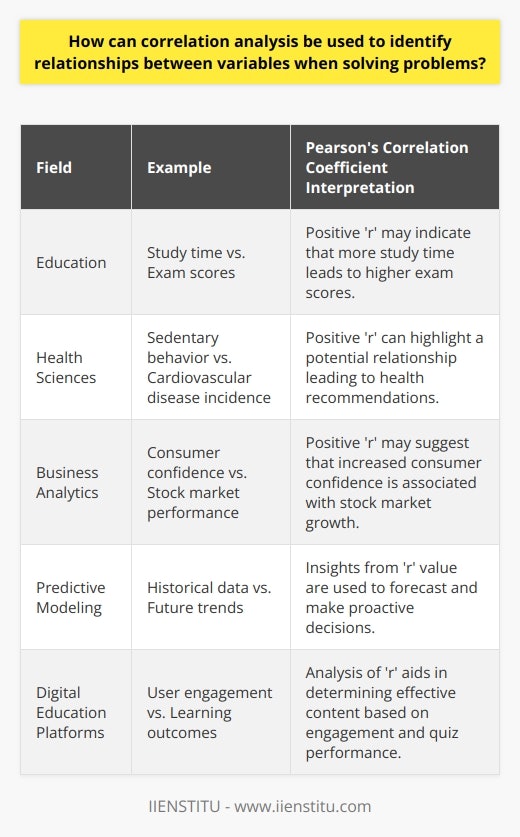
What are the benefits of using estimation theory when attempting to solve complex problems?
Estimation theory is a powerful tool when attempting to solve complex problems. This theory involves making educated guesses or estimations about the value of a quantity that is difficult or impossible to measure directly. By utilizing estimation theory, one can reduce uncertainty and make decisions more confidently.
The main benefit of using estimation theory is that it allows for the quantification of uncertainty. By estimating, one can determine the range of possible outcomes and make decisions based on the likelihood of each outcome. This helps to reduce the risks associated with making decisions as it allows one to make better decisions based on the available data.
Another benefit of using estimation theory is that it can be applied to many problems. Estimation theory can be used to solve problems in fields such as engineering, finance, and economics. It can also be used to estimate a stock's value, the project's cost, or the probability of a certain event. Estimation theory is also useful in predicting the behavior of a system over time.
Estimation theory can also be used to make decisions in cases where the data is limited. By estimating, one can reduce the amount of data needed to make a decision and make more informed decisions. Furthermore, estimation theory can be used to make decisions even when the data is incomplete or inaccurate. This is especially useful when making decisions in situations where the data is uncertain or incomplete.
In conclusion, estimation theory is a powerful tool for solving complex problems. It can be used to reduce uncertainty, make decisions in cases where data is limited or incomplete, and make predictions about the behavior of a system over time. By utilizing estimation theory, one can make more informed decisions and reduce the risks associated.
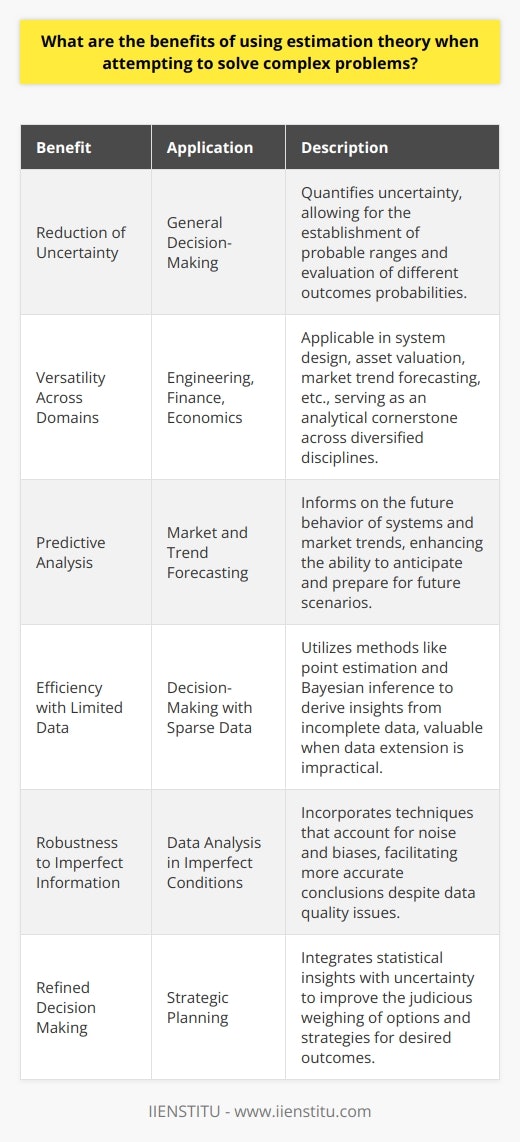
How does the application of statistical methods contribute to effective problem-solving in various fields?
**Statistical Methods in Problem-solving** Statistical methods play a crucial role in effective problem-solving across various fields, including natural and social sciences, economics, and engineering. One primary contribution lies in the quantification and analysis of data. **Data Quantification and Analysis** Through descriptive statistics, researchers can summarize, organize, and simplify large data sets, enabling them to extract essential features and identify patterns. In turn, this fosters a deeper understanding of complex issues and aids in data-driven decision-making. **Prediction and Forecasting** Statistical methods can help predict future trends and potential outcomes with a certain level of confidence by extrapolating obtained data. Such prediction models are invaluable in fields as diverse as finance, healthcare, and environmental science, enabling key stakeholders to take proactive measures. **Hypothesis Testing** In the scientific process, hypothesis testing enables practitioners to make inferences about populations based on sample data. By adopting rigorous statistical methods, researchers can determine the likelihood of observed results occurring randomly or due to a specific relationship, thus validating or refuting hypotheses. **Quality Control and Improvement** In industries and manufacturing, statistical methods are applied in quality control measures to ensure that products and services meet established standards consistently. By identifying variations, trends, and deficiencies within production processes, statistical techniques guide improvement efforts. **Design of Experiments** Statistical methods are vital in the design of experiments, ensuring that the collected data is representative, reliable, and unbiased. By utilizing techniques such as random sampling and random assignment, researchers can mitigate confounding variables, increase generalizability, and establish causal relationships. In conclusion, the application of statistical methods contributes to effective problem-solving across various fields by enabling data quantification, analysis, and prediction. Additionally, these methods facilitate hypothesis testing, quality control, and the design of experiments, fostering confidence in decision-making and enhancing outcomes.
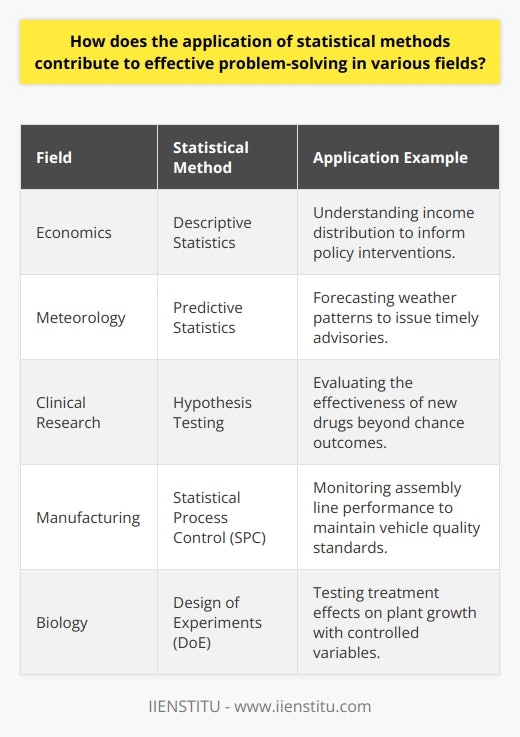
In what ways can statistical analysis enhance the decision-making process when facing complex challenges?
Statistical analysis in decision-making Statistical analysis plays a crucial role in the decision-making process when facing complex challenges by enabling evidence-based decisions. It provides a systematic approach to accurately interpret data and transform it into meaningful and actionable insights. In turn, these insights enhance decision-making by reducing uncertainty, minimizing risks, and increasing confidence in the chosen strategy. Quantitative approach By adopting a quantitative approach, decision-makers can objectively evaluate various options using statistical techniques, such as regression analysis or hypothesis testing. This process facilitates the identification of patterns and relationships within the data, highlighting crucial factors that can significantly impact desired outcomes. Consequently, leaders can make informed decisions that optimize available resources and maximize benefits, ultimately increasing the overall success rate of implemented strategies. Addressing biases Statistical analysis helps to address cognitive biases that may otherwise cloud judgment and impede the decision-making process. These biases could include confirmation bias, anchoring bias, and availability heuristic, among others. Employing quantitative methods illuminates the influence these biases may have on subjective interpretations and assists decision-makers in mitigating potential negative impacts. Risk analysis In the context of complex challenges, risk analysis plays an essential role in decision-making. By employing statistical models, decision-makers can quantify risk, estimate probabilities of potential outcomes, and determine the optimal balance between risk and reward. This information can be invaluable for organizations when allocating resources, prioritizing projects, and managing uncertainty in dynamic environments. Data-driven forecasts Statistical analysis enables decision-makers to create accurate forecasts by extrapolating historical data and incorporating current trends. These forecasts can inform strategic planning, budget allocations, and resource management, reducing the likelihood of unforeseen obstacles and ensuring long-term success. In addition to providing a strong basis for future planning, these data-driven predictions also enable organizations to quickly adapt and respond to emerging trends and challenges. In conclusion, statistical analysis is an invaluable tool for enhancing the decision-making process when facing complex challenges. By adopting a quantitative approach, addressing cognitive biases, conducting risk analysis, and producing data-driven forecasts, decision-makers can make informed choices that optimize outcomes and minimize potential risks.
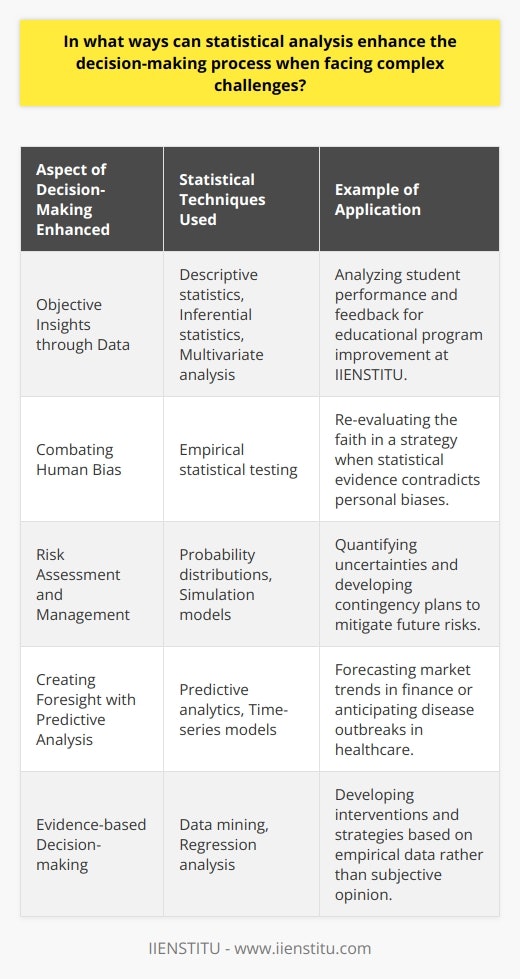
How can concepts like statistical hypothesis testing and regression analysis be applied to solve real-world problems and make informed decisions?
Applications of Hypothesis Testing Statistical hypothesis testing can be a vital tool in decision-making processes, particularly when it comes to addressing real-world problems. In business, for example, managers may use hypothesis testing to determine whether a new product or strategy will lead to higher revenues or customer satisfaction. This can then inform their decisions on whether to invest in the product or strategy or explore other options. In medicine, researchers can use hypothesis testing to compare the effectiveness of a new treatment or intervention compared to standard care, which can provide valuable evidence to guide clinical practice. Regression Analysis to Guide Decisions Similarly, regression analysis is a powerful statistical technique used to understand relationships between variables and predict future outcomes. By modeling the connections between different factors, businesses can make data-driven decisions and develop strategies based on relationships found in historical data. For instance, companies can use regression analysis to forecast future sales, evaluate the return on investment for marketing campaigns, or identify factors that contribute to customer churn. In fields like public health, policymakers can use regression analysis to identify the effects of various interventions on health outcomes, leading to more effective resource allocation and targeting of mass media campaigns. Assessing Real-World Solutions The implementation of statistical hypothesis testing and regression analysis enables stakeholders across diverse disciplines to evaluate and prioritize potential solutions to complex problems. By identifying significant relationships between variables and outcomes, practitioners can develop evidence-based approaches to improve decision-making processes. These methods can be applied to problems in various fields, such as healthcare, public policy, economics, and environmental management, ultimately providing benefits for both individuals and society. Ensuring Informed Decisions In conclusion, both statistical hypothesis testing and regression analysis have a vital role in solving real-world problems and informing decisions. These techniques provide decision-makers with the necessary evidence to evaluate different options, strategies, or interventions to make the most appropriate choices. By incorporating these statistical methods into the decision-making process, stakeholders can increase confidence in their conclusions and improve the overall effectiveness of their actions, leading to better outcomes in various fields.
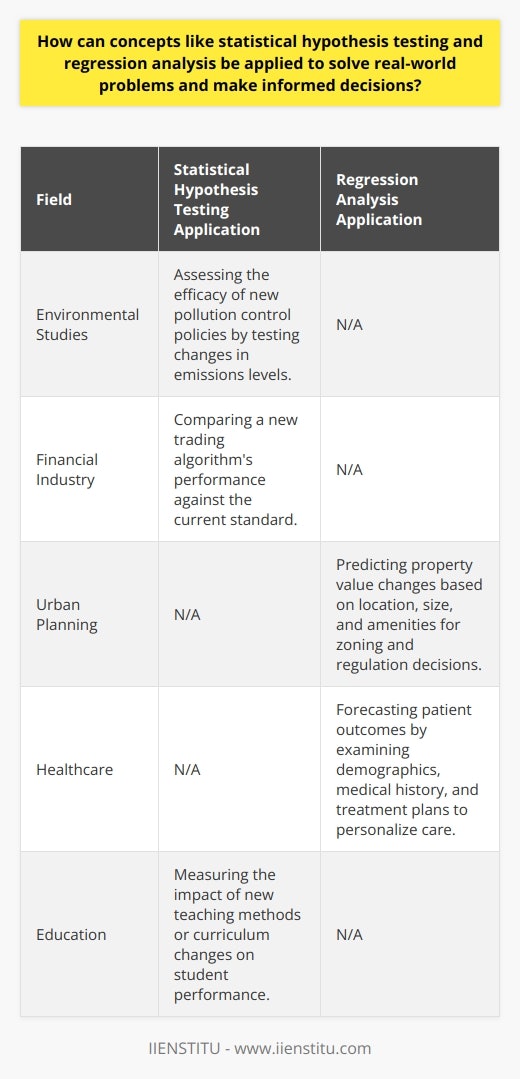
How does the use of descriptive and inferential statistics improve our understanding of complex problems and inform decision-making?
The Importance of Descriptive and Inferential Statistics in Problem Solving Descriptive statistics provide essential context Descriptive statistics summarize, organize, and simplify data, offering a comprehensive snapshot of a data set. By presenting data in a meaningful and easily interpretable manner, descriptive statistics enable researchers to understand and describe the key characteristics of a data set. This initial step in any data analysis is crucial for establishing context, identifying patterns, and generating hypotheses that contribute to a better understanding of complex problems. Inferential statistics as a tool for decision-making Inferential statistics, on the other hand, involve drawing conclusions and making generalizations about a larger population based on the analysis of a sample. Through hypothesis testing, confidence intervals, and regression analysis, researchers can determine relationships among variables, identify trends, and predict outcomes. By offering insights that go beyond the data at hand, inferential statistics enable researchers to make informed decisions and create strategies for tackling complex problems. The synergy of descriptive and inferential statistics In combination, both descriptive and inferential statistics enhance the understanding and decision-making process in various fields. Descriptive statistics provide a solid foundation by organizing and summarizing data, while inferential statistics enable researchers to delve deeper, uncovering relationships and trends that facilitate evidence-based decision-making. This combination empowers researchers to identify solutions and make more informed decisions when tackling complex problems.
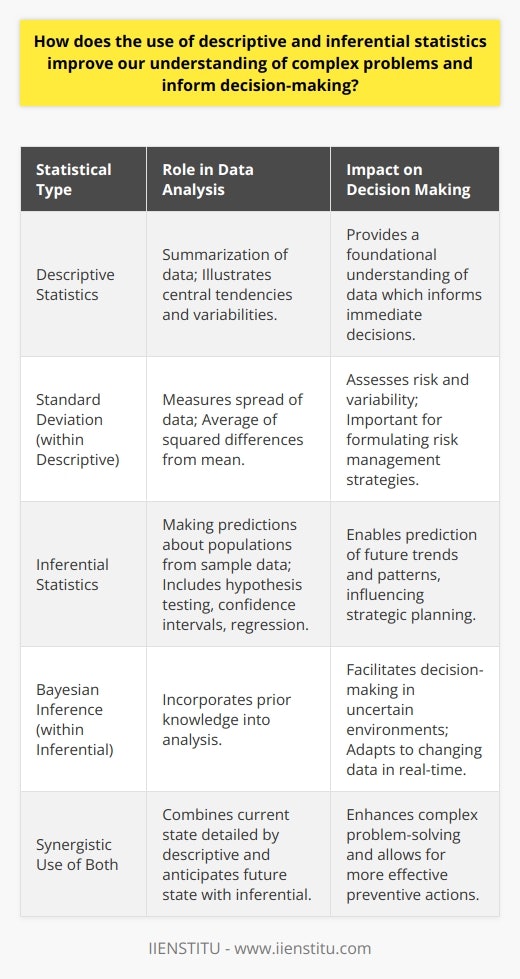
What is the role of experimental design and sampling techniques in ensuring reliable and accurate conclusions when utilizing statistical analysis for problem-solving?
Role of Experimental Design Experimental design plays a pivotal role in ensuring reliable and accurate conclusions in statistical analysis when solving problems. A well-defined experimental design outlines a systematic approach to conducting research, including the selection of participants, allocation of resources, and timing of interventions. It helps control potential confounding factors and biases, allowing researchers to attribute the study results to the intended interventions accurately. Moreover, experimental design enables researchers to quantify uncertainty in their findings through hypothesis testing, thereby establishing the statistical significance of their conclusions. Sampling Techniques Sampling techniques are another essential component in achieving valid and reliable results in statistical analysis. They ensure that the data collected from a population is representative of the whole, thus allowing for accurate generalizations. Proper sampling techniques, such as random sampling or stratified sampling, minimize the prevalence of sampling bias, which may otherwise lead to false or skewed conclusions. Additionally, determining the appropriate sample size—large enough to maintain statistical accuracy and minimize type I and type II errors—is crucial in enhancing the reliability and precision of study results. Achieving Accurate Conclusions To draw accurate conclusions in statistical analysis, researchers must ensure that their experimental design and sampling techniques are carefully planned and executed. This involves selecting the most appropriate methods in accordance with study goals and population demographics. Furthermore, vigilance regarding potential confounders and biases, and continuous monitoring of data quality, contribute to the validity and reliability of statistical findings for problem-solving. Overall, a skillful combination of experimental design and sampling techniques is imperative for researchers to derive reliable and accurate conclusions from statistical analysis. By addressing potential pitfalls and adhering to best practices, this potent mix of methodologies allows for efficient problem-solving and robust insights into diverse research questions.
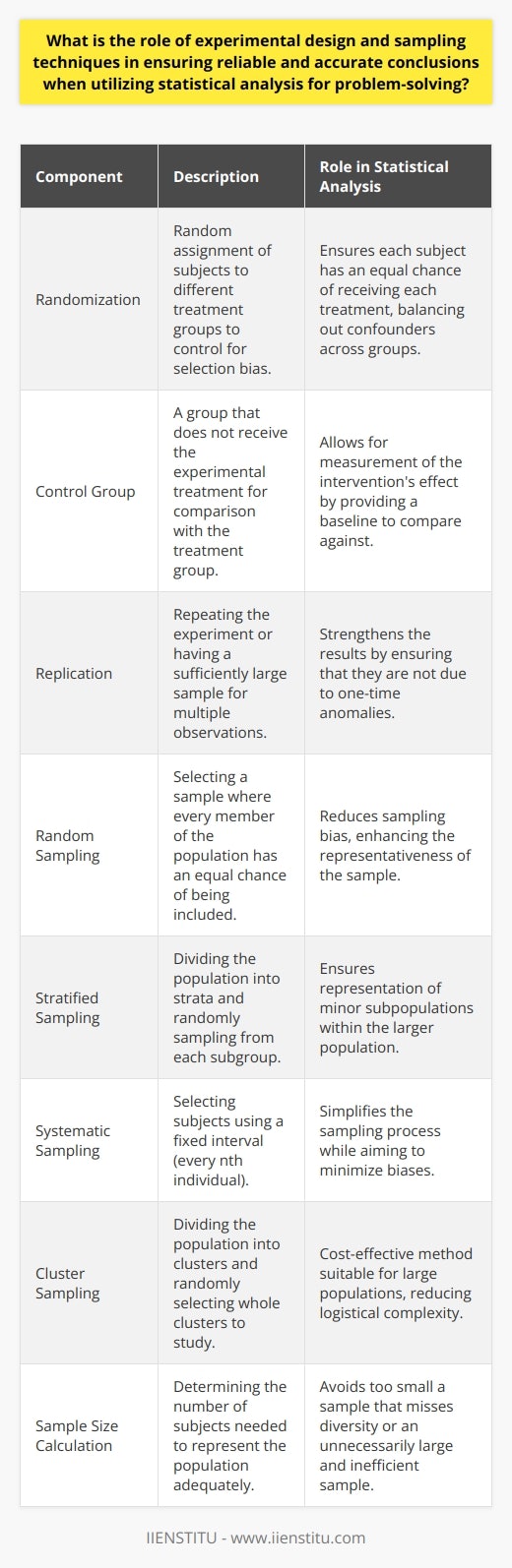
How do visualization techniques and exploratory data analysis contribute to a more effective interpretation of statistical findings in the context of real-world issues?
Enhancing Interpretation through Visualization Techniques Visualization techniques play a significant role in interpreting statistical findings related to real-world issues. By converting complex data into visually appealing and easy-to-understand formats, these techniques allow decision-makers to quickly grasp the underlying patterns and trends. Graphs, plots, and charts are some common visualization tools that make data more accessible, aiding in the identification of outliers and hidden relationships among variables. Exploratory Data Analysis: A Key Step Exploratory data analysis (EDA) is critical for effective interpretation of statistical findings. This approach involves an initial assessment of the data's characteristics, emphasizing summarizing and visualizing key aspects. Employing EDA allows researchers to identify errors, missing values, and inconsistencies in the data, which is instrumental when addressing real-world issues. By obtaining insights into the dataset's structure and potential biases, analysts can formulate appropriate statistical models and ensure more accurate predictions and inferences. Complementarity for Improved Data Interpretation Combining visualization techniques and EDA contributes to a more effective interpretation of statistical findings by offering a complementary approach. Visualization supports the exploration of data, enabling pattern and relationship identification, while EDA provides a deeper insight into data quality and potential limitations. Together, these methods facilitate a comprehensive understanding of the data, allowing for a more informed decision-making process when addressing real-world issues. In conclusion, visualization techniques and exploratory data analysis are essential tools for effectively interpreting statistical findings. By offering complementary benefits, they enhance decision-making processes and increase the likelihood of informed choices when examining real-world issues. As our world continues to produce vast amounts of data, these methods will remain critical to ensuring that statistical findings are accurate, relevant, and useful in solving pressing problems.
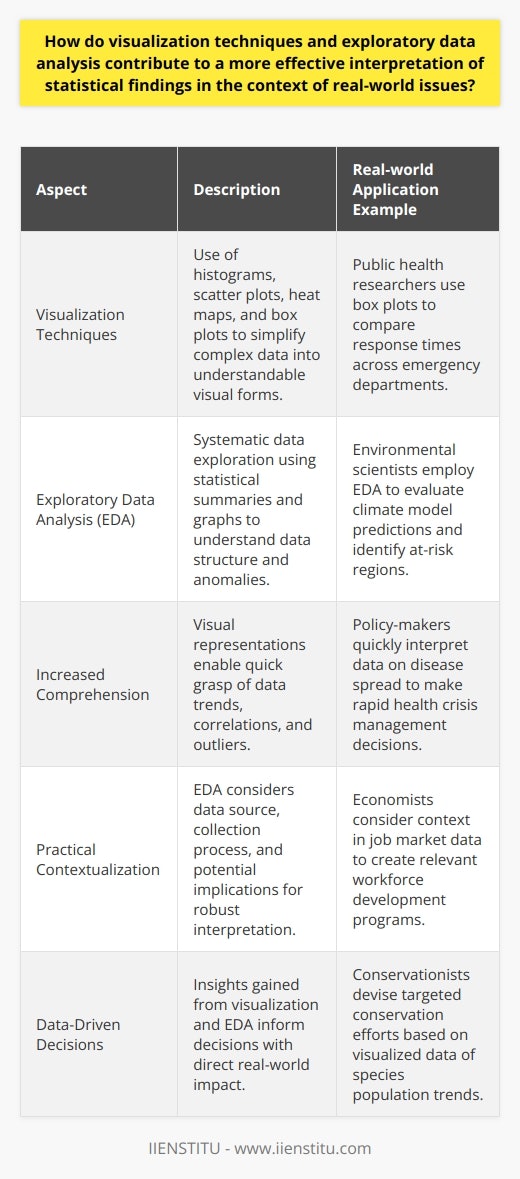
How does statistics help in problem-solving?
Role of Statistics in Problem-solving Understanding the Problem Statistics play a significant role in problem-solving by providing accurate data and quantitative evidence to better understand complex issues. The collection, analysis, and interpretation of numerical data enable decision-makers to observe trends, patterns, and relationships within the data, thus facilitating informed decision-making. To effectively solve problems, it is crucial to have a thorough understanding of the issue at hand, and statistics provide the necessary tools to explore and interpret the relevant data. Identifying Patterns and Trends Statistics help in identifying underlying patterns and trends within a dataset, which aids in understanding the problem's nature and behavior. By employing graphical and numerical techniques, statisticians can visualize relationships, fluctuations, and distributions within the data. Identifying these patterns can lead to the generation of hypotheses, proposing possible solutions, and implementing interventions to address the issues. Evaluating Solutions Once potential solutions are identified, statistics can be used to objectively evaluate their effectiveness by comparing the outcomes of different scenarios or interventions. Experimental designs such as controlled trials, surveys, and longitudinal studies are powerful tools for assessing the impact of problem-solving strategies. Furthermore, statistical significance testing allows decision-makers to determine the likelihood of results occurring by chance, providing more confidence in the selected solutions. Making Informed Decisions Through the use of statistical methods, decision-makers can be guided towards making more informed, evidence-based choices when solving problems. By basing decisions on empirical data, rather than relying on anecdotal evidence, intuition, or assumptions, organizations and policymakers can significantly improve the likelihood of producing successful outcomes. Statistical analysis enables the ranking of possible solutions according to their efficacy, which is crucial for resource allocation and prioritization within any setting. In conclusion, statistics play a crucial role in problem-solving by providing a systematic and rigorous approach to understanding complex issues, identifying patterns and trends, evaluating potential solutions, and guiding informed decision-making. The use of quantitative data and statistical methods allows for greater objectivity, accuracy, and confidence in the process of solving problems and ultimately leads to more effective and efficient solutions.
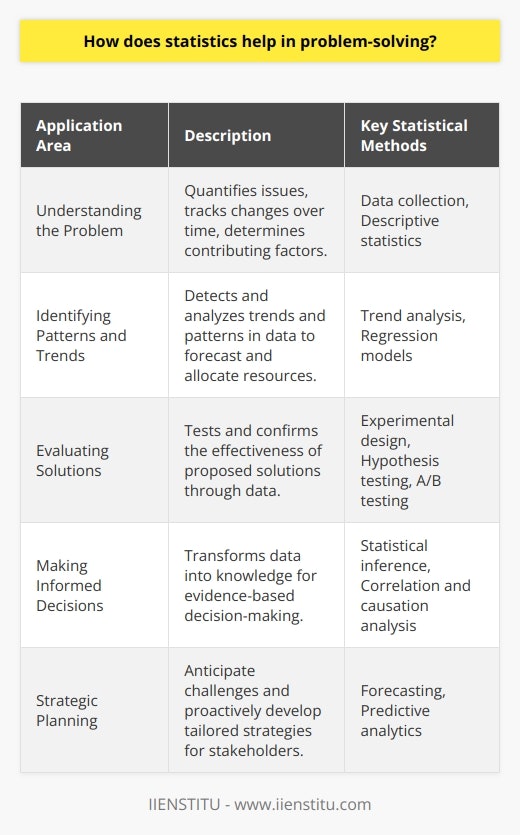
What are the five statistical processes in solving a problem?
Statistical Processes Overview The process of solving a problem using statistical methods involves five key steps. These steps enable researchers to analyze data and make inferences based on the results. 1. Defining the Problem The first step in any statistical problem-solving process is to clearly define the problem. This involves identifying the research question, objective, or hypothesis that needs to be tested. The problem should be specific and clearly stated to guide the subsequent steps in the process. 2. Data Collection Once the problem is defined, the next step is to collect data that will be used for analysis. Data can be collected through various methods, such as surveys, experiments, or secondary sources. The choice of data collection method should be based on the nature of the problem and the type of data required. It is important to collect data accurately and consistently to ensure the validity of the analysis. 3. Data Organization and Summarization After collecting the data, it needs to be organized and summarized in a way that makes it easy to analyze. This may involve using tables, graphs, or charts to display the data. Descriptive statistics, such as measures of central tendency (mean, median, mode) and measures of dispersion (range, variance, standard deviation), can be used to summarize the data. 4. Analysis and Interpretation At this stage, the data is analyzed using various statistical techniques to answer the research question or test the hypothesis. Inferential statistics, such as correlation analysis or hypothesis testing, can be employed to make inferences about the underlying population based on the sample data. It is crucial to choose the appropriate statistical method for the analysis, keeping in mind the research question and the nature of the data. 5. Drawing Conclusions and Recommendations The final step in the statistical process is to draw conclusions from the analysis and provide recommendations based on the findings. This involves interpreting the results of the analysis in the context of the research question and making generalizations or predictions about the population. The conclusions and recommendations should be communicated effectively, ensuring that they are relevant and useful for decision-making or further research. In conclusion, the five statistical processes in solving a problem are defining the problem, data collection, data organization and summarization, analysis and interpretation, and drawing conclusions and recommendations. These steps allow researchers to effectively analyze data and make informed decisions and predictions based on the results.
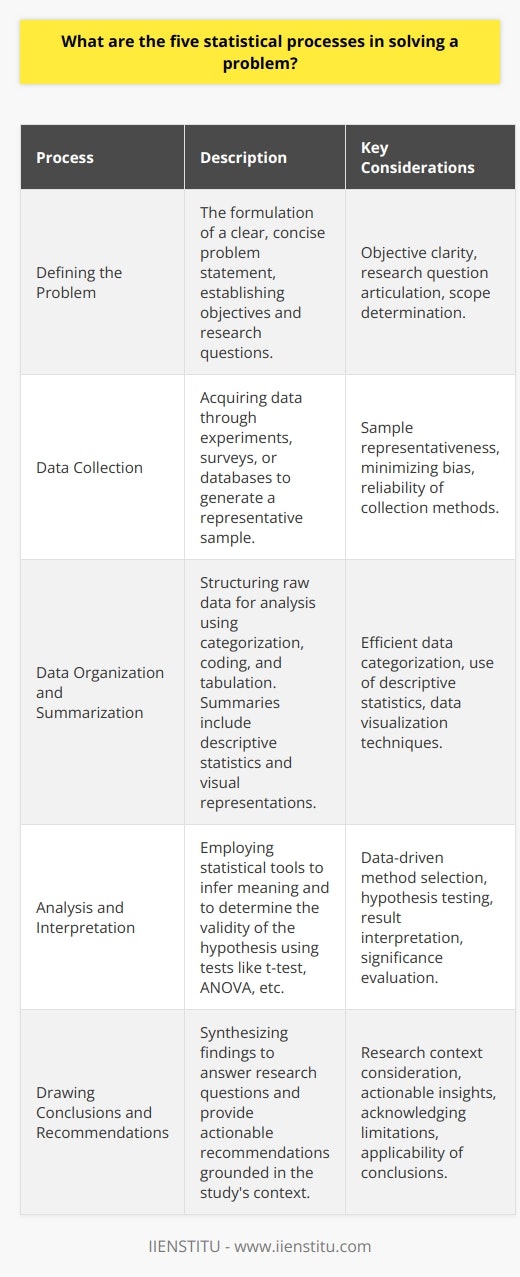
How can you use statistics effectively to resolve problems in everyday life?
Understanding the Basics of Statistics Statistics provides a systematic method for individuals to collect, analyze and interpret data. Through this approach, one can efficiently utilize these results to tackle issues they may encounter daily. In the ensuing discussion, we will delve into the process of incorporating statistics to address these everyday concerns effectively. Identifying the Problem Firstly, it is essential to accurately outline the issue at hand. This preliminary stage entails formulating definitive questions, which will guide the data gathering process. Such specificity ensures the assembled information directly pertains to the focal problem and eliminates the possibility of superfluous distractions. Collecting Relevant Data Next, amassing reliable and diverse information allows for well-informed interpretations. To successfully achieve this, it is crucial to identify suitable sources that offer the pertinent data required for a comprehensive analysis. Moreover, obtaining data from diverse sources helps mitigate the potential for biased or skewed outcomes. Implementing Appropriate Statistical Techniques Upon compiling a robust dataset, the implementation of applicable statistical methods becomes crucial. Techniques such as descriptive statistics (e.g., mean, median, mode) or inferential statistics (e.g., regression, ANOVA) empower individuals to systematically extract informative conclusions. Ultimately, this data-driven process leads to a deeper understanding of the issue at hand and facilitates informed decision-making. Interpreting Results and Drawing Conclusions The final step involves rigorously assessing the conclusions derived from statistical analyses. This careful evaluation demands a thorough examination of any potential limitations or biases. Additionally, acknowledging alternative interpretations strengthens the overall argument by mitigating the risk of oversimplifying complex matters. Incorporating Feedback and Adjustments A critical aspect of effectively applying statistics revolves around the willingness to reevaluate one's approach. Engaging in an iterative process and incorporating feedback helps refine the problem-solving strategy, ultimately leading to more accurate and reliable solutions. In summary, the proper use of statistics has the potential to greatly enhance individuals' ability to resolve problems in everyday life. By employing a methodical approach that involves identifying the issue, collecting relevant data, utilizing suitable techniques and critically evaluating conclusions, one can swiftly address concerns and make informed decisions.
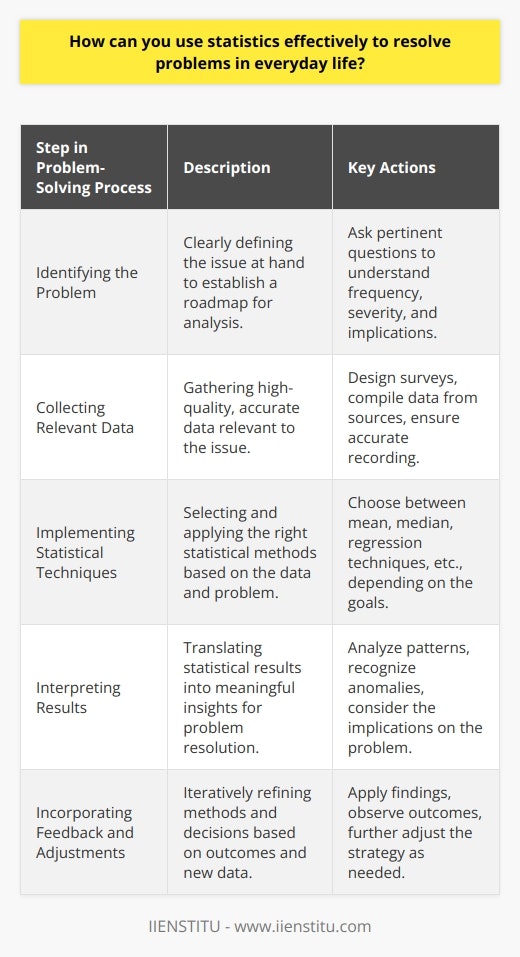
How can statistical inference be utilized to draw conclusions about a population when only a sample is available for analysis?
Statistical Inference and Population Analysis Statistical inference is an essential tool in understanding populations. It allows scientists to analyze a small, representative subset or sample of a larger population. This way, we can extract conclusions about an entire population from the analysis of a sample. Use of Sample Analysis In sample analysis, researchers collect data from a smaller subset instead of assessing the entire population. It significantly reduces the required resources and time. Nevertheless, a sample must adequately represent the characteristics of the population for valid inferences. Role of Probability Probability plays a pivotal role in statistical inference. The application of probability theories provides information about the likelihood of particular results. The conclusions drawn about the population feature a degree of certainty conveyed by probability. Statistical Tests Stepping further, statistical tests employed in the process illuminate the differences between groups within the sample. They provide guidelines for finding if observed differences occurred due to chance. By employing these tests, we can generalize findings from a sample to the entire population. Importance of Confidence Intervals Confidence intervals are another critical component of statistical inference. They present the range of values within which we expect the population value to fall a certain percent of the time, say 95%. Confidence intervals reveal more about the population parameter than a single point estimate. Conclusion and Future Predictions Between sample analysis, probability, statistical tests, and confidence intervals, statistical inference enables efficient, accurate conclusions about large population groups. Its effective use facilitates not only a comprehensive understanding of the present population status but also assists in predicting future trends. In a nutshell, statistical inference acts as a bridge connecting sample data to meaningful conclusions about the broader population. By analyzing a sample, predicting probabilities, applying statistical tests, and measuring confidence intervals, we can glean holistic insights about the entire population.
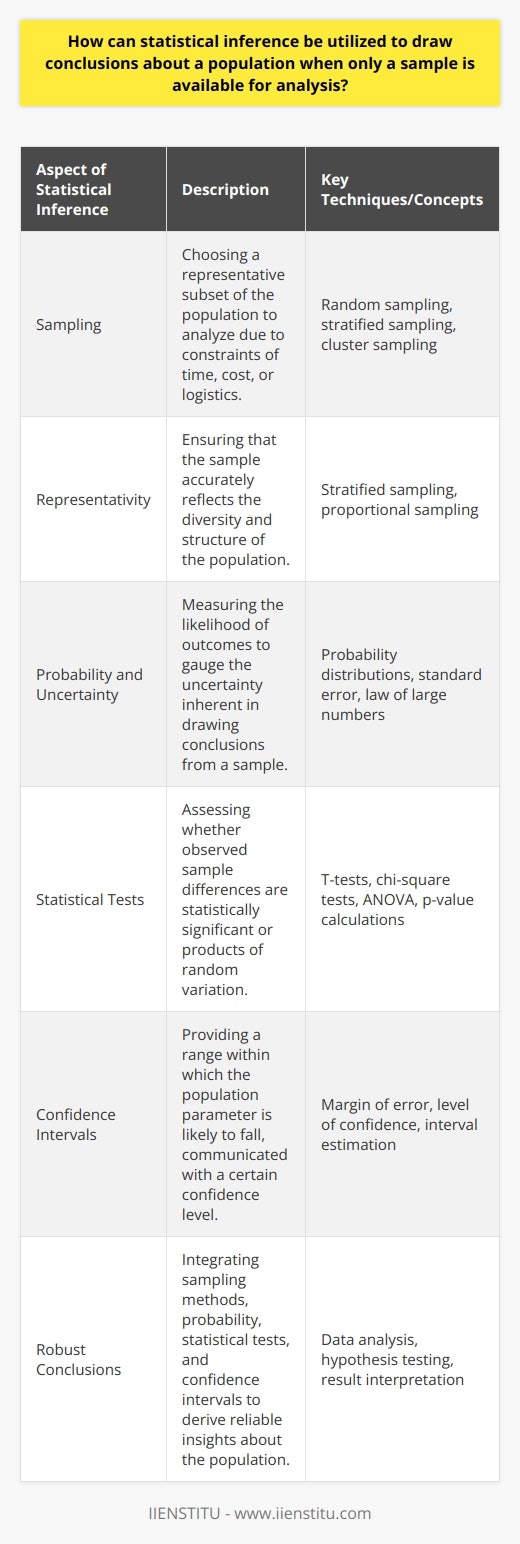
What are the key principles of robust statistical modeling, and how can these principles be applied to enhance the effectiveness of problem-solving efforts?
Understanding Robust Statistical Modeling Principles Robust statistical modeling works on three key principles. They are the use of robust measures, an effective model selection strategy, and consideration of outliers. These principles play a crucial role to ensure the robustness of statistical results. Applying Robust Measures The first principle revolves around applying robust measures. These measures are resistant to the outliers in the data set. They work by minimizing the effect of extreme values. By using these robust measures, researchers can increase the accuracy of their statistical models. Model Selection Strategy Next comes the strategy for selecting the model. It involves choosing an appropriate statistical model that aligns well with the provided data set. In this case, the most reliable models are ones that demonstrate significant results and fit the data well. Selecting an efficient model, hence, can lead to more accurate predictions or inferences. Addressing Outliers Finally, a detailed consideration of outliers is vital. Outliers can skew the results of a model significantly. They need careful handling to prevent any bias in the final results. Recognizing and appropriately managing these outliers aids in maintaining the integrity of statistical findings. Enhancing Problem-Solving Efforts These principles, when applied effectively, can significantly enhance problem-solving efforts. By using robust measures, researchers can achieve more accurate results, increasing the credibility of their findings. A well-chosen model can enhance the interpretability and usefulness of the results. Furthermore, careful handling of outliers can prevent skewed results, ensuring more reliable conclusions. In essence, by embracing these principles, one can substantially elevate their problem-solving capabilities, making the process more efficient and effective. Thus, robust statistical modeling acts as a powerful tool in addressing various research questions and solving complex problems.
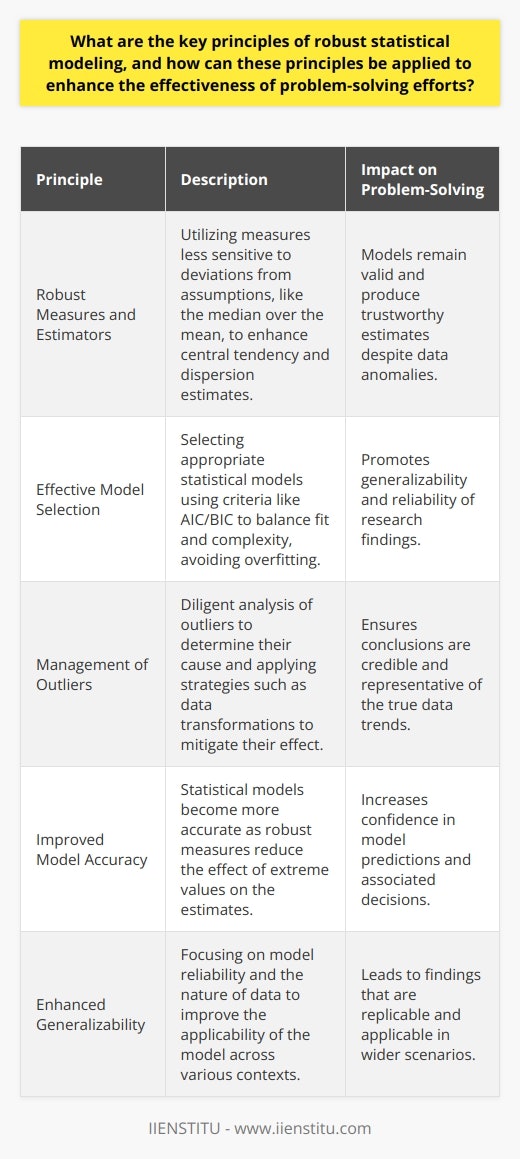
How can the utilizations of time series analysis in statistics support trend identification and forecasting in the context of complex/problem-solving situations?
Identifying Trends with Time Series Analysis: A crucial aspect of time series analysis in statistics is trend identification. Time series analysis allows statisticians to discern patterns in data collected over time. These trends indicate changes in variables, creating a historical line that tracks these alterations across a span of time. Support for Complex Problem Solving: In complex problem-solving situations, time series analysis can provide valuable support. Specifically, it can facilitate independent, variable-dependent trend analysis and insights into relationships within data sequences. This is vital for complex situations requiring deeper analysis. Time Series Analysis for Forecasting: Another primary use of time series analysis is for forecast predictions in future scenarios. By analyzing the trends identified, predictions can suggest plausible future scenarios. This forecasting capability can be critical in planning and preparation for potential future events based on the observed trends. Predictive Modeling: Predictive modeling can be improved with time series analysis. It helps understand population trends or related metrics. By revealing underlying patterns, time series analysis supports data-driven decision making in complex situations. In summary, time series analysis plays an instrumental role in statistics. Through trend identification and forecasting, it provides invaluable support for complex problem-solving situations. This statistical tool is essential for those working in an environment that requires a clear, predictive understanding of data over time.
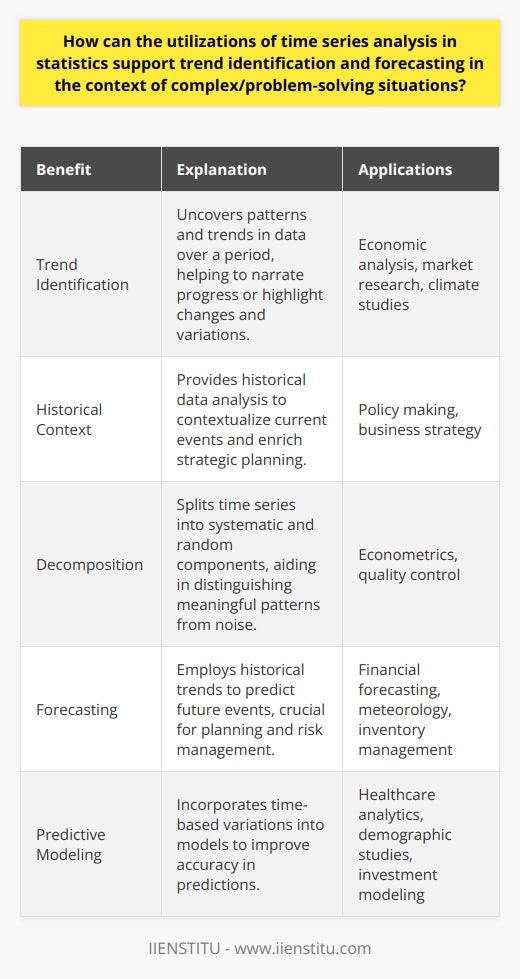
How can statistics help with problem solving?
Effective Use of Statistics Statistics offers efficient problem-solving tools. They provide the ability to measure, forecast, and make informed decisions. When faced with a problem, statistics help in gathering relevant data. Understanding the Problem Statistics helps to describe the problem objectively. Before proceeding with problem solving, a clear definition of the problem is necessary. Statistics describe problems quantitatively, bringing precision in problem definition. Identifying Solutions Statistics aids in identifying potential solutions. By using predictive analytics, statistics can forecast the outcomes of various solutions. Thus, it assists in the selection of most efficient solution based on the forecasted results. Evaluating Results Once a solution is implemented, statistics help in evaluation. They measure the effectiveness of the solution by comparing the outcomes with the predicted results. Promoting Continuous Improvement Statistics guide continuous improvement. They pinpoint deviations, enabling identification of areas of improvement. This leads to enhanced effectiveness in problem solving. Statistics has a pivotal role in problem solving. The data-driven approach enhances the credibility of the problem-solving process and the ultimate solutions. The various statistical tools improve both the efficiency and effectiveness, leading to better solutions.
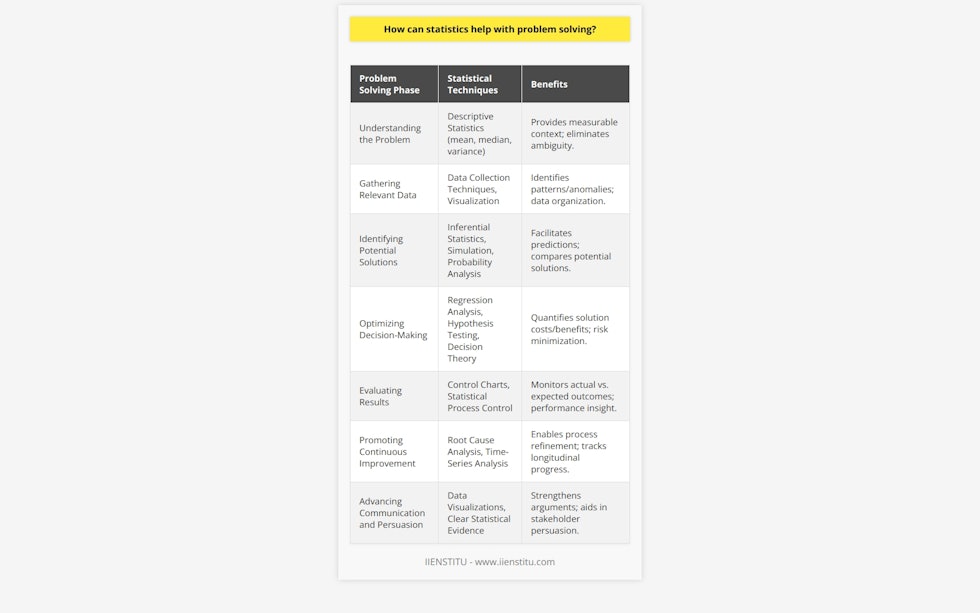
Why is data analysis important in problem solving?
Data Analysis and Problem-Solving: A Crucial Connection Data analysis stands as a critical tool in problem solving in the contemporary business environment. Essentially, it offers insightful measurements of challenges. By examining data, we uncover patterns and trends to identify problems. Identification of Issues The initial step in problem-solving involves the recognition of a problem. It is here that data analysis proves vital. It grants a robust basis for this recognition, presenting objective rather than subjective identifiers. Understanding the Nature of Problems Once we identify a problem, we must understand its nature. In-depth data analysis can provide a detailed insight into why problems arise. It examines multiple variable relationships, often revealing root causes. Generating Solutions Data analysis aids in creating suitable solutions. By understanding the problem from a data perspective, we can draw up potential fixes. These solutions are often grounded on empirical evidence, hence sound and reliable. Evaluating Outcomes After solution implementation, evaluation follows closely. Analyzing data post-implementation helps measure the effectiveness of the solution. It provides a measure on the success of the problem-solving process. In conclusion, data analysis is a strong ally in problem-solving. It facilitates issue identification, enhances understanding, helps to generate solutions, and evaluates outcomes. By utilizing this tool, we can significantly improve our problem-solving efforts, leading to more effective and measurable results.
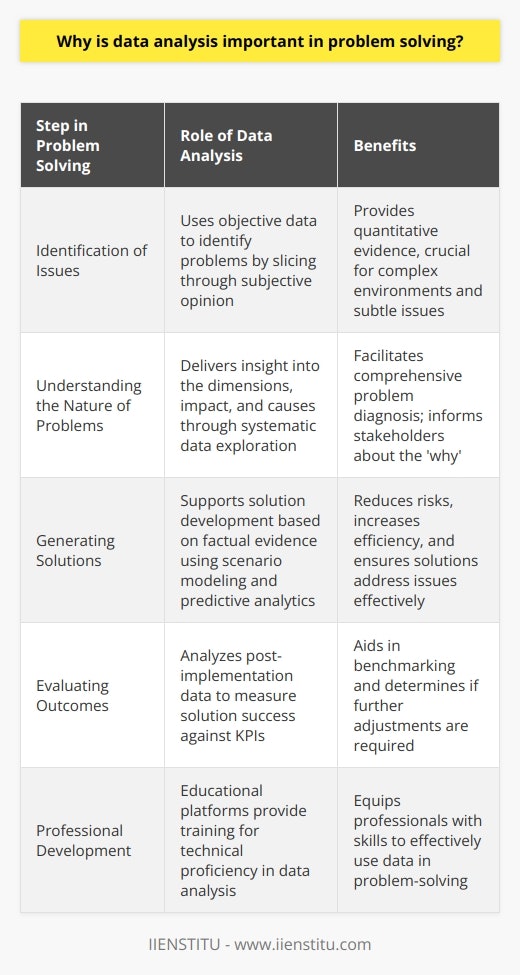
How does statistics make you a better thinker?
Enhancing Reasoning and Decision Making Skills Statistics equips one with necessary tools to question and interpret data intelligently. It sharpens critical reasoning abilities by offering ways to identify patterns or anomalies, thus improving decision-making efficiency. Understanding Probabilities and Predictions Statistics introduces individuals to the concept of probability, enabling them to weigh the likelihood of different scenarios accurately. Consequently, it allows them to make precise and informed predictions, honing their thinking and analytical skills. Building Quantitative Literacy Statistics promotes quantitative literacy, a vital skill in a data-driven world. Understanding numerical information helps individuals decipher complex data and convert it into actionable insights. This heightens critical thinking abilities and enables better understanding of the world. Critiquing Data Effectively Statistics improves a person's ability to critically analyze presented data. Using statistical tools, one can identify manipulation or misinterpretation in data, preventing them from taking misleading information at face value. Developing Logical Reasoning Statistics fosters effective problem-solving skills by inciting logical reasoning. It drives individuals to meticulously analyze data, look for patterns and draw logical conclusions, thus streamlining strategic decision-making processes. In conclusion, mastering the use of statistics can effectively enhance a person's thinking capacity. It works on multiple fronts ranging from decision-making to quantitative literacy to critiquing data, making one a more discerning and astute individual. Statistics, therefore, plays a pivotal role in developing vital cognitive abilities.
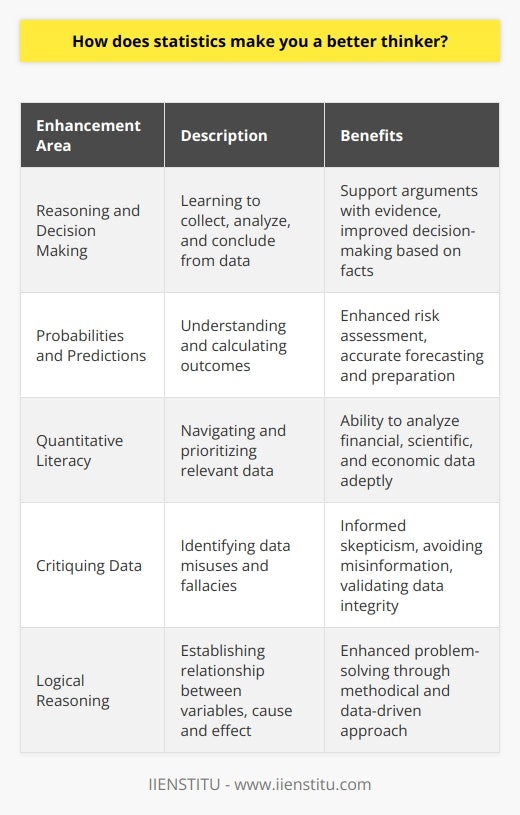
Yu Payne is an American professional who believes in personal growth. After studying The Art & Science of Transformational from Erickson College, she continuously seeks out new trainings to improve herself. She has been producing content for the IIENSTITU Blog since 2021. Her work has been featured on various platforms, including but not limited to: ThriveGlobal, TinyBuddha, and Addicted2Success. Yu aspires to help others reach their full potential and live their best lives.
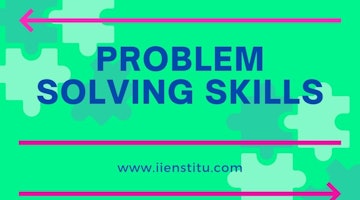
What are Problem Solving Skills?
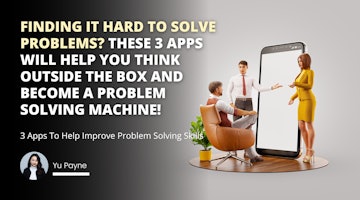
3 Apps To Help Improve Problem Solving Skills
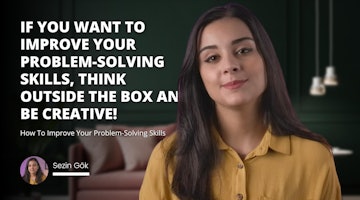
How To Improve Your Problem-Solving Skills
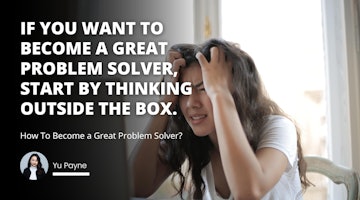
How To Become a Great Problem Solver?

Free math problem solver answers your statistics homework questions with step-by-step explanations. Mathway. Visit Mathway on the web. Start 7-day free trial on the app. Start 7-day free trial on the app. Download free on Amazon. Download free in Windows Store. get Go. Statistics. Basic Math. Pre-Algebra. Algebra. Trigonometry. Precalculus.
Welcome! Here, you will find all the help you need to be successful in your statistics class. Check out our statistics calculators to get step-by-step solutions to almost any statistics problem. Choose from topics such as numerical summary, confidence interval, hypothesis testing, simple regression and more.
Learn how to conquer statistical problems by leveraging tools such as statistical software, graphing calculators, and online resources. Discover the key steps to effectively solve statistical challenges: define the problem, gather data, select the appropriate model, use tools like R or Python, and validate results. Dive into the world of DataCamp for interactive statistical learning experiences.
This website provides training and tools to help you solve statistics problems quickly, easily, and accurately - without having to ask anyone for help. ... Test your understanding of key topics, through sample problems with detailed solutions. Be prepared. Get the score that you want on the AP Statistics test.
Descriptive statistics is a branch of statistics that deals with summarizing, organizing and describing data. Descriptive statistics uses measures such as central tendency (mean, median, and mode) and measures of variability (range, standard deviation, variance) to give an overview of the data.
It involves a team armed with process and product knowledge, having willingness to work together as a team, can undertake selection of some statistical methods, have willingness to adhere to principles of economy and willingness to learn along the way. Statistical Problem Solving (SPS) could be used for process control or product control.
There are 10 modules in this course. Statistical Thinking for Industrial Problem Solving is an applied statistics course for scientists and engineers offered by JMP, a division of SAS. By completing this course, students will understand the importance of statistical thinking, and will be able to use data and basic statistical methods to solve ...
Statistical Thinking and Problem Solving. Statistical thinking is about understanding, controlling and reducing process variation. Learn about process maps, problem-solving tools for defining and scoping your project, and understanding the data you need to solve your problem.
Consider statistics as a problem-solving process and examine its four components: asking questions, collecting appropriate data, analyzing the data, and interpreting the results. This session investigates the nature of data and its potential sources of variation. Variables, bias, and random sampling are introduced. View Transcript.
Table of contents. Step 1: Write your hypotheses and plan your research design. Step 2: Collect data from a sample. Step 3: Summarize your data with descriptive statistics. Step 4: Test hypotheses or make estimates with inferential statistics.
A statistics problem typically contains four components: 1. Ask a Question. Asking a question gets the process started. It's important to ask a question carefully, with an understanding of the data you will use to find your answer. 2, Collect Data. Collecting data to help answer the question is an important step in the process.
Problem 1. In one state, 52% of the voters are Republicans, and 48% are Democrats. In a second state, 47% of the voters are Republicans, and 53% are Democrats. Suppose a simple random sample of 100 voters are surveyed from each state. What is the probability that the survey will show a greater percentage of Republican voters in the second state ...
Statistical thinking is vital for solving real-world problems. At the heart of statistical thinking is making decisions based on data. This requires disciplined approaches to identifying problems and the ability to quantify and interpret the variation that you observe in your data. In this module, you will learn how to clearly define your ...
1 Define the problem. The first step is to clearly define the problem you want to solve and the objectives you want to achieve. You should also identify the variables that are relevant to the ...
The Six Sigma approach is a truly powerful problem-solving tool. By working from a practical problem to a statistical problem, a statistical solution and finally a practical solution, you will be assured that you have identified the correct root cause of the problem which affects the quality of your products.
The Shainin System™ (SS) is defined as a problem-solving system designed for medium- to high-volume processes where data are cheaply available, statistical methods are widely used, and intervention into the process is difficult. ... Converge executes the strategies by applying statistical tools in a series of tactical cycles where each cycle ...
Get full access to all Solution Steps for any math problem By continuing, you agree to our ... Word Problems. Functions & Graphing. Geometry. Trigonometry. Pre Calculus. Calculus. Statistics. Calculations. Popular Statistics Problems. maximum of 1,-4,5 maximum\:1,-4,5: 19 choose 8 19C8: median of 48,52,54,51,55 ... Study Tools AI Math Solver ...
Seven Statistical Tools Overview. Course Duration: 1 Day - 8 Hours/day. This one-day seminar provides training on the seven statistical tools. These tools are used in problem solving and continual improvement endeavors. The seven statistical tools were first introduced by Dr. Ishikawa when he introduced problem solving in Japan.
Minitab provides a multitude of solutions for engineers to aid in problem solving and analytics. Attack problems with brainstorming tools, plan projects and process improvements through visual tools, and then collect data and analyze it, all within the Minitab ecosystem. Our solutions can help you find the answers you need using graphical tools ...
Statistical analytics could be an excellent career match for those with an affinity for math, data, and problem-solving. Here are some popular courses to consider as you prepare for a career in statistical analysis: Learn fundamental processes and tools with Google's Data Analytics Professional Certificate. You'll learn how to process and ...
Six Sigma tools are defined as the problem-solving tools used to support Six Sigma and other process improvement efforts. The Six Sigma expert uses qualitative and quantitative techniques to drive process improvement. ... Some of the statistical and graphical tools commonly used in improvement projects are: DMAIC: The define, measure, analyze ...
2.1 Automation of Computational Procedures. Automation of calculations and graphing has long been touted as a primary benefit for how technology tools can assist students and teachers in focusing on higher level concepts and problem solving in statistics (e.g., Ben-Zvi Citation 2000; Chance et al. Citation 2007).One reason researchers support the use of technology in education is that, when ...
Problem-solving is an essential skill that everyone must possess, and statistics is a powerful tool that can be used to help solve problems. Statistics uses probability theory as its base and has a rich assortment of submethods, such as probability theory, correlation analysis, estimation theory, sampling theory, hypothesis testing, least squares fitting, chi-square testing, and specific ...

IMAGES
VIDEO
COMMENTS
Following her extensive refit at Drettmann Yachts in Germany's Bremen, motor yacht Bandido 75 has found her new Owner. Previously owned by ex Formula 1 star Ralf Schumacher, luxury yacht Bandido 75 is a lovely explorer, constructed in 2008.
Lloyds Register classification. Sleeps 8 overnight. 8.14m/26'8" HIghfield Tender. The 26.21m/86' motor yacht 'Bandido' was built by Jade Yachts in Taiwan. Her interior is styled by design house Jade Yachts and she was completed in 2007. This luxury vessel's exterior design is the work of Espinosa Yacht Design and she was last refitted in 2022.
Drettmann Yachts' most recent sale is a Bandido 90, for which they were able to inspire a German owner. "The customer was immediately impressed by the outstanding build quality," says Albert Drettmann, under whose direction the Explorer was once built, when Drettmann Yachts had been highly successful in placing the Bandido series on the market.
About Bandido Yachts . On the water the freedom of this world still seems boundless. Endless horizons, sun, wind and still unknown areas let you forget your everyday life and tickle the spirit of adventure in every owner and guest. The Bandido Yachts brand was developed precisely for this experience: On the outside as an uncompromising explorer ...
8. BANDIDO is a 27.9 m Motor Yacht, built in Taiwan by Jade Yachts and delivered in 2008. She is one of 8 Bandido 90 Explorer models. Her top speed is 12.9 kn and she boasts a maximum range of 3500.0 nm when navigating at cruising speed, with power coming from two Caterpillar diesel engines. She can accommodate up to 8 guests in 4 staterooms ...
She is the first yacht from the Bandido series and therefore continues the legacy of this design. This remarkable vessel, built in 2007, is a luxurious classic and stands for excellent german construction quality and robustness. ... A stunning owner's suite spanning the entire width of the main deck. Impeccable craftsmanship and attention to ...
A Summary of Motor Yacht BANDIDO. Coming from the Drettmann shipyard in Germany the BANDIDO is 27 m 90 (foot) in length. Completed by 2007 the comparatively recent interior design illustrates the progressive thinking of owner and Drettmann. Superyacht BANDIDO is able to accommodate up to 12 people with 3 qualified crew.
Bandido is a 63ft, triple award-winning, Oyster 625 - the signature creation of the world-renowned yacht designer, Rob Humphreys who works with the Oyster Design Team in coordination with owners to develop beautiful semi-custom vessels. One of the advantages from the onset of her design was that Bandido is designed to be an owner-driven boat, as she is currently owned and operated solely by ...
Bandido boats for sale on YachtWorld are listed for a range of prices from $1,596,757 on the relatively more affordable end, with costs up to $18,050,299 for the most advanced and biggest yachts. What Bandido model is the best? Some of the most iconic Bandido models currently listed include: 75, 90, 170, 90' Explorer and Drettman Horizon 79.
In the world rankings for largest yachts, the superyacht, Bandido I, is listed at number 7927. She is the 8th-largest yacht built by Jade Yachts. Bandido I's owner is shown in SYT iQ and is exclusively available to subscribers. On SuperYacht Times, we have 60 photos of the yacht, Bandido I, and she is featured in 2 yacht news articles.
The 23.67m/77'8" motor yacht 'Bandido 75' was built by Horizon in Taiwan. This luxury vessel's exterior design is the work of Horizon. Guest Accommodation. ... Bandido 75 Yacht Owner, Captain or marketing company 'Yacht Charter Fleet' is a free information service, if your yacht is available for charter please contact us with details and photos ...
In fact, Felci Yacht Design is able to offer complete customization of the boat down to the smallest details. The Ice 70 Bandido welcomes those who enter the boat with very light colours, shades of whites that make the environment extremely bright and elegant. Protagonists, in the centre of saloon, are two remarkable armchairs signed Natuzzi in ...
About Bandido 80 . Drettmann Yachts have firmly established their presence across the world's oceans for a considerable duration, progressively increasing in numbers. ... earning the admiration of numerous owners. The most recent models are completely new, having been designed and developed from the ground up. Comfort, space and motorization ...
Italian shipyard Ice Yachts has launched the luxury sailing yacht BANDIDO at a private ceremony attended by the Owner in June 2022. ... The ICE 70 model sailing yacht BANDIDO was constructed with a fibreglass sandwich and carbon hull and superstructure to a design by Felci Yacht Design. A plumb bow and sturdy proportions combine traditional and ...
News, yachts for sale & yachts for charter, cruising destinations and yachting intelligence ... is a luxury yacht builder based in Germany providing naval architecture & new building services to the most discerning owners. ... Bandido Services. Naval architecture shipyard. Shipyards: New Building. Bandido Contact Details. Arberger Hafendamm 22 ...
El Bandido is a custom motor yacht launched in 2008 by Custom, in Turkey. Design. El Bandido measures 31.70 metres in length and has a beam of 6.50 feet. El Bandido has a steel hull. Performance and Capabilities. El Bandido has a top speed of 14.00 knots and a cruising speed of 12.00 knots. . Accommodation. El Bandido accommodates up to 8 ...
In 1938, it was granted town status. [citation needed]Administrative and municipal status. Within the framework of administrative divisions, it is incorporated as Elektrostal City Under Oblast Jurisdiction—an administrative unit with the status equal to that of the districts. As a municipal division, Elektrostal City Under Oblast Jurisdiction is incorporated as Elektrostal Urban Okrug.
Bandido Yachts The sea has always held fascination for people: infinite space, sun, wind and freedom. Technically superb, suitable for voyages of discovery on the high seas yet luxurious and sporty - the Explorer yachts in the Bandido series ensure a unique yachting experience.Measuring from 80 to 148 feet, the Bandidos boast an innovative pod drive, an impressive beach club and lots of space.
March 17, 2011. Pavel Oderov was appointed as Head of the International Business Department pursuant to a Gazprom order. Pavel Oderov was born in June 1979 in the town of Elektrostal, Moscow Oblast. He graduated from Gubkin Russian State University of Oil and Gas with an Economics degree in 2000 and a Management degree in 2002.
Luxury sailing yacht BANDIDO from Italian shipyard Ice Yachts is a 21.3m/70ft ICE 70 model with exterior styling from Felci Yacht Design. The hull and superstructure are constructed from carbon with a fibreglass sandwich to create a lightweight draft and excellent efficiency while cruising. Depending on the ICE 70 layout the Owner selected, S/Y ...
Apr 24, 2018. Hanse's E-motion electric rudder drive represents a true breakthrough in auxiliary propulsion for saiboats. When news that Hanse Yachts had launched a new form of electric-powered yacht first broke in the winter of 2016, it was widely reported. After all, Hanse is one of the world's biggest builders of sailing boats, so this .....
Fusing activities with luxury, this robust yacht boasts generous onboard space for tenders, surfboards, diving gear, and water toys, without compromising on comfort. The latest models ranging from 80 to 148 feet represent an exponential leap forward in refinement compared to their esteemed predecessors. Features, such as the revamped interior ...
Impressions; At 91 meters in length, Lady Lara is an ultramodern superyacht with sweeping curves and an elegantly balanced profile. Dynamic, sculpted features carry through her ex